DQN Approach for Adaptive Self-Healing of VNFs in Cloud-Native Network
IEEE ACCESS(2024)
摘要
The transformation from physical network function to Virtual Network Function (VNF) requires a fundamental design change in how applications and services are tested and assured in a hybrid virtual network. Once the VNFs are onboarded in a cloud network infrastructure, operators need to test VNFs in real-time at the time of instantiation automatically. This paper explicitly analyses the problem of adaptive self-healing of a Virtual Machine (VM) allocated by the VNF with the Deep Reinforcement Learning (DRL) approach. The DRL-based big data collection and analytics engine performs aggregation to probe and analyze data for troubleshooting and performance management. This engine helps to determine corrective actions (self-healing), such as scaling or migrating VNFs. Hence, we proposed a Deep Queue Learning (DQL) based Deep Queue Networks (DQN) mechanism for self-healing VNFs in the virtualized infrastructure manager. Virtual network probes of closed-loop orchestration perform the automation of the VNF and provide analytics for real-time, policy-driven orchestration in an open networking automation platform through the stochastic gradient descent method for VNF service assurance and network reliability. The proposed DQN/DDQN mechanism optimizes the price and lowers the cost by 18% for resource usage without disrupting the Quality of Service (QoS) provided by the VNF. The outcome of adaptive self-healing of the VNFs enhances the computational performance by 27% compared to other state-of-the-art algorithms.
更多查看译文
关键词
Self-healing VNF,deep queue networks,operational automation,cloud-native deployment,ONAP,network intelligence
AI 理解论文
溯源树
样例
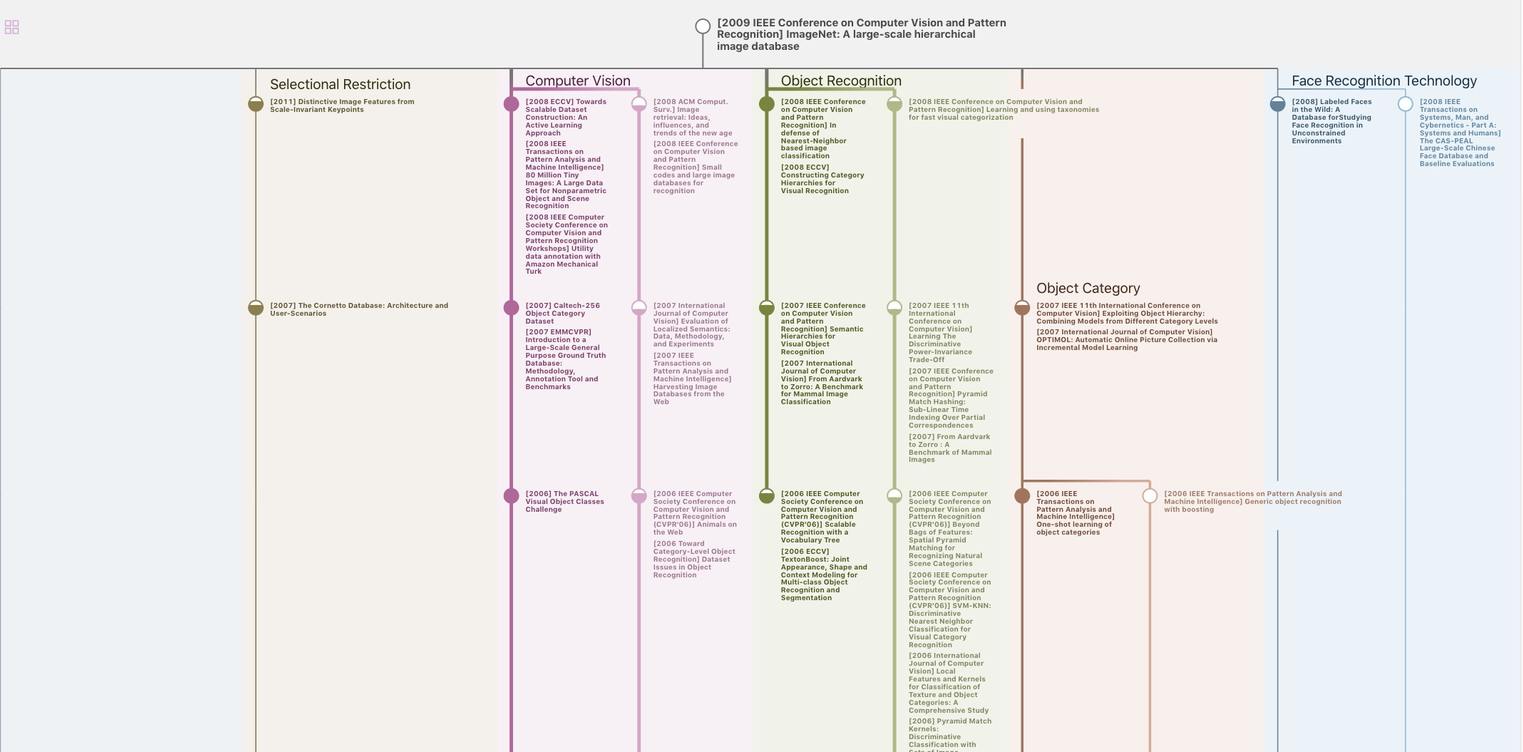
生成溯源树,研究论文发展脉络
Chat Paper
正在生成论文摘要