From Simulations to Reality: Dark Energy Reconstruction with Simulated SNIa data from the Vera C. Rubin Observatory
arxiv(2024)
摘要
In this paper, we present an Artificial Neural Network (ANN) based
reconstruction analysis of the Supernova Ia (SNIa) distance moduli (μ(z)),
and hence dark energy, using LSST simulated three-year SNIa data. Our ANN
reconstruction architecture can model both the distance moduli and their
corresponding error estimates. For this we employ astroANN and incorporate
Monte Carlo dropout techniques to quantify uncertainties in our predictions. We
tune our hyperparameters through advanced genetic algorithms, including
elitism, utilizing the DEAP library. We compared the performance of the ANN
based reconstruction with two theoretical descriptions of dark energy models,
ΛCDM and Chevallier-Linder-Polarski (CPL). We perform a Bayesian
analysis for these two theoretical models using the LSST simulations and also
compare with observations from Pantheon and Pantheon+ SNIa real data. We show
that our model-independent reconstruction using ANN is consistent with both of
them. We assessed the performance using mean squared error (MSE) and showed
that the ANN can produce distance estimates in better agreement with the LSST
dataset than either ΛCDM or CPL, albeit very small. We included an
additional residual analysis and a null test with F-scores to show that the
reconstructed distances from the ANN model, are in excellent agreement with the
ΛCDM or CPL model.
更多查看译文
AI 理解论文
溯源树
样例
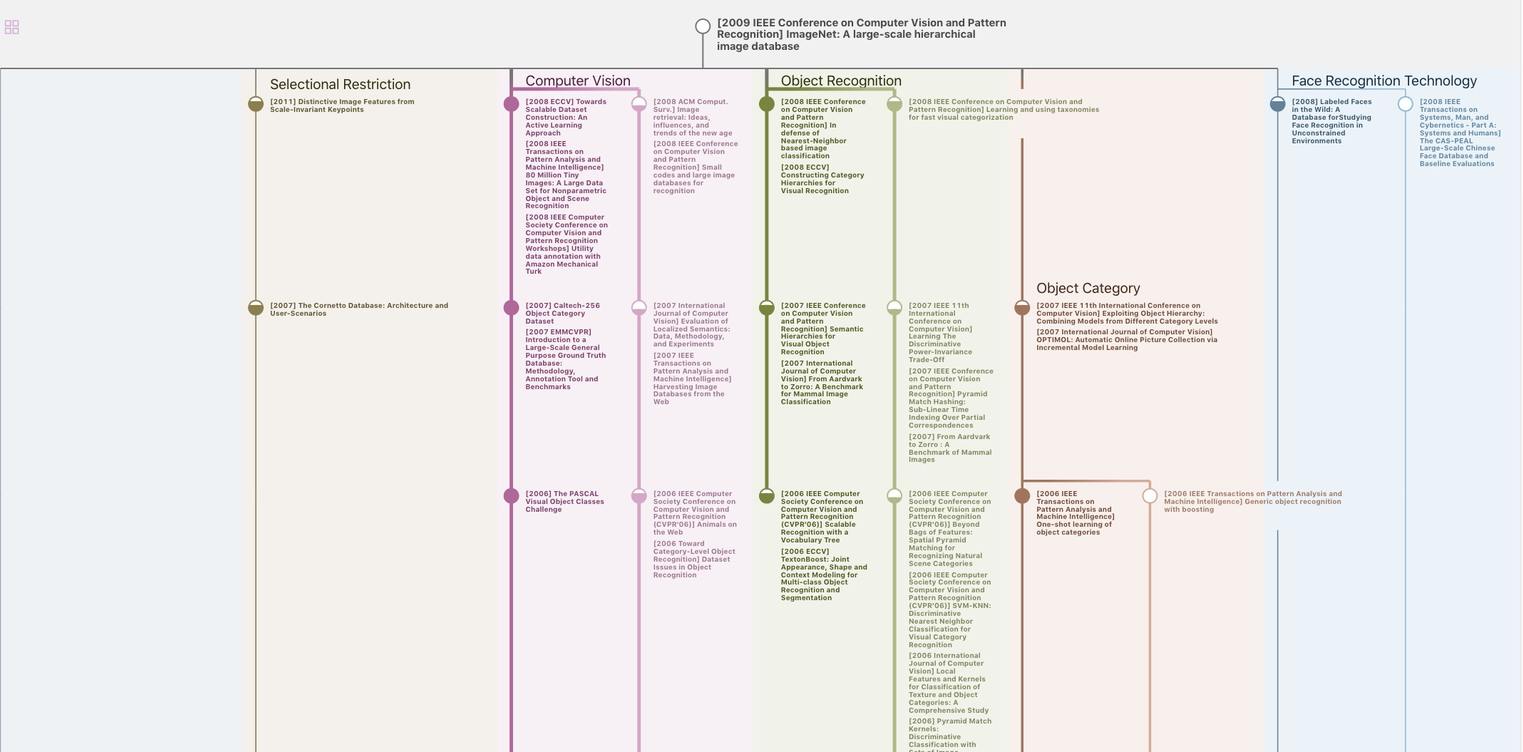
生成溯源树,研究论文发展脉络
Chat Paper
正在生成论文摘要