NO-QSM: combining convolutional neural network with numerical optimization algorithm for Quantitative Susceptibility Mapping reconstruction
IEEE Access(2024)
摘要
In gradient echo MRI, quantitative susceptibility mapping (QSM) quantifies the magnetic susceptibility distributions of tissues, which has great potential in detecting brain diseases. However, QSM reconstruction is an ill-conditional inversion problem because of the zeros in the frequency domain of the dipole kernel. The intrinsic nature of the ill-posedness would affect the accuracy of quantifying tissue susceptibility. Recently, deep learning-based methods have been proposed to improve accuracy by suppressing the streaking artifacts. In this work, we proposed a hybrid architecture to enforce data consistency by involving numerical optimization blocks within convolutional neural networks (CNN), which aimed to reconstruct high-quality QSM images, referred to as NO-QSM. The Calculation of Susceptibility through Multiple Orientation Sampling (COSMOS) QSM maps were used as labels for training. The performance of the proposed method was evaluated on two healthy volunteers and brain images of patients with diseases. Our experiments showed that the proposed method achieved good performance in terms of quantitative metrics and could effectively suppress artifacts in reconstructed QSM images, demonstrating its potential for future applications. For experiments on patients with multiple sclerosis (MS), the proposed method could better detect lesion regions in the results of NO-QSM.
更多查看译文
关键词
Quantitative susceptibility mapping,dipole kernel inversion,MRI,numerical optimization,convolutional neural netwrok
AI 理解论文
溯源树
样例
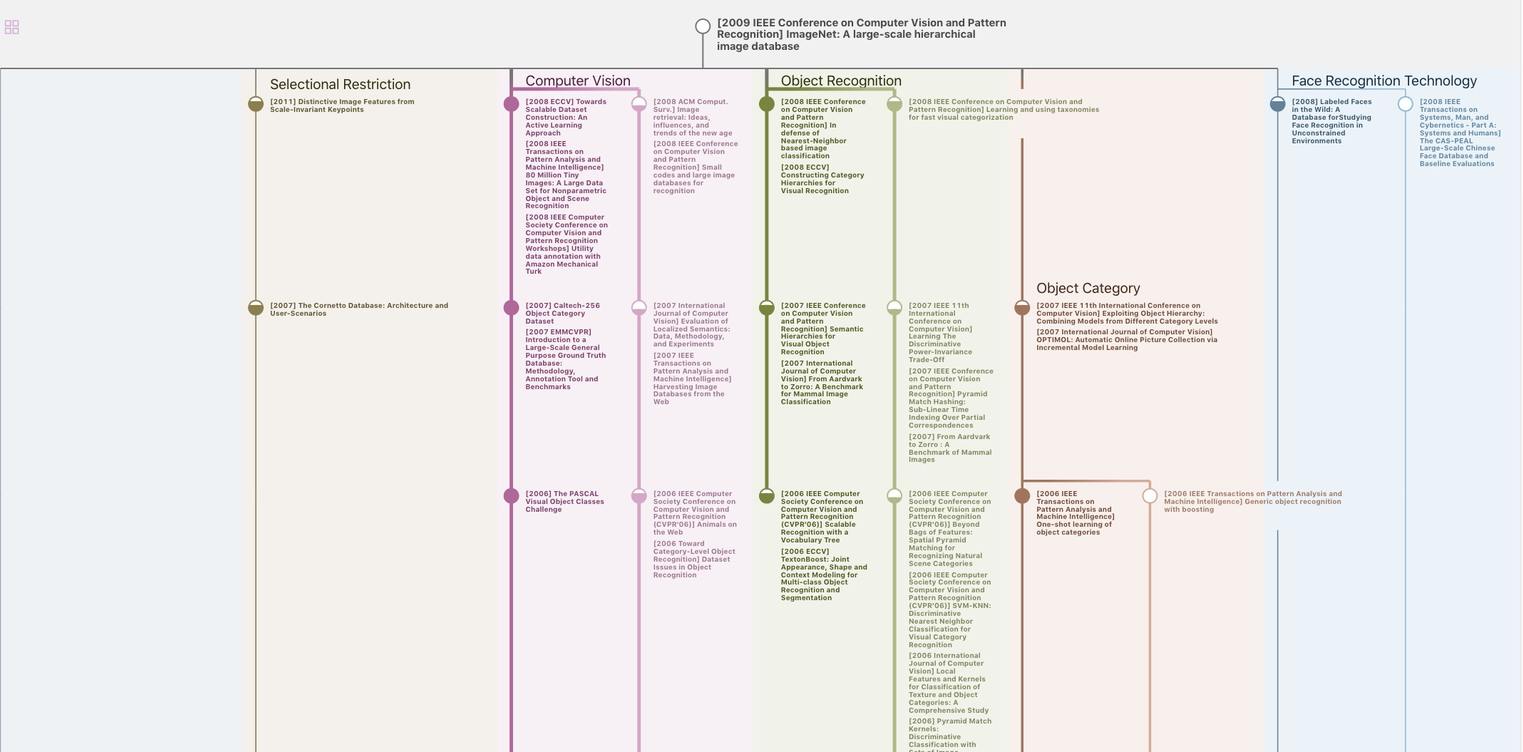
生成溯源树,研究论文发展脉络
Chat Paper
正在生成论文摘要