Convergence of Momentum-based Distributed Stochastic Approximation with RL Applications
2023 NINTH INDIAN CONTROL CONFERENCE, ICC(2023)
摘要
We develop a novel proof strategy for deriving almost sure convergence of momentum-based distributed stochastic approximation (DSA) schemes. Popular momentum-based schemes such as Polyak's heavy-ball and Nesterov's Accelerated SGD can be analyzed using our template. Our technique enables us to do away with three restrictive assumptions of existing approaches. One, we do not need the communication matrix to be doubly stochastic. Two, we do not need the noise to be uniformly bounded. Lastly, our approach can handle cases where there are multiple or non-point attractors. As an application, we use our technique to derive convergence for momentum-based extensions of the multi-agent TD(0) algorithm, where the above restrictive assumptions do not hold.
更多查看译文
关键词
Stochastic Approximation,Application Of Reinforcement Learning,Convergence Rate,Update Rule,Multi-agent Reinforcement Learning
AI 理解论文
溯源树
样例
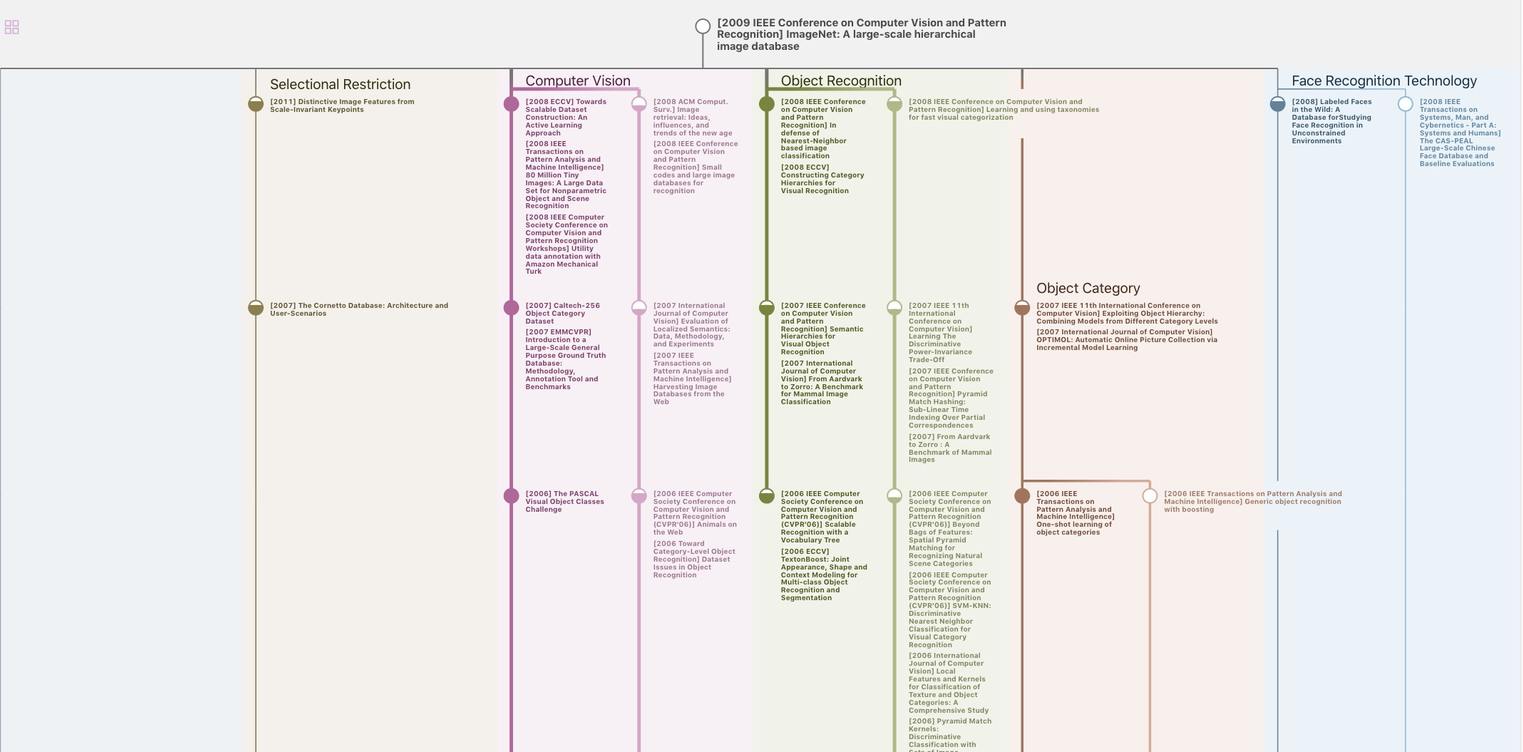
生成溯源树,研究论文发展脉络
Chat Paper
正在生成论文摘要