Improving Beamforming Performance with Practical Phase Shifters Using Robust Mapping and Deep Learning
IEEE Transactions on Antennas and Propagation(2024)
摘要
In this paper, the problem of beamforming and maximizing the phased array gain for practical phase shifters is addressed using a deep learning approach. For an ideal phase shifter, the amplitude is constant and the output phase changes linearly with the control voltage. However, the practical phase shifters show a nonlinear behavior in both amplitude and phase with respect to the control voltage. The main objective of this paper is to design a deep neural network, which estimates the optimum voltage values of the phase shifter using the noisy data of the receiver antenna array. The proposed approach is general and extendible to any array geometry. Usually, the quality of the trained model is affected by large variations in the voltage values for certain adjacent Directions of Arrival (DOAs). To overcome this issue, we introduce the robust mapping technique, which avoids sudden changes in voltage values during the training process. The amplitude and phase data of a typical reflective-type phase shifter is measured for different control voltage values, to be used in the training process. The suggested algorithm is applied to a receiver array of four antennas with reflective type phase shifters. The maximum array gain for different DOAs are very close to the optimum results showing the superior performance of the proposed method. Compared to the existing methods, the RMSE of the array gain decreased to 0.0283. It is shown that the proposed method is more resilient to noise and performs substantially better than other methods in low SNR scenarios.
更多查看译文
关键词
Beamforming,phase shifter,phased array,deep learning,machine learning,neural network
AI 理解论文
溯源树
样例
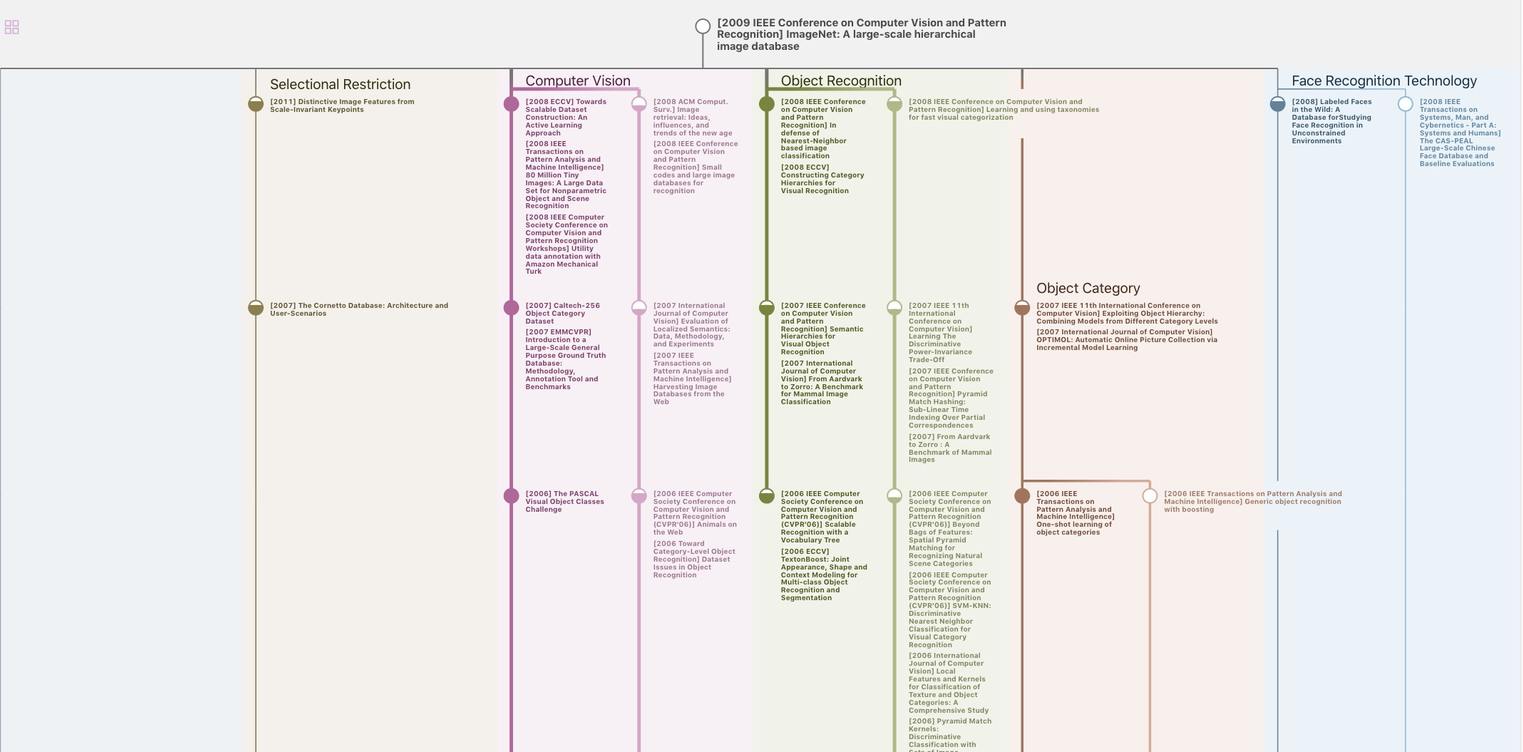
生成溯源树,研究论文发展脉络
Chat Paper
正在生成论文摘要