A Membership Inference and Adversarial Attack Defense Framework for Network Traffic Classifiers
IEEE Transactions on Artificial Intelligence(2024)
摘要
Malicious traffic identification methods in intrusion detection systems have evolved from rule-based matching to machine learning. However, security risks such as membership inference and adversarial attacks hinder the practical deployment of machine learning-based network intrusion detection systems (ML-NIDS). In this work, we design a defense framework called HierarchicalDP to safeguard ML-NIDS against membership inference and adversarial attacks. First, we analyse the principles of membership inference and adversarial attacks to find their correlation. Based on this, we propose the Feature Distribution Security Metric (FDSM) to measure the risk of membership inference and adversarial attacks on ML-NIDS. Then, we design the Hierarchical Differential Privacy (HierarchicalDP) framework, which partitions network traffic sample features according to security levels and introduces distinct noise on each security level feature to satisfy FDSM, thus defensing against membership inference and adversarial attacks. Finally, we evaluate the defensive performance of the HierarchicalDP framework on two network traffic datasets and four machine learning models. The HierarchicalDP defense framework, based on Laplace noise, reduces the success rate of membership inference from 64.9% to 54.4% (ineffective binary classification), the evasion rate of adversarial samples from 86.1% to 23.2%, and maintains model accuracy fluctuations within 4.2%. Furthermore, the HierarchicalDP framework adjusts sample features without modifying the model, thereby not affecting the inference speed. HierarchicalDP offers efficient and convenient defenses for ML-NIDS deployed in a network.
更多查看译文
关键词
Artificial intelligence in cyber-security,Artificial intelligence safety,Machine learning,Responsible artificial intelligence,Verifiable artificial intelligence
AI 理解论文
溯源树
样例
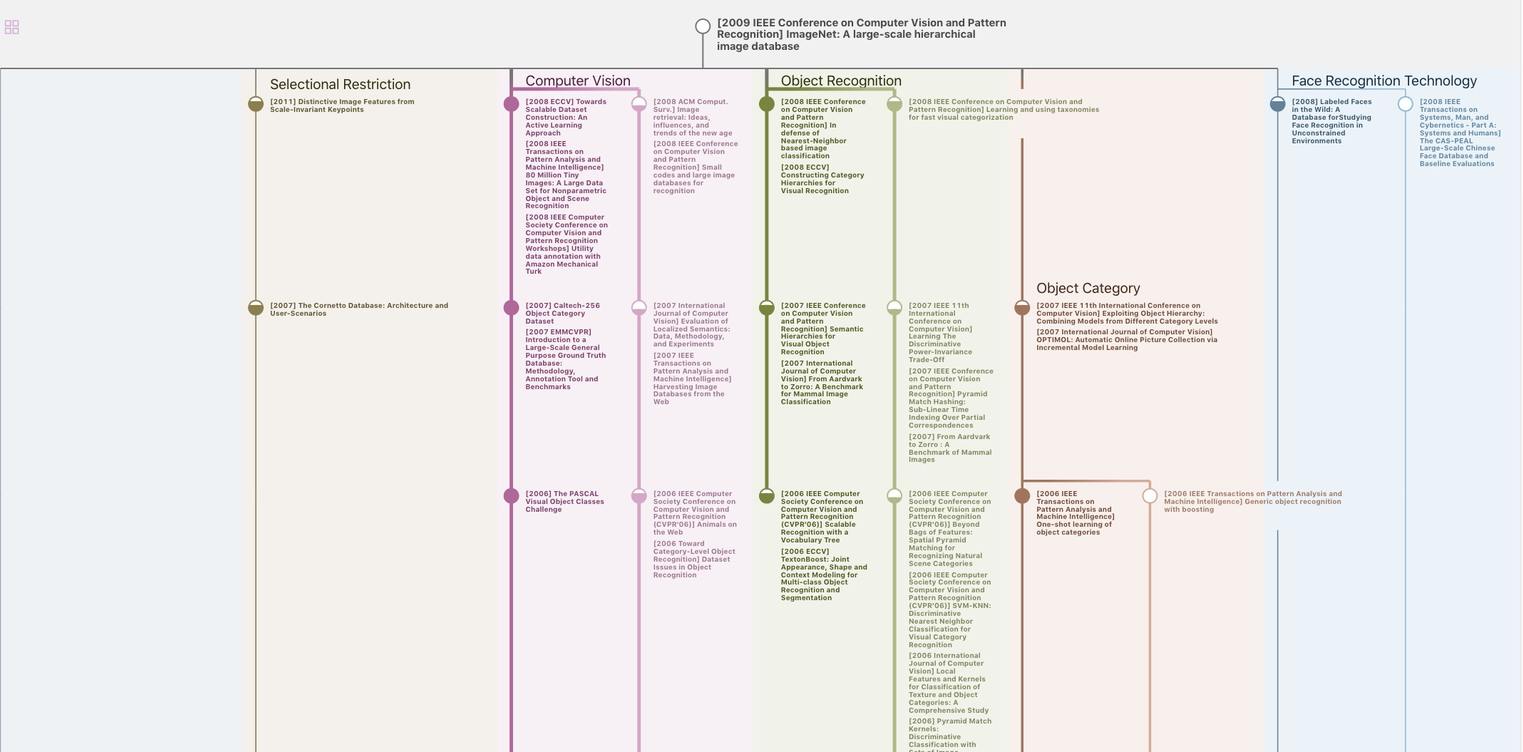
生成溯源树,研究论文发展脉络
Chat Paper
正在生成论文摘要