Augmented EEG Signal Classification for Energy Data Visualizations
2023 International Conference on Electrical, Communication and Computer Engineering (ICECCE)(2023)
摘要
Classification of electroencephalogram (EEG) data is dependent on data size, quality, and generalizability to decode invaluable information from brain-computer interface (BCI) systems. EEG data collection typically occurs within controlled laboratory environments using long sections. Participation in these experiments draws less attention due to the challenging nature of the EEG experiments and the intended objective. Hence, an approach that offers the potential for both cost and time efficiency is the use of artificial data generation. Data augmentation can improve the classification performance by increasing training data. This study employs a Generative Adversarial Network (GAN) model to enhance the empirically collected EEG dataset, which was introduced for the purpose of analyzing the perceptions of energy users regarding energy data visualizations. The data size is initially expanded through the use of a GAN model. Subsequently, the augmented data is assessed in terms of its classification performance. Three different sample groups—defined as male, female, and mixed—are shown in the results. Each group is used to generate synthetic EEGs, separately. To evaluate the enhanced EEG data, a scenario of transforming EEG data into spectrogram images and then classifying them using a Convolutional Neural Network (CNN) is used. The results show that the female sample group performed the best in terms of valence and arousal, with accuracies of 87.9% and 89.06%, respectively. This study shows promising results in terms of increasing EEG data size and improving classification performance.
更多查看译文
关键词
Brain-computer interface,Data visualization,Energy,Electroencephalography,Generative Adversarial Network,Augmentation
AI 理解论文
溯源树
样例
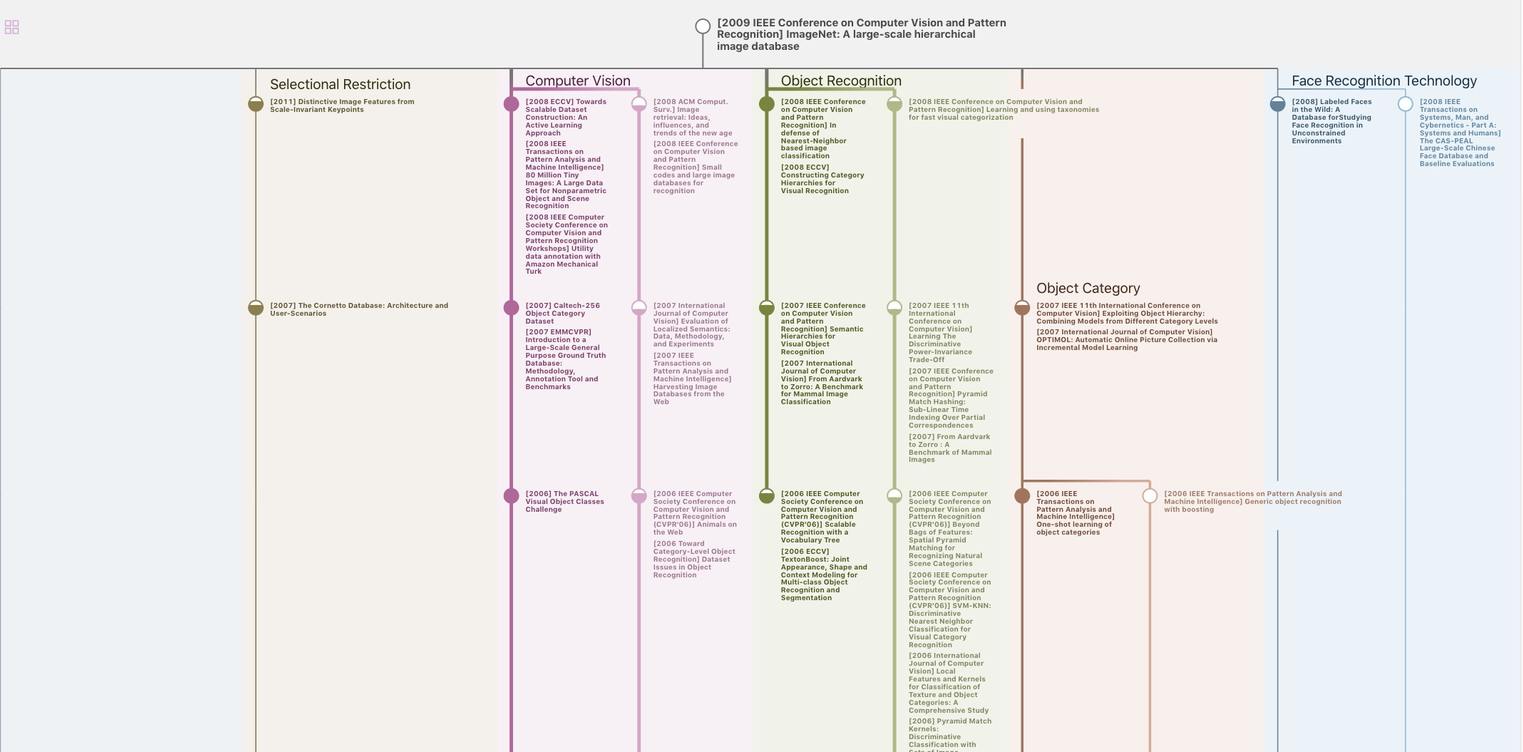
生成溯源树,研究论文发展脉络
Chat Paper
正在生成论文摘要