Stacked Broad Learning System Empowered FCL Assisted by DTN for Intrusion Detection in UAV Networks
IEEE CONFERENCE ON GLOBAL COMMUNICATIONS, GLOBECOM(2023)
摘要
An efficient Intrusion Detection System (IDS) model is essential for the protection of Unmanned Aerial Vehicles (UAVs) networks against network intrusion. However, when designing IDS models using distributed data collected by UAVs, it is crucial to ensure the security and privacy of the data. Moreover, most IDS models only focus on one-time learning and lack continuous learning capabilities. To address this, we present a Federated Continuous Learning framework with a Stacked Broad Learning System (FCL-SBLS) that utilizes Digital Twin Network (DTN) to enable quick and continuous learning on new data. To enhance the efficiency and quality of the IDS model during training and aggregation, we adopt an asynchronous federated learning architecture. Additionally, we introduce a Deep Deterministic Policy Gradient (DDPG)-based UAV selection scheme assisted by DTN to aid in global IDS model aggregation. This approach ensures that the IDS model can effectively and efficiently learn from distributed data while preserving the privacy and security of the data.The presented algorithm is validated using the CIC-IDS2017 dataset, and the simulation results reveal that our algorithm achieves higher efficiency and accuracy than the existing FL scheme.
更多查看译文
关键词
UAV network,intrusion detection system,federated continuous learning,stacked broad learning system,digital twin network
AI 理解论文
溯源树
样例
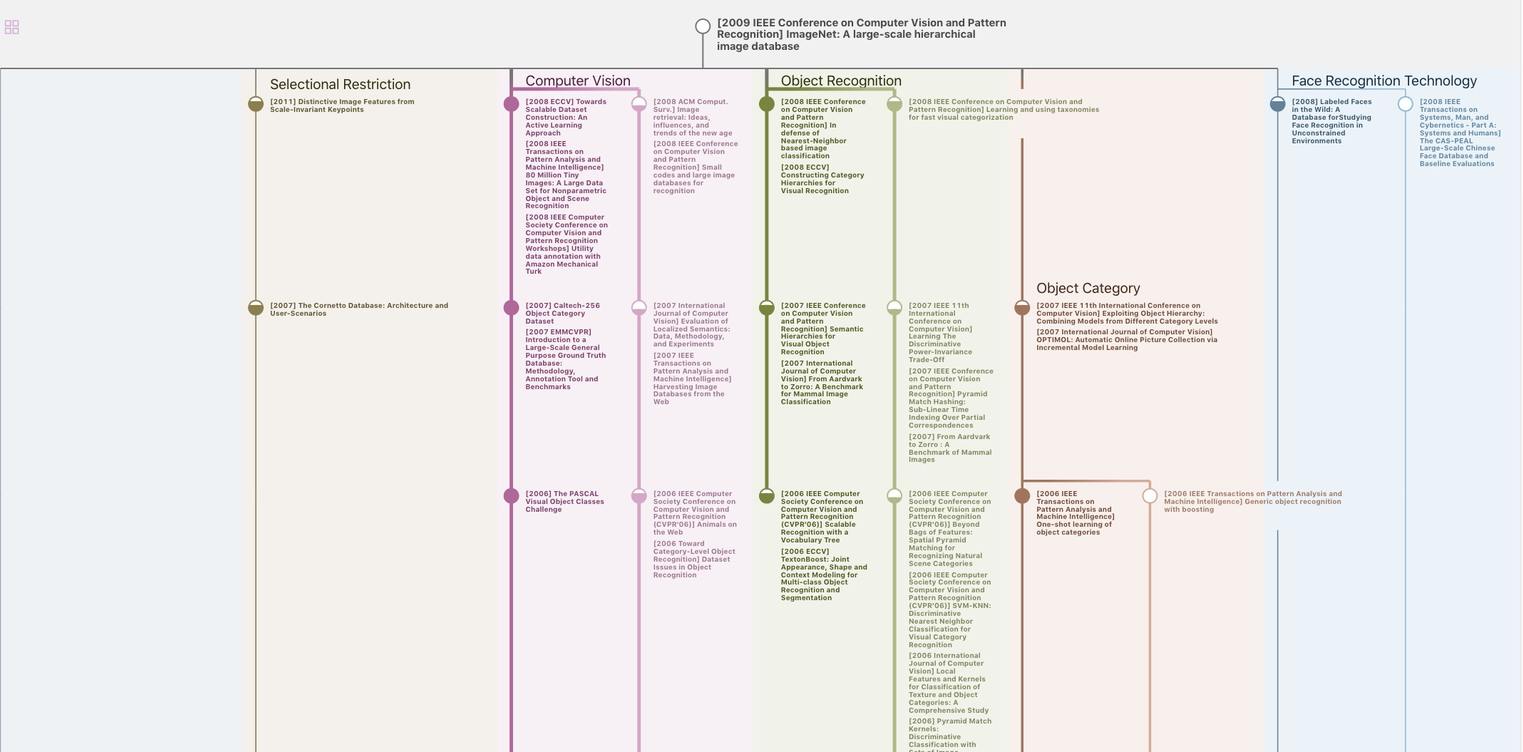
生成溯源树,研究论文发展脉络
Chat Paper
正在生成论文摘要