Exploring the Potential: ML vs. DL in Network Security with Explainable AI (XAI) Insights
2023 26th International Conference on Computer and Information Technology (ICCIT)(2023)
Abstract
The increasing complexity and diversity of cyber threats have driven the widespread adoption of Machine Learning (ML) and Deep Learning (DL) techniques for Network Intrusion Detection Systems (NIDSs) due to their capacity to effectively adapt and detect evolving threats. However, the inherent black-box nature of ML and DL-powered NIDSs has raised concerns surrounding explainability, transparency, and interpretability. This challenge has led to a prevailing sense of distrust in these systems. In this paper, we propose an improved Network Intrusion Detection System (NIDS) and introduce a ML and a DL-based classifier to detect malicious network traffics. To overcome the explainibility and trust issues, we innovatively integrate advanced Explainable Artificial Intelligence (XAI) techniques, such as SHAP, LIME, and ELI5, with our proposed ML and DL classifiers. These techniques provide vital insights and detailed explanations behind the decision-making processes of the NIDS. This approach is pivotal for multiple reasons, including ensuring that the classifiers are fair and unbiased, articulating the rationale behind their outcomes, or for debugging the models when they make mistakes. Furthermore, we evaluate and compare the performance of ML and DL classifiers using a variety of metrics such as Accuracy, Precision, Recall, F1-score, ROC AUC curve, PR AUC, Training time, Confusion matrix, and also compare the explainability the classifiers. The need to compare ML and DL-based classifiers stems from the aim to optimize network security measures, improve threat detection, ensure that resources are used efficiently in the face of evolving cyber threats, and understanding the differences in their decision-making processes. In this study, the ML Classifier (Accuracy 94.5%) outperformed the DL Classifier (Accuracy 93.5%).
MoreTranslated text
Key words
Network Security,Network Intrusion Detection Systems,ML,DL,Explainable AI,Cyber Threat Intelligence
AI Read Science
Must-Reading Tree
Example
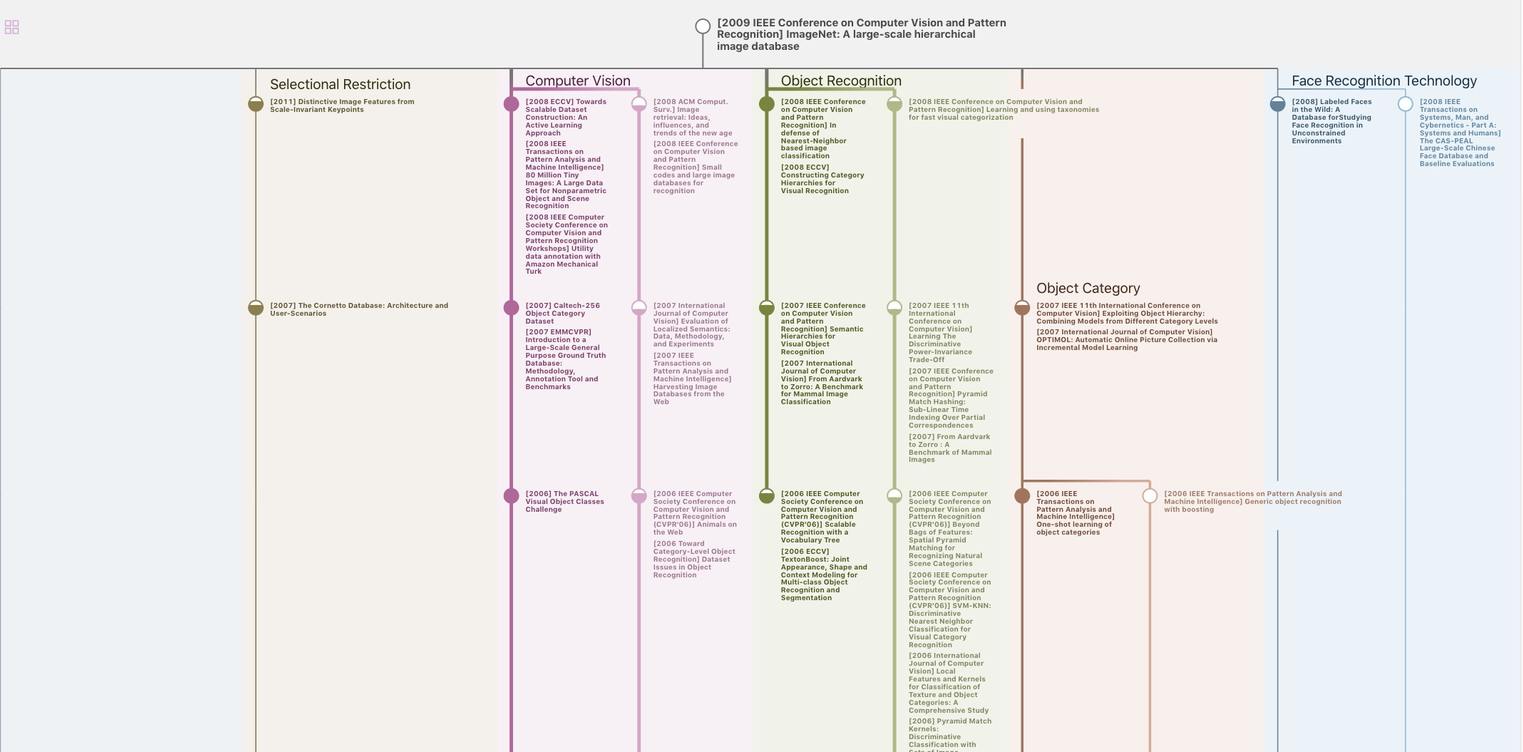
Generate MRT to find the research sequence of this paper
Chat Paper
Summary is being generated by the instructions you defined