EXNet: (2+1)D Extreme Xception Net for Hyperspectral Image Classification
IEEE JOURNAL OF SELECTED TOPICS IN APPLIED EARTH OBSERVATIONS AND REMOTE SENSING(2024)
摘要
3-D CNNs have demonstrated their capability to capture intricate nonlinear relationships within hyperspectral images (HSIs). However, the computational complexity of 3-D CNNs often leads to slower processing speeds, limited generalization, and susceptibility to overfitting. In response to these challenges, this study introduces the concept of depthwise separable convolutions using (2+1)-D convolutions as an alternative to traditional 3-D convolutions for hyperspectral image classification (HSIC). The study observes that (2+1)-D convolutions can effectively approximate the complex relationships represented by 3-D convolutions while requiring fewer convolutional operations, thereby reducing the computational overhead associated with classification. Experimental results obtained from benchmark HSI datasets, including Indian Pines, Botswana, Pavia University, and Salinas, demonstrate that the proposed model yields results that are comparable to those achieved by various state-of-the-art models in the existing literature.
更多查看译文
关键词
(2+1)-D convolutions,deep neural network,depthwise separable convolutions,inception,Xception
AI 理解论文
溯源树
样例
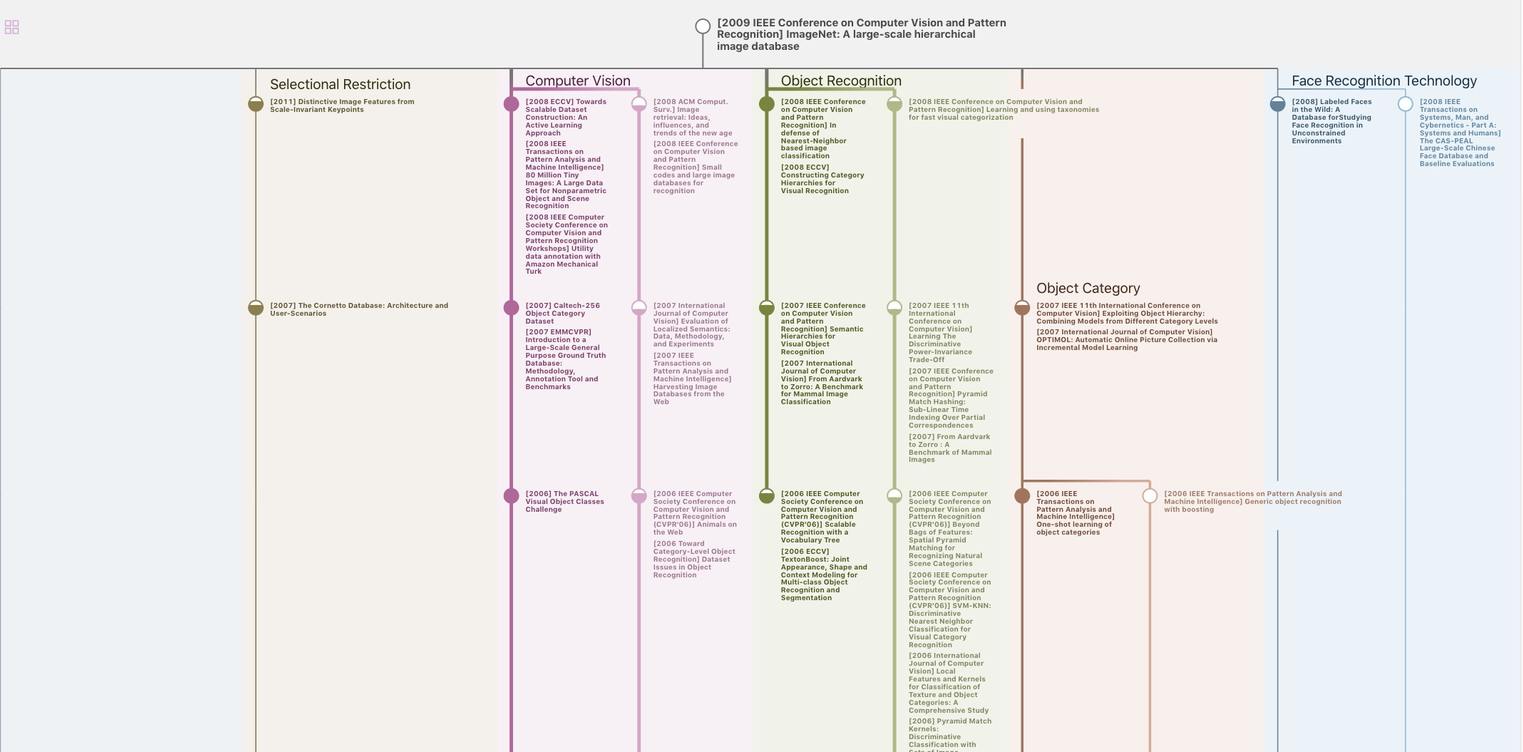
生成溯源树,研究论文发展脉络
Chat Paper
正在生成论文摘要