MIGAN: A Privacy Leakage Evaluation Scheme for CIoT-Based Federated Learning Users
IEEE TRANSACTIONS ON CONSUMER ELECTRONICS(2024)
摘要
Federated Learning (FL) in Consumer Internet of Things (CIoT) encounters significant privacy security threats when collaborative training Machine Learning models using data distributed among numerous smart CIoT devices. This paper's objective is to evaluate the potential privacy risks associated with FL within CIoT context, specifically through the lens of passive malicious users. We propose an evaluation scheme (MIGAN) for privacy leakage, which incorporates various privacy inference attacks. First, this paper analyzes the characteristics of privacy inference attacks in FL and summarizes privacy security threats to guide the design of the MIGAN evaluation scheme. Then, to fully utilize the privacy information in FL, this paper proposes faster model inversion and white-box membership inference. Integrating these two modules into the MIGAN framework facilitates fast and high-quality privacy data reconstruction. Finally, this paper constructs the evaluation function to measure the correlation between compromised privacy data and users' training data. Experiments conducted on the MNIST and CelebA datasets reveal that MIGAN's data privacy reconstruction outperforms comparable methods. Notably, MIGAN quantifies the likelihood of potential privacy leakage at 14.7% for MNIST and 8.3% for CelebA in FL with 30 clients. These results validates the practicality and relevance of MIGAN in evaluating privacy leakage risks.
更多查看译文
关键词
Federated learning,privacy attack,model inversion,membership inference
AI 理解论文
溯源树
样例
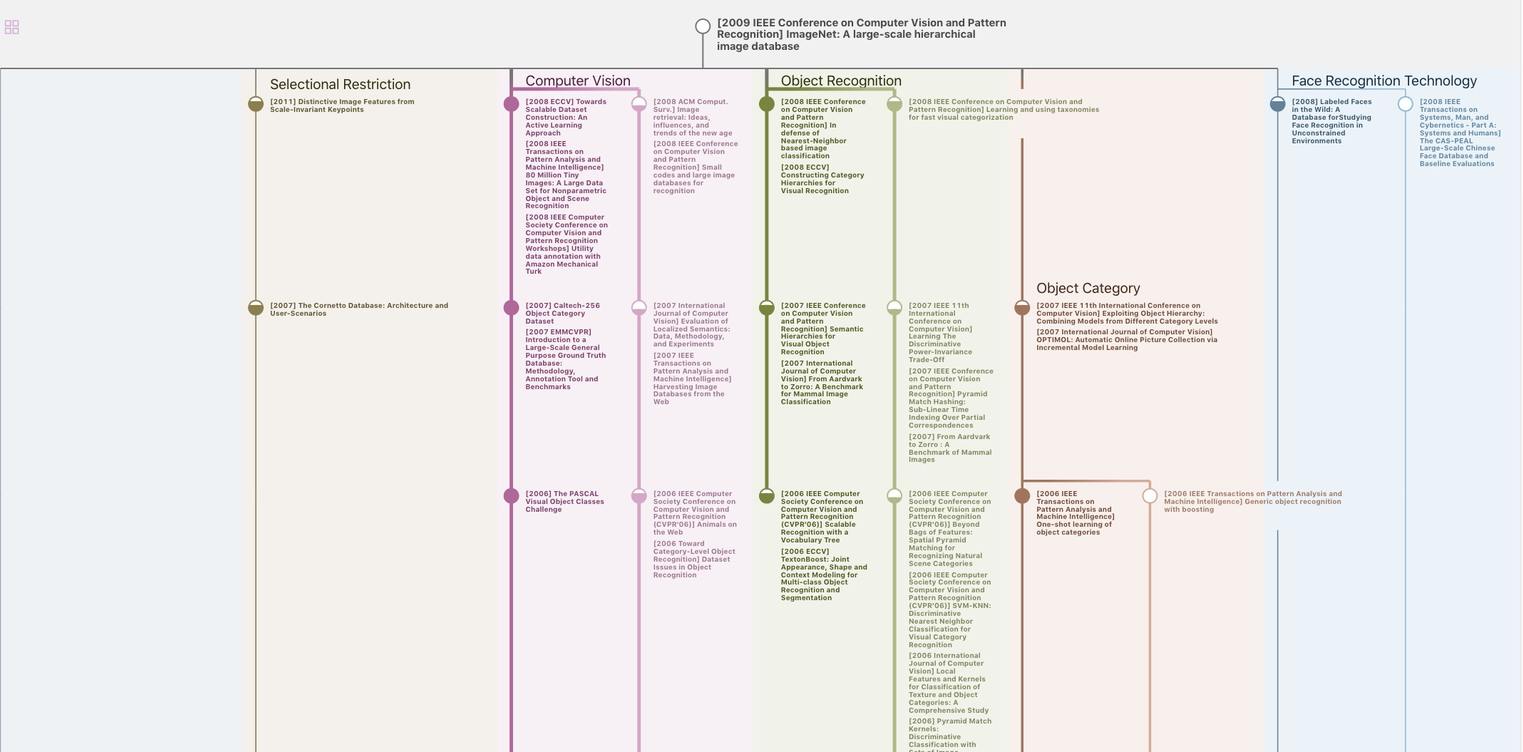
生成溯源树,研究论文发展脉络
Chat Paper
正在生成论文摘要