Enhance Adversarial Robustness via Geodesic Distance
IEEE Transactions on Artificial Intelligence(2024)
摘要
Adversarial training is an effective method to improve the model’s adversarial robustness. To realize a considerable tradeoff between clean accuracy and adversarial robustness, surrogate loss minimization can be utilized to regularize for robustness. This study advances beyond previous research efforts by taking a further step. It is informed by two theoretical perspectives. First, adversarial examples are inevitable within a unit sphere surrounding clean data. Second, geodesics can characterize the shortest distance between two points in Riemannian geometry. Accordingly, this study employs geodesic distance as a regularized surrogate loss item to capture the minimal divergence between the distribution of natural examples and the distribution of adversarial examples. This approach yields a more compact upper bound of risk errors than previous studies, which is beneficial for improving adversarial robustness. Based on the theoretical insight, this study proposes a metric of Geodesic Loss and a framework of Geodesic Adversarial Training to boost the adversarial robustness of neural networks. The empirical study on the diverse datasets demonstrates the considerable performance of the proposed method against diverse attacks, including white-box attacks, black-box corruptions, adaptive attacks, and auto attacks. Our code is available at:
https://github.com/momo1986/GeodesicAdversarialTraining
.
更多查看译文
关键词
Deep Learning,Adversarial Examples,Adversarial Training,Differential Geometry
AI 理解论文
溯源树
样例
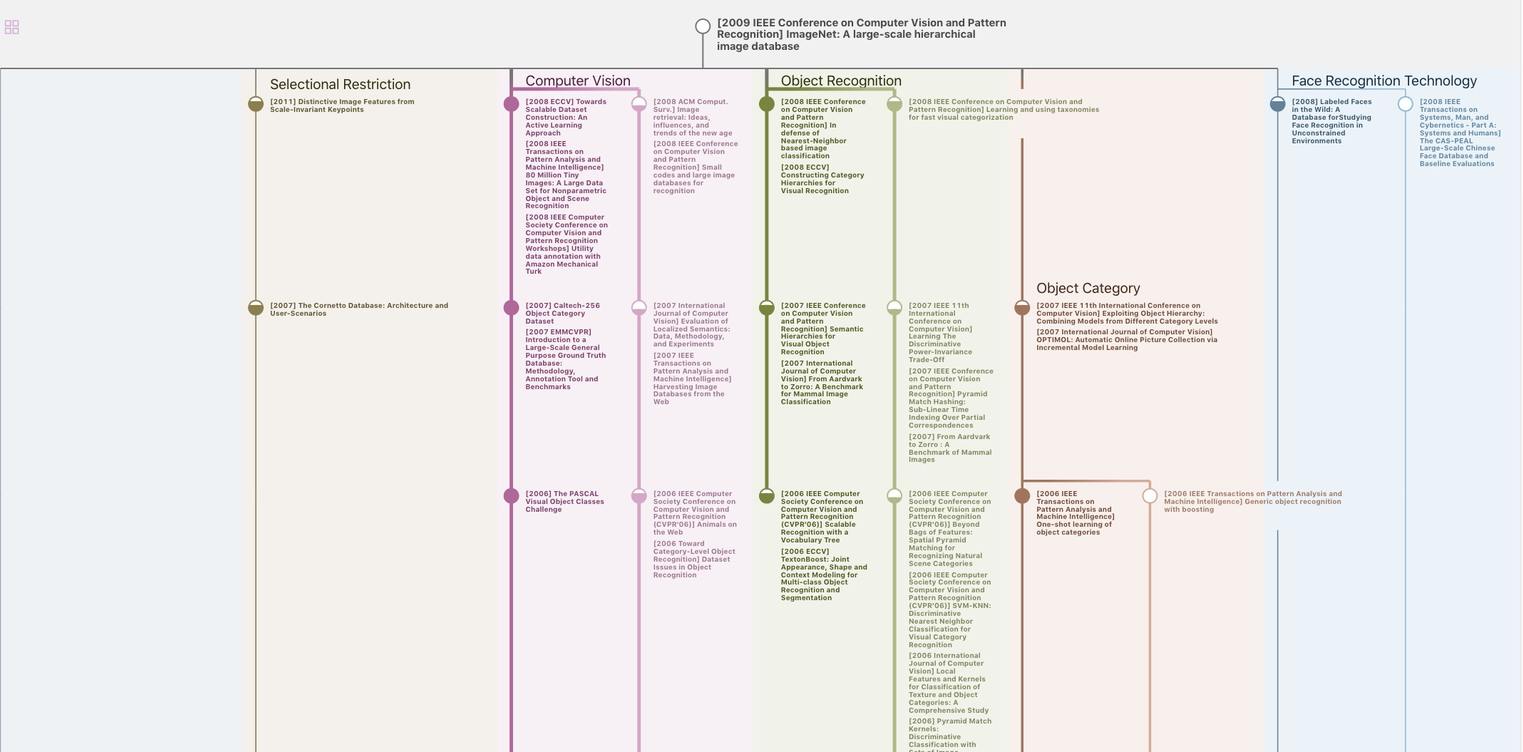
生成溯源树,研究论文发展脉络
Chat Paper
正在生成论文摘要