Source-Free Domain Adaptation With Domain Generalized Pretraining for Face Anti-Spoofing.
IEEE transactions on pattern analysis and machine intelligence(2024)
摘要
Source-free domain adaptation (SFDA) shows the potential to improve the generalizability of deep learning-based face anti-spoofing (FAS) while preserving the privacy and security of sensitive human faces. However, existing SFDA methods are significantly degraded without accessing source data due to the inability to mitigate domain and identity bias in FAS. In this paper, we propose a novel Source-free Domain Adaptation framework for FAS (SDA-FAS) that systematically addresses the challenges of source model pre-training, source knowledge adaptation, and target data exploration under the source-free setting. Specifically, we develop a generalized method for source model pre-training that leverages a causality-inspired PatchMix data augmentation to diminish domain bias and designs the patch-wise contrastive loss to alleviate identity bias. For source knowledge adaptation, we propose a contrastive domain alignment module to align conditional distribution across domains with a theoretical equivalence to adaptation based on source data. Furthermore, target data exploration is achieved via self-supervised learning with patch shuffle augmentation to identify unseen attack types, which is ignored in existing SFDA methods. To our best knowledge, this paper provides the first full-stack privacy-preserving framework to address the generalization problem in FAS. Extensive experiments on nineteen cross-dataset scenarios show our framework considerably outperforms state-of-the-art methods.
更多查看译文
关键词
Domain generalized pretraining,face anti-spoofing,source-free domain adaptation
AI 理解论文
溯源树
样例
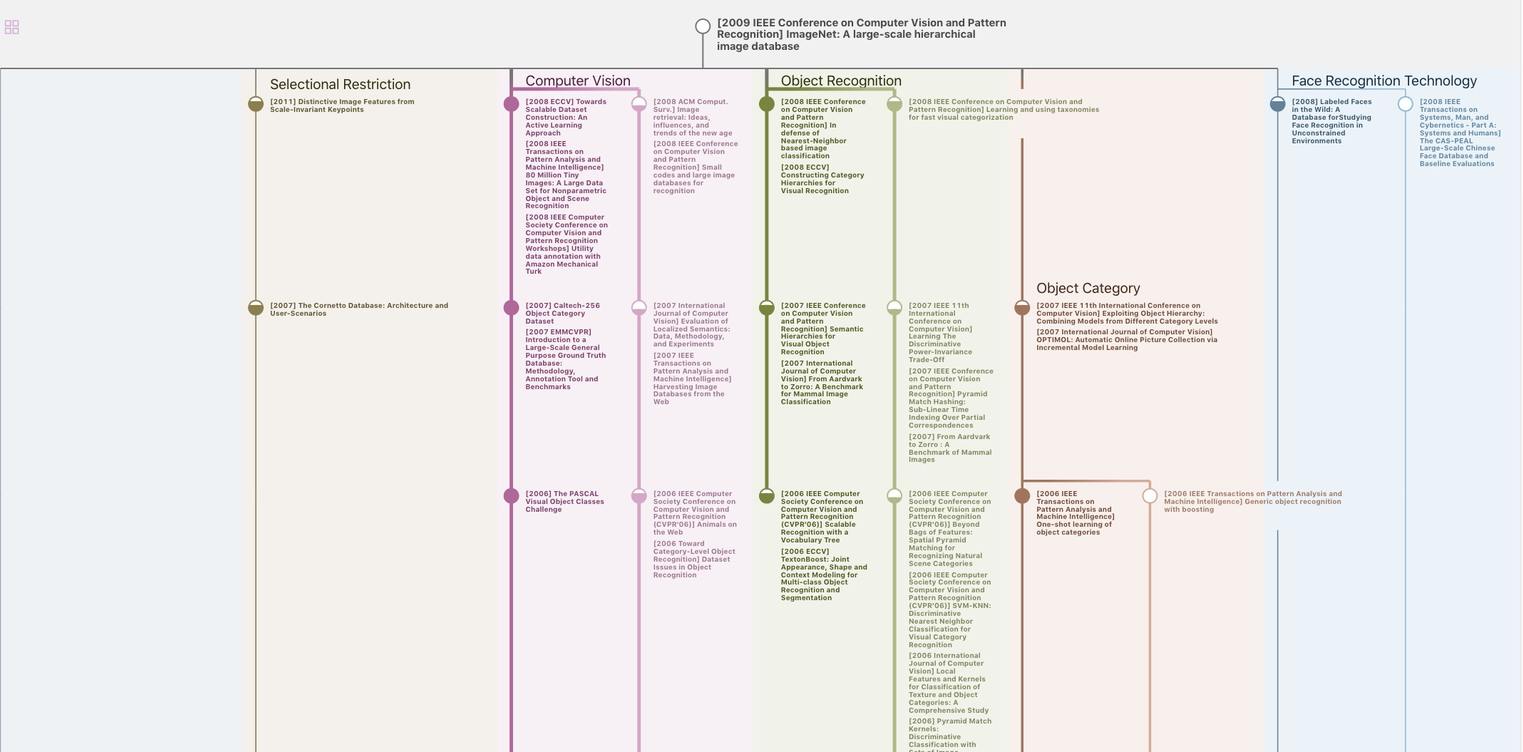
生成溯源树,研究论文发展脉络
Chat Paper
正在生成论文摘要