Diffusion-based Neural Network Weights Generation
CoRR(2024)
摘要
Transfer learning is a topic of significant interest in recent deep learning
research because it enables faster convergence and improved performance on new
tasks. While the performance of transfer learning depends on the similarity of
the source data to the target data, it is costly to train a model on a large
number of datasets. Therefore, pretrained models are generally blindly selected
with the hope that they will achieve good performance on the given task. To
tackle such suboptimality of the pretrained models, we propose an efficient and
adaptive transfer learning scheme through dataset-conditioned pretrained
weights sampling. Specifically, we use a latent diffusion model with a
variational autoencoder that can reconstruct the neural network weights, to
learn the distribution of a set of pretrained weights conditioned on each
dataset for transfer learning on unseen datasets. By learning the distribution
of a neural network on a variety pretrained models, our approach enables
adaptive sampling weights for unseen datasets achieving faster convergence and
reaching competitive performance.
更多查看译文
AI 理解论文
溯源树
样例
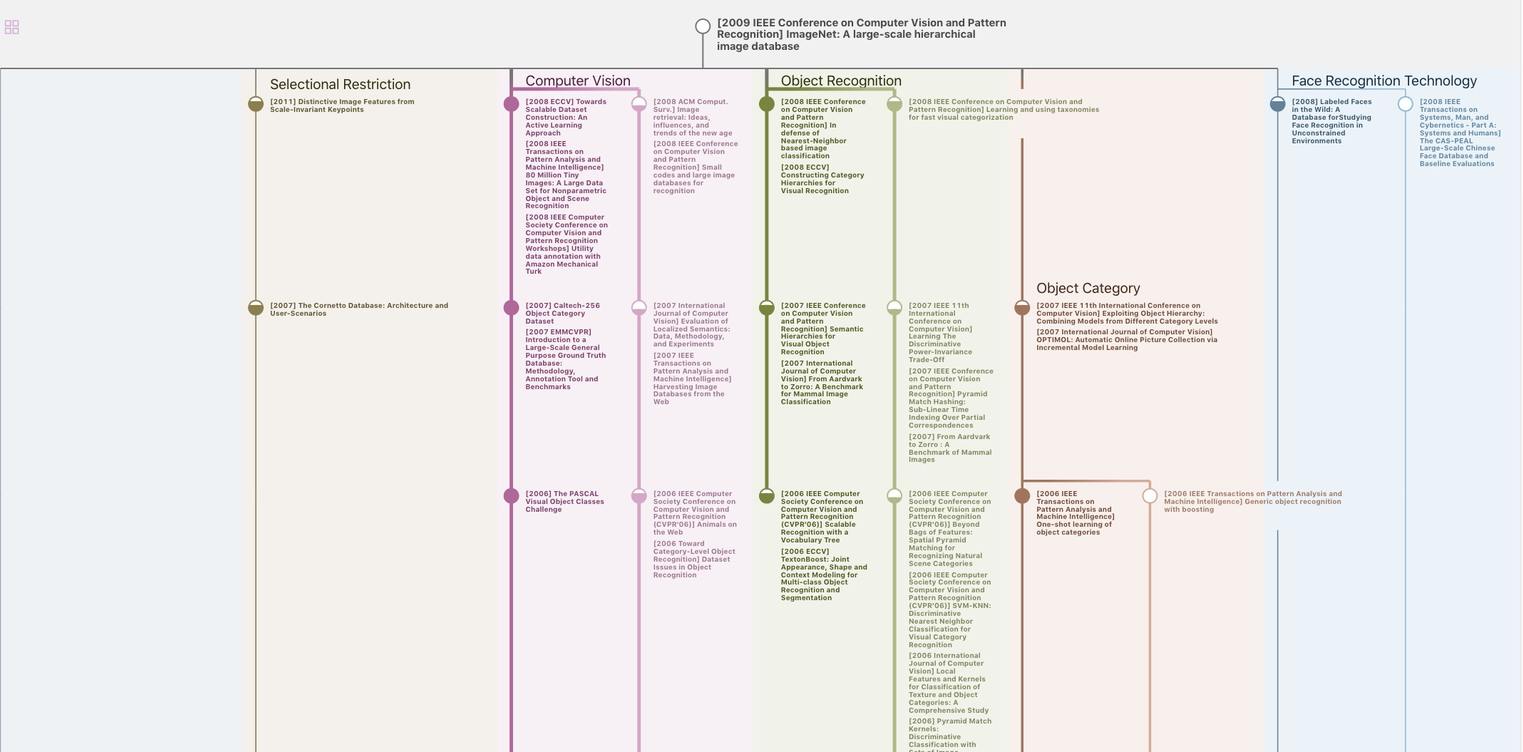
生成溯源树,研究论文发展脉络
Chat Paper
正在生成论文摘要