A Degree-Dependent Polynomial-Based Reciprocally Convex Matrix Inequality and Its Application to Stability Analysis of Delayed Neural Networks
IEEE TRANSACTIONS ON CYBERNETICS(2024)
摘要
In this article, several improved stability criteria for time-varying delayed neural networks (DNNs) are proposed. A degree-dependent polynomial-based reciprocally convex matrix inequality (RCMI) is proposed for obtaining less conservative stability criteria. Unlike previous RCMIs, the matrix inequality in this article produces a polynomial of any degree in the time-varying delay, which helps to reduce conservatism. In addition, to reduce the computational complexity caused by dealing with the negative definite of the high-degree terms, an improved lemma is presented. Applying the above matrix inequalities and improved negative definiteness condition helps to generate a more relaxed stability criterion for analyzing time-varying DNNs. Two examples are provided to illustrate this statement.
更多查看译文
关键词
High-order polynomials,neural networks,reciprocally convex matrix inequality (RCMI),stability,time-varying delay
AI 理解论文
溯源树
样例
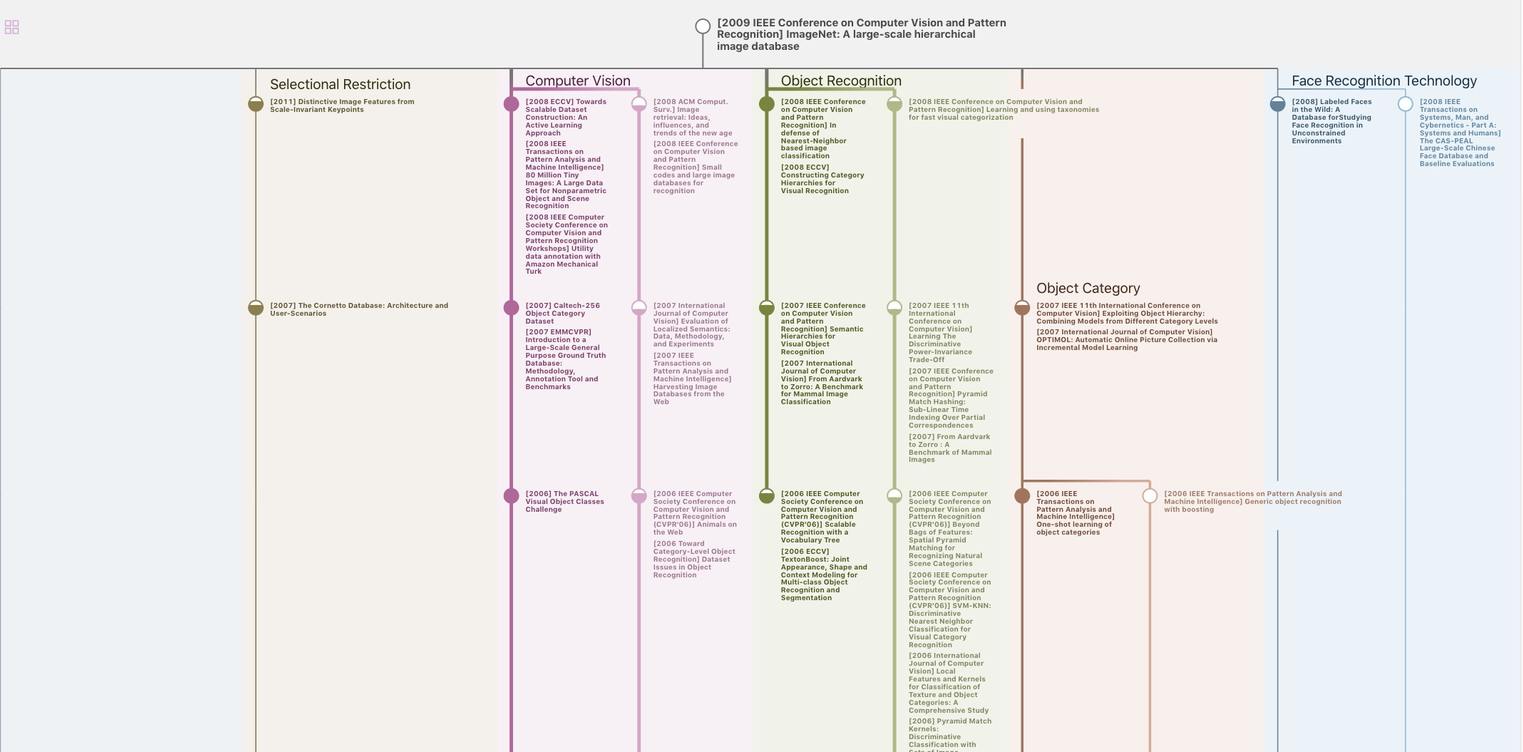
生成溯源树,研究论文发展脉络
Chat Paper
正在生成论文摘要