Comparison of clinical geneticist and computer visual attention in assessing genetic conditions
PLOS GENETICS(2024)
摘要
Artificial intelligence (AI) for facial diagnostics is increasingly used in the genetics clinic to evaluate patients with potential genetic conditions. Current approaches focus on one type of AI called Deep Learning (DL). While DL- based facial diagnostic platforms have a high accuracy rate for many conditions, less is understood about how this technology assesses and classifies (categorizes) images, and how this compares to humans. To compare human and computer attention, we performed eye-tracking analyses of geneticist clinicians (n = 22) and non-clinicians (n = 22) who viewed images of people with 10 different genetic conditions, as well as images of unaffected individuals. We calculated the Intersection-over-Union (IoU) and Kullback-Leibler divergence (KL) to compare the visual attentions of the two participant groups, and then the clinician group against the saliency maps of our deep learning classifier. We found that human visual attention differs greatly from DL model's saliency results. Averaging over all the test images, IoU and KL metric for the successful (accurate) clinician visual attentions versus the saliency maps were 0.15 and 11.15, respectively. Individuals also tend to have a specific pattern of image inspection, and clinicians demonstrate different visual attention patterns than non-clinicians (IoU and KL of clinicians versus non-clinicians were 0.47 and 2.73, respectively). This study shows that humans (at different levels of expertise) and a computer vision model examine images differently. Understanding these differences can improve the design and use of AI tools, and lead to more meaningful interactions between clinicians and AI technologies. Artificial intelligence (AI) is increasingly used in medicine. In clinical practice, medical geneticists often use AI tools to help them examine the facial features of a patient who might have a genetic condition. While these tools are very popular, less is understood about how they work, and which parts of the face are most important to the AI tools, especially compared to medical geneticists. To address this, we performed a study where medical geneticists (as well as non-clinicians) looked at pictures of people with and without genetic conditions. We used eye-tracking tools to visualize which parts of the images the medical geneticists preferentially looked at. We compared this to the parts of the image that were the most important to the AI tools. We found that the medical geneticists and the AI tools tended to pay attention to very different features. We also found that medical geneticists and non-clinicians look at different parts of the images. Understanding how AI tools examine images compared to clinicians can help ensure the tools function properly, and could also help perform tasks like alerting medical geneticists to important clinical findings that could otherwise be overlooked.
更多查看译文
AI 理解论文
溯源树
样例
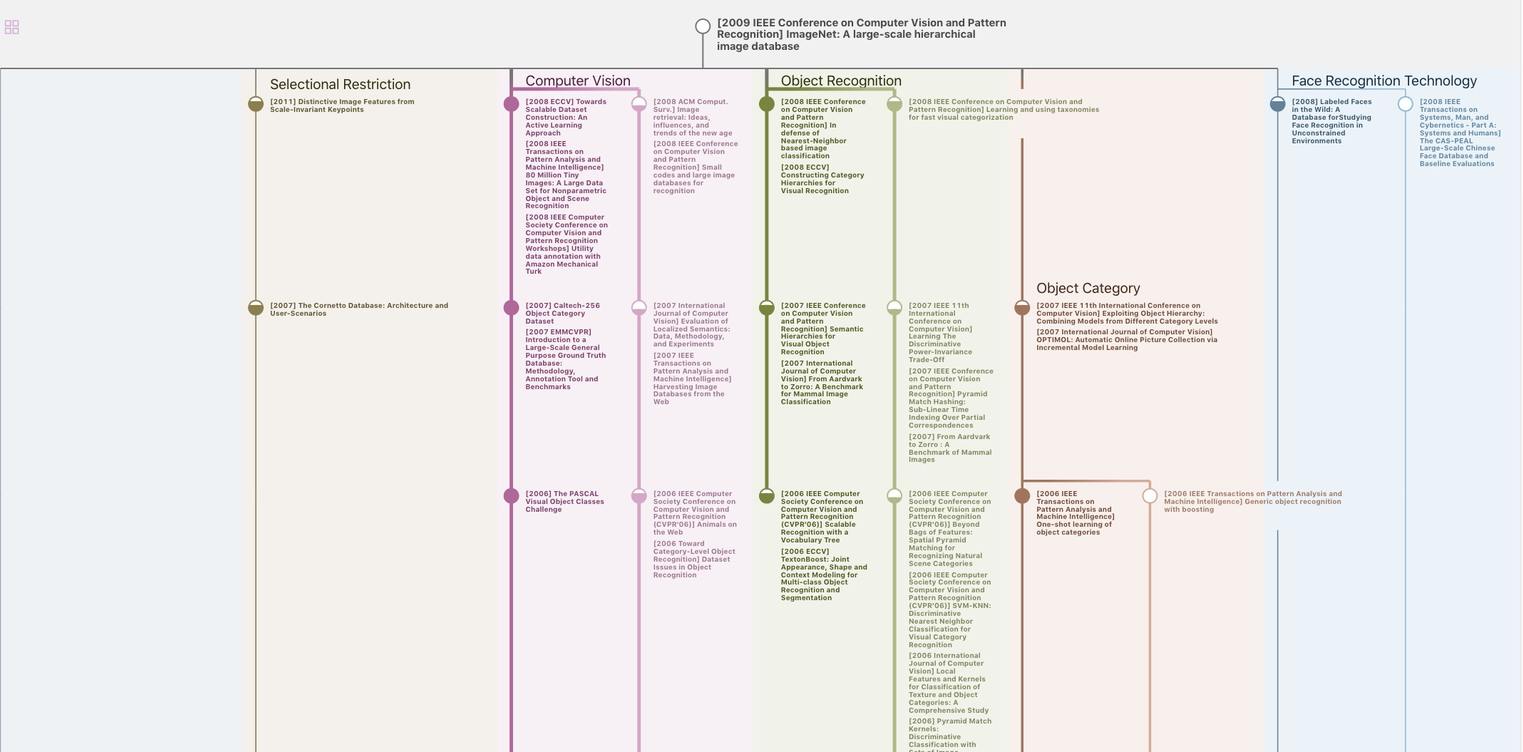
生成溯源树,研究论文发展脉络
Chat Paper
正在生成论文摘要