Inverse Design Method for Horn Antennas Based on Knowledge-Embedded Physics-Informed Neural Networks
IEEE Antennas and Wireless Propagation Letters(2024)
Abstract
This letter aims to overcome the challenges faced by Physics-Informed Neural Networks (PINNs) in metal structure design. Taking the design of horn antennas as an example, we propose a knowledge-embedded PINNs (KE-PINNs) inverse design framework. Normalized Maxwell's equations are employed to address convergence issues caused by differences in magnitudes. Unlike traditional PINNs, we built field component neural networks, inherently satisfying the boundary conditions of the metal structure. At the same time, the boundary conditions of the port and aperture fields are embedded within the neural network using a hard constraint boundary approach. The embedded knowledge further simplifies the loss function. The numerical experiments showcase the inverse design results of the H-plane horn antenna profile under different target aperture field requirements. The results demonstrate that the inverse-designed antennas effectively achieve the super-gain and beam deflection respectively, validating the feasibility and practical value of the proposed method.
MoreTranslated text
Key words
Inverse Problems,Inverse Design,Physics-Informed Neural Networks,Topology Optimization
AI Read Science
Must-Reading Tree
Example
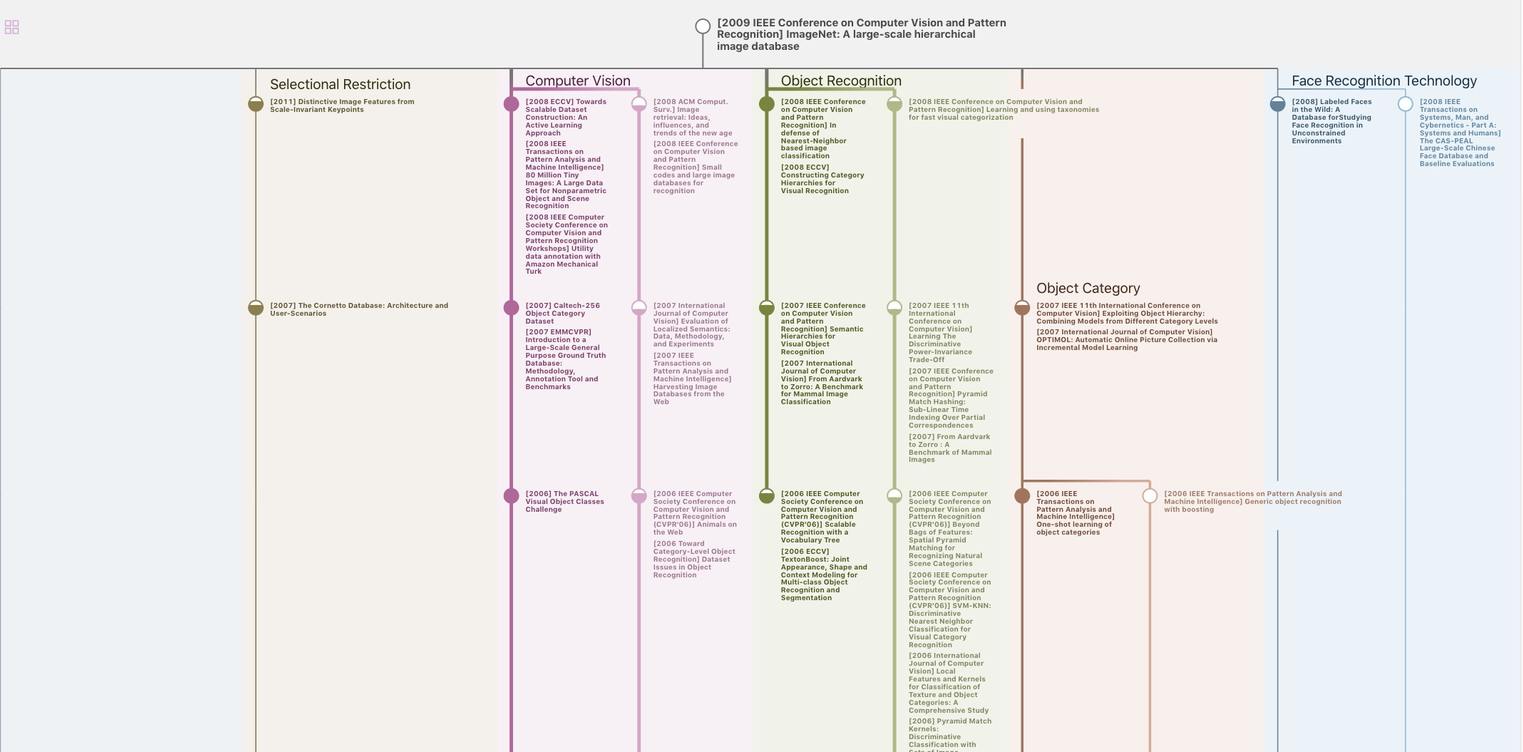
Generate MRT to find the research sequence of this paper
Chat Paper
Summary is being generated by the instructions you defined