Adjustable Multi-Stream Block-Wise Farthest Point Sampling Acceleration in Point Cloud Analysis.
IEEE Trans. Circuits Syst. II Express Briefs(2024)
Abstract
Point cloud is increasingly used in a variety of applications. Farthest Point Sampling (FPS) is typically employed for down-sampling to reduce the size of point cloud and enhance the representational capability by preserving contour points in point cloud analysis. However, due to low parallelism and high computational complexity, high energy consumption and long latency are caused, which becomes a bottleneck of hardware acceleration. In this brief, we propose an adjustable multi-stream block-wise FPS algorithm, adjusted by four configurable parameters, according to hardware and accuracy requirements. A unified hardware architecture with one parameter is designed to implement the adjustable multi-stream block-wise FPS algorithm. Furthermore, we present a rapid searching algorithm to select the optimal configuration of the five parameters. Designed in an industrial 28-nm CMOS technology, the proposed hardware architecture achieves a latency of 0.005 (1.401) ms and a frame energy consumption of 0.09 (27.265) μJ/frame for 1 k (24 k) input points at 200 MHz and 0.9 V supply voltage. Compared to the state of the art, the proposed hardware architecture reduces the latency by up to 99.9%, saves the energy consumption by up to 99.5%, and improves the network accuracy by up to 9.34%.
MoreTranslated text
Key words
Point cloud neural networks,mapping,parallelism,FPS,acceleration framework,energy consumption,network accuracy,hardware architecture
AI Read Science
Must-Reading Tree
Example
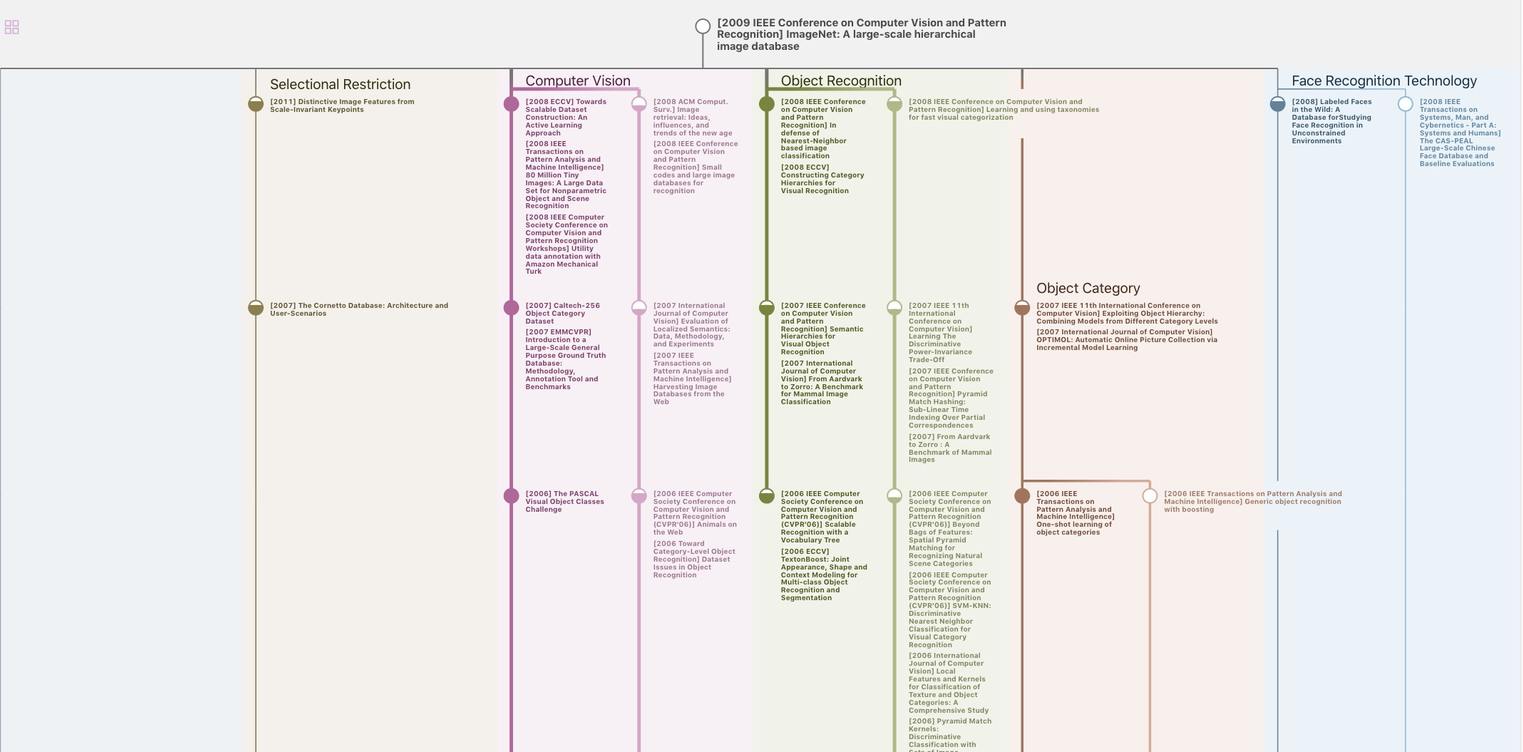
Generate MRT to find the research sequence of this paper
Chat Paper
Summary is being generated by the instructions you defined