Clinical risk prediction using language models: benefits and considerations
JOURNAL OF THE AMERICAN MEDICAL INFORMATICS ASSOCIATION(2024)
摘要
Objective The use of electronic health records (EHRs) for clinical risk prediction is on the rise. However, in many practical settings, the limited availability of task-specific EHR data can restrict the application of standard machine learning pipelines. In this study, we investigate the potential of leveraging language models (LMs) as a means to incorporate supplementary domain knowledge for improving the performance of various EHR-based risk prediction tasks.Methods We propose two novel LM-based methods, namely "LLaMA2-EHR" and "Sent-e-Med." Our focus is on utilizing the textual descriptions within structured EHRs to make risk predictions about future diagnoses. We conduct a comprehensive comparison with previous approaches across various data types and sizes.Results Experiments across 6 different methods and 3 separate risk prediction tasks reveal that employing LMs to represent structured EHRs, such as diagnostic histories, results in significant performance improvements when evaluated using standard metrics such as area under the receiver operating characteristic (ROC) curve and precision-recall (PR) curve. Additionally, they offer benefits such as few-shot learning, the ability to handle previously unseen medical concepts, and adaptability to various medical vocabularies. However, it is noteworthy that outcomes may exhibit sensitivity to a specific prompt.Conclusion LMs encompass extensive embedded knowledge, making them valuable for the analysis of EHRs in the context of risk prediction. Nevertheless, it is important to exercise caution in their application, as ongoing safety concerns related to LMs persist and require continuous consideration.
更多查看译文
关键词
electronic health records,risk prediction,large language models,substance use disorder,opioid use disorder
AI 理解论文
溯源树
样例
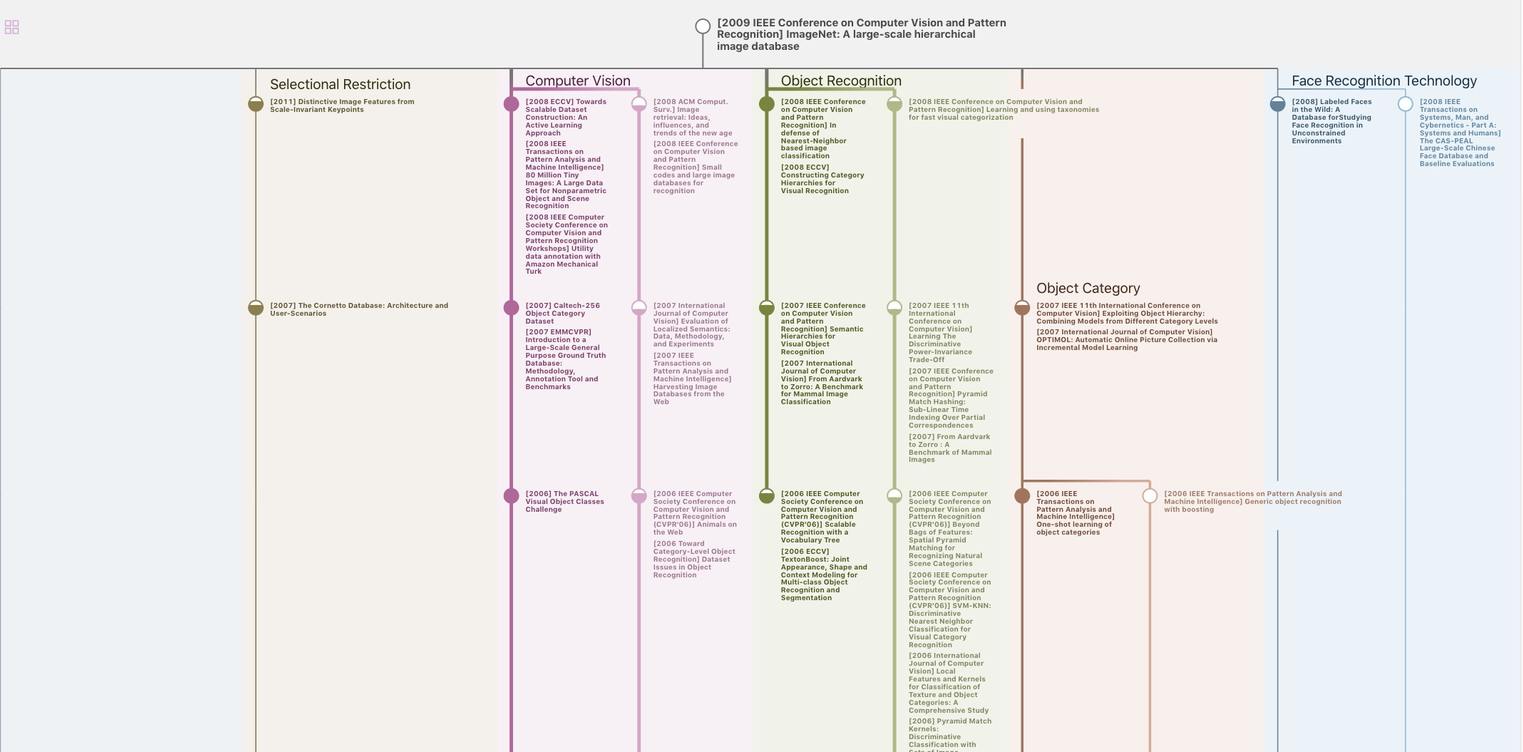
生成溯源树,研究论文发展脉络
Chat Paper
正在生成论文摘要