Learning Intrinsic Dimension via Information Bottleneck for Explainable Aspect-based Sentiment Analysis
CoRR(2024)
摘要
Gradient-based explanation methods are increasingly used to interpret neural
models in natural language processing (NLP) due to their high fidelity. Such
methods determine word-level importance using dimension-level gradient values
through a norm function, often presuming equal significance for all gradient
dimensions. However, in the context of Aspect-based Sentiment Analysis (ABSA),
our preliminary research suggests that only specific dimensions are pertinent.
To address this, we propose the Information Bottleneck-based Gradient
() explanation framework for ABSA. This framework leverages an
information bottleneck to refine word embeddings into a concise intrinsic
dimension, maintaining essential features and omitting unrelated information.
Comprehensive tests show that our approach considerably improves
both the models' performance and interpretability by identifying
sentiment-aware features.
更多查看译文
AI 理解论文
溯源树
样例
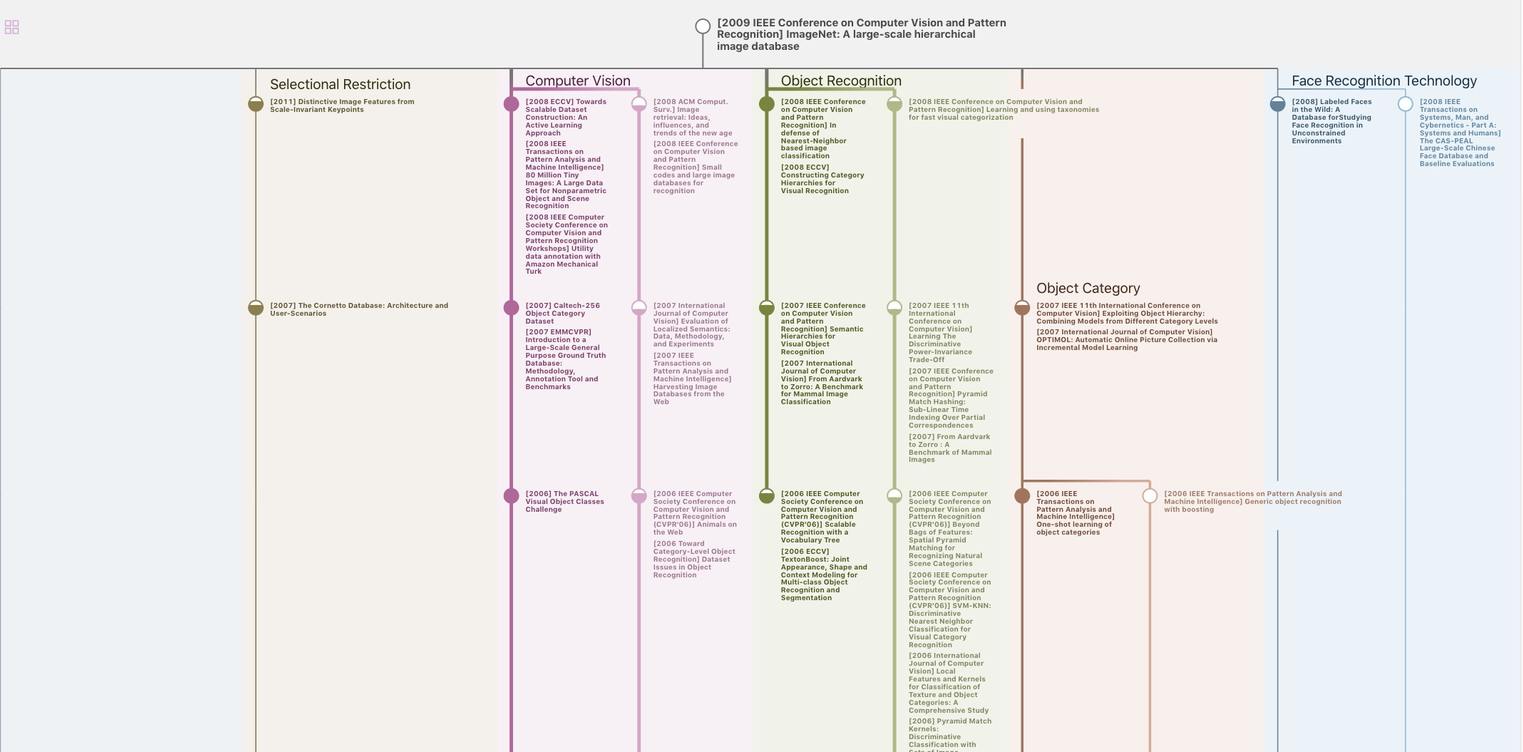
生成溯源树,研究论文发展脉络
Chat Paper
正在生成论文摘要