Enhanced Convolutional Neural Networks based on Bonferroni mean operator and their different combination in the Pooling layer
2023 IEEE 20th India Council International Conference (INDICON)(2023)
摘要
Convolutional neural networks (CNN) employ the classical maximum or arithmetic mean function to suppress the convolutional layer’s extracted features in the down-sampling procedure. However, both classical pooling functions are not able to model possible dependencies among the data values to be reduced in the process. With this view, in this study, we aim to improve CNN by replacing the classical pooling aggregation with Bonferroni mean-type (BM) aggregation operators, which model the dependency among the features by considering the pairwise contribution of the significant data values required to assess the data dependency on their neighborhood information. The use of different variations of Bonferroni mean functions and their composite functions are analyzed in the pooling layer. To empirically show the working of BM aggregation in the pooling layer, experiments on various models of different complexity are performed by choosing real and medical datasets, and the results are compared with the classical pooling functions and other state-of-the-art approaches. It is observed that the utilization of the BM-type pooling aggregation function outperforms most of them, and computational complexity is analogous to the classical functions.
更多查看译文
关键词
CNN,Bonferroni mean,Pooling functions,Composite aggregation functions,Classification,Segmentation
AI 理解论文
溯源树
样例
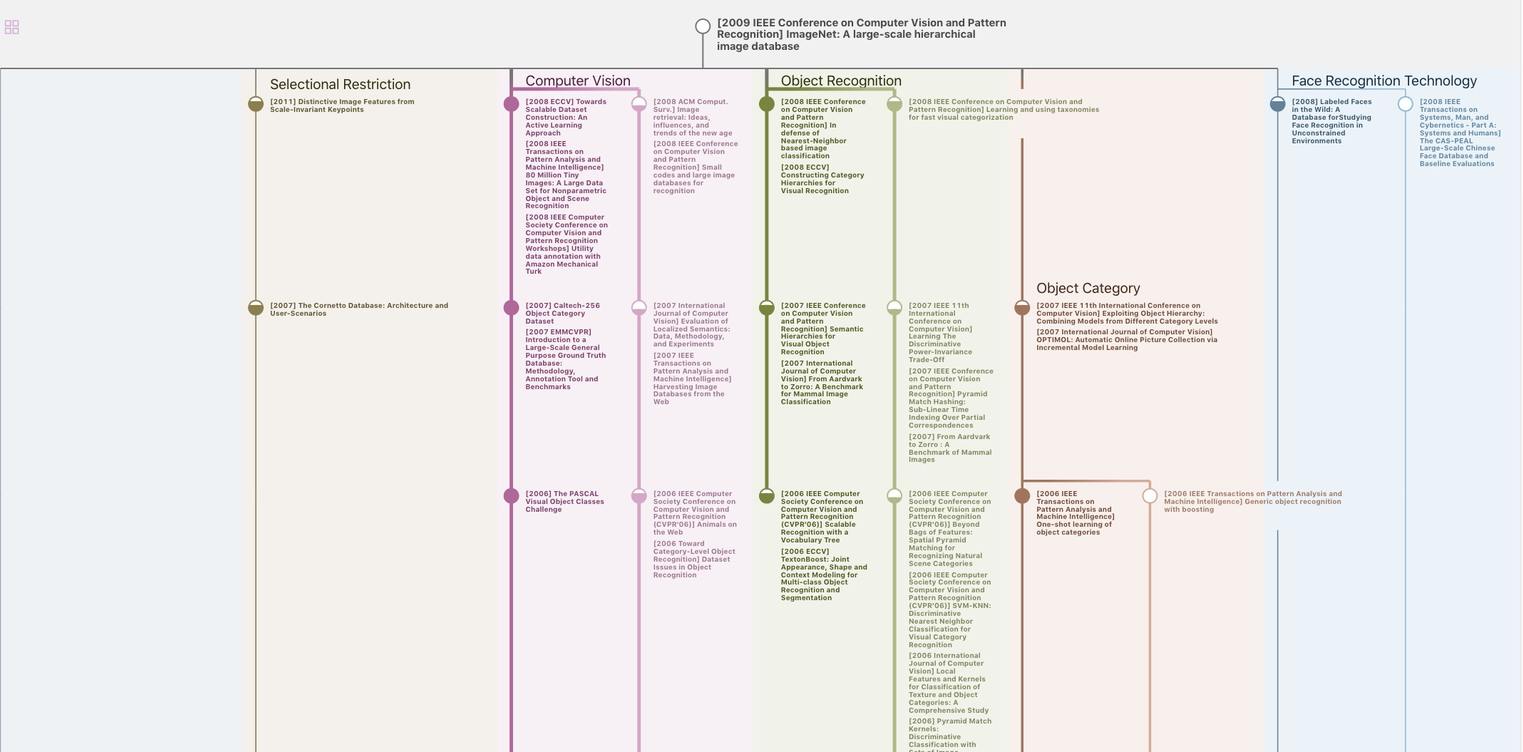
生成溯源树,研究论文发展脉络
Chat Paper
正在生成论文摘要