Explainable AI for Tomato Leaf Disease Detection: Insights into Model Interpretability
2023 26th International Conference on Computer and Information Technology (ICCIT)(2023)
摘要
Plant diseases pose a significant threat to global food security by limiting access to safe and abundant food sources while impacting agricultural productivity and food safety. To address this challenge, innovative disease detection techniques are crucial. This paper presents a novel approach to tomato leaf disease detection grounded in deep transfer learning. We employ convolutional neural networks (CNNs) to recognize and categorize diseases, leveraging pre-trained models such as EfficientNetB3, Xception, and MobileNetV2. Our experimental results highlight the efficacy of these models, with the CNN achieving an accuracy of 0.92, MobileNetV2 at 0.93, Xception at 0.94 and EfficientNetB3 at 0.993. By combining these machine learning techniques with image processing methods, we create a system capable of diagnosing tomato leaf diseases promptly. The system employs image processing techniques to facilitate disease recognition, enabling automated disease identification as soon as symptoms manifest on leaves or plants. This empowers farmers with timely information and appropriate treatment options, ultimately leading to precise disease management. Furthermore, our research delves into model interpretability by employing Saliency maps and Grad-CAM techniques, providing valuable insights into the decision-making process of our deep learning models. This transparency enhances the trustworthiness of the AI-driven disease detection system, fostering its practical application in agriculture.
更多查看译文
关键词
Image processing,Machine learning techniques,Image diagnosis,Convolutional neural network
AI 理解论文
溯源树
样例
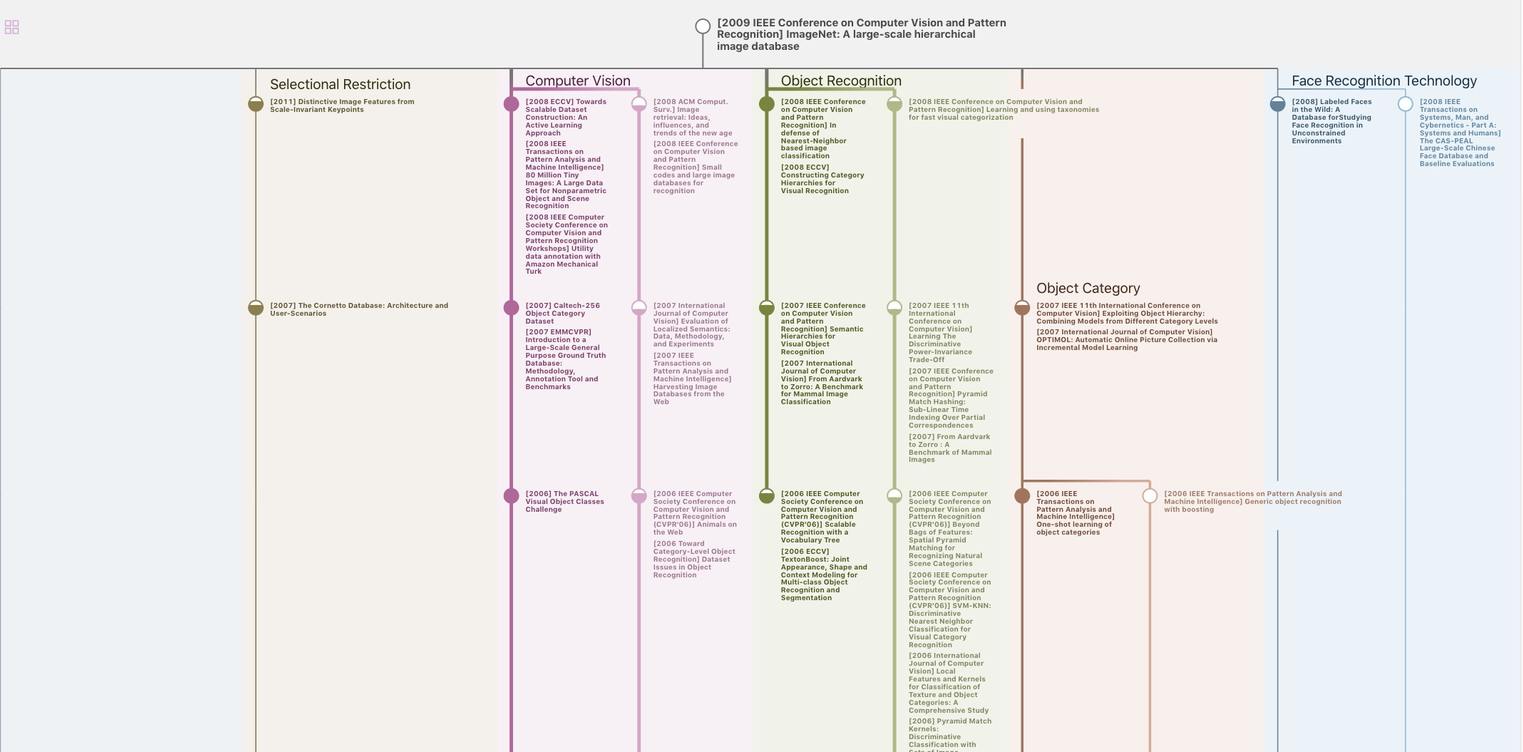
生成溯源树,研究论文发展脉络
Chat Paper
正在生成论文摘要