A Multi-Modal ELMo Model for Image Sentiment Recognition of Consumer Data
IEEE Transactions on Consumer Electronics(2024)
摘要
Recent advancements in consumer electronics as well as imaging technology have generated abundant multimodal data for consumer-centric AI applications. Effective analysis and utilization of such heterogeneous data hold great potential for consumption decisions. Hence, effective analysis of multi-modal consumer-generated content is a prominent research topic in the field of customer-centric artificial intelligence (AI). However, two key challenges that arise in this task are multi-modal representation and fusion. To address these issues, we propose a multimodal embedding from the language model (MELMo) enhanced decision-making model. The main idea is to extend the ELMo to a multi-modal scenario by designing a deep contextualized visual embedding from the language model (VELMo) and modeling multi-modal fusion at the decision level by using the cross-modal attention mechanism. In addition, we also designed a novel multitask decoder to learn the shared knowledge from related tasks. We evaluate our approach on two benchmark datasets, CMUMOSI and CMU-MOSEI, and show that MELMo outperforms state-of-the-art approaches. The F1 scores on the CMU-MOSI and CMU-MOSEI datasets reach 86.1% and 85.2%, respectively, representing an improvement of approximately 1.0% and 1.3% over the state-of-the-art system, providing an effective technique for multimodal consumer analytics in electronics and beyond.
更多查看译文
关键词
Consumer-centric AI,Image sentiment analysis,multi-modal fusion,deep learning
AI 理解论文
溯源树
样例
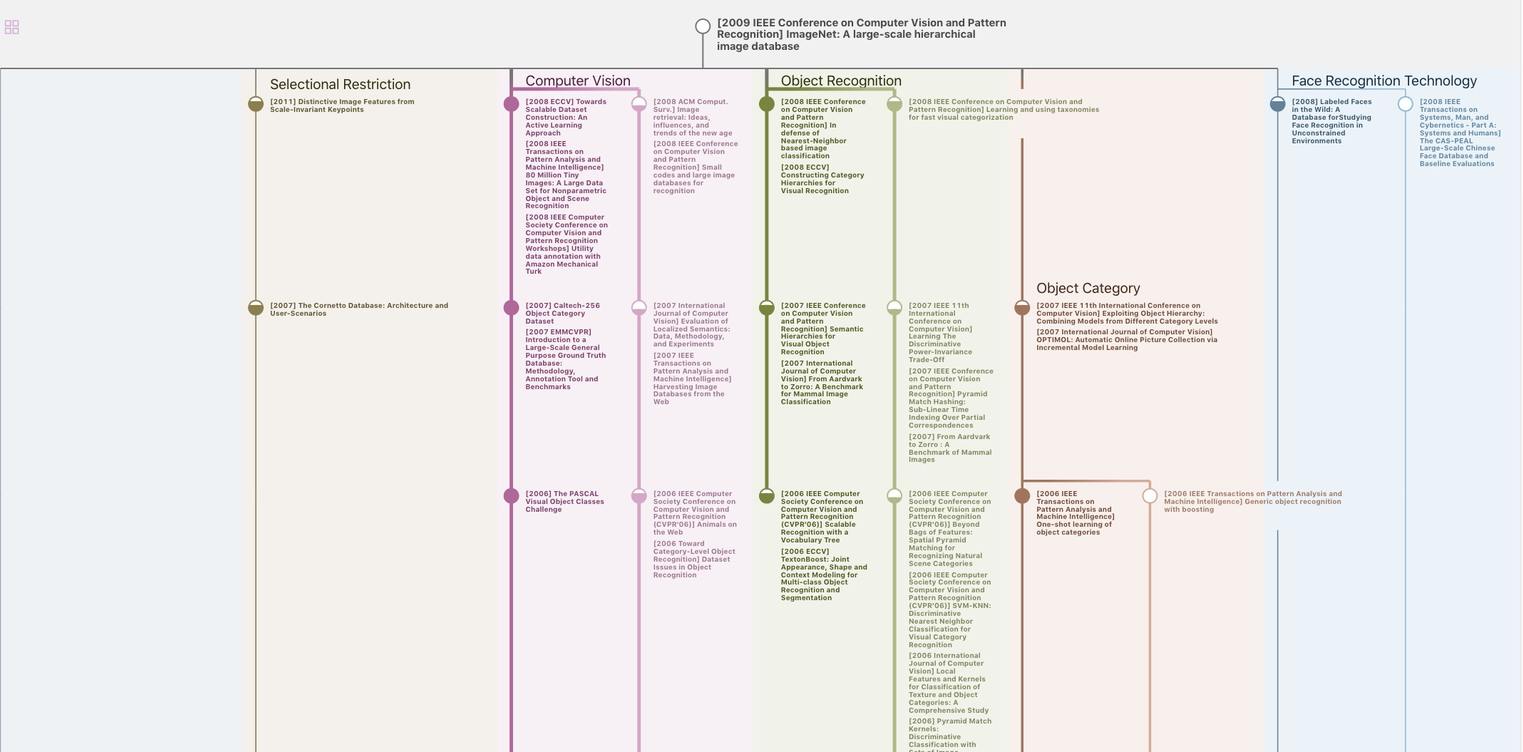
生成溯源树,研究论文发展脉络
Chat Paper
正在生成论文摘要