SetTron: Towards Better Generalisation in Penetration Testing with Reinforcement Learning
IEEE CONFERENCE ON GLOBAL COMMUNICATIONS, GLOBECOM(2023)
Abstract
Intelligent penetration testing (pen-testing), utilising Deep Reinforcement Learning (DRL) has gained attention due to its potential for improving testing efficiency and cost-effectiveness in evaluating network system security. Nonetheless, current approaches which rely on simplistic neural network architectures suffer limitations in transferability and their ability to generalise to new tasks, thus impeding their practical application. This paper aims to address these issues by formalising the pen-testing decision process as a Host-Centric Markov decision process (HC-MDP), as well as establishing a structural representation of the relationships among the hosts within a network system. Further, we propose a flexible policy architecture, the "SetTron", that leverages this structural representation to augment architectural inductive bias in a DRL agent and then practically evaluate our approach on pen-testing simulator platforms. The findings show SetTron to demonstrate superior performance, in terms of learning efficiency and policy convergence, compared to state-of-the-art methods and baselines with shorter penetration sequences and enhanced rewards. Besides, SetTron exhibits remarkable zero-shot generalisation capabilities, enabling perfect transfer to new tasks with randomly placed target hosts, achieving a 100% success rate, and outperforming baselines by a factor of 6 when comparing normalised scores.
MoreTranslated text
Key words
Network Security,Penetration Testing,Deep Reinforcement Learning,Generalisation,Inductive Bias
AI Read Science
Must-Reading Tree
Example
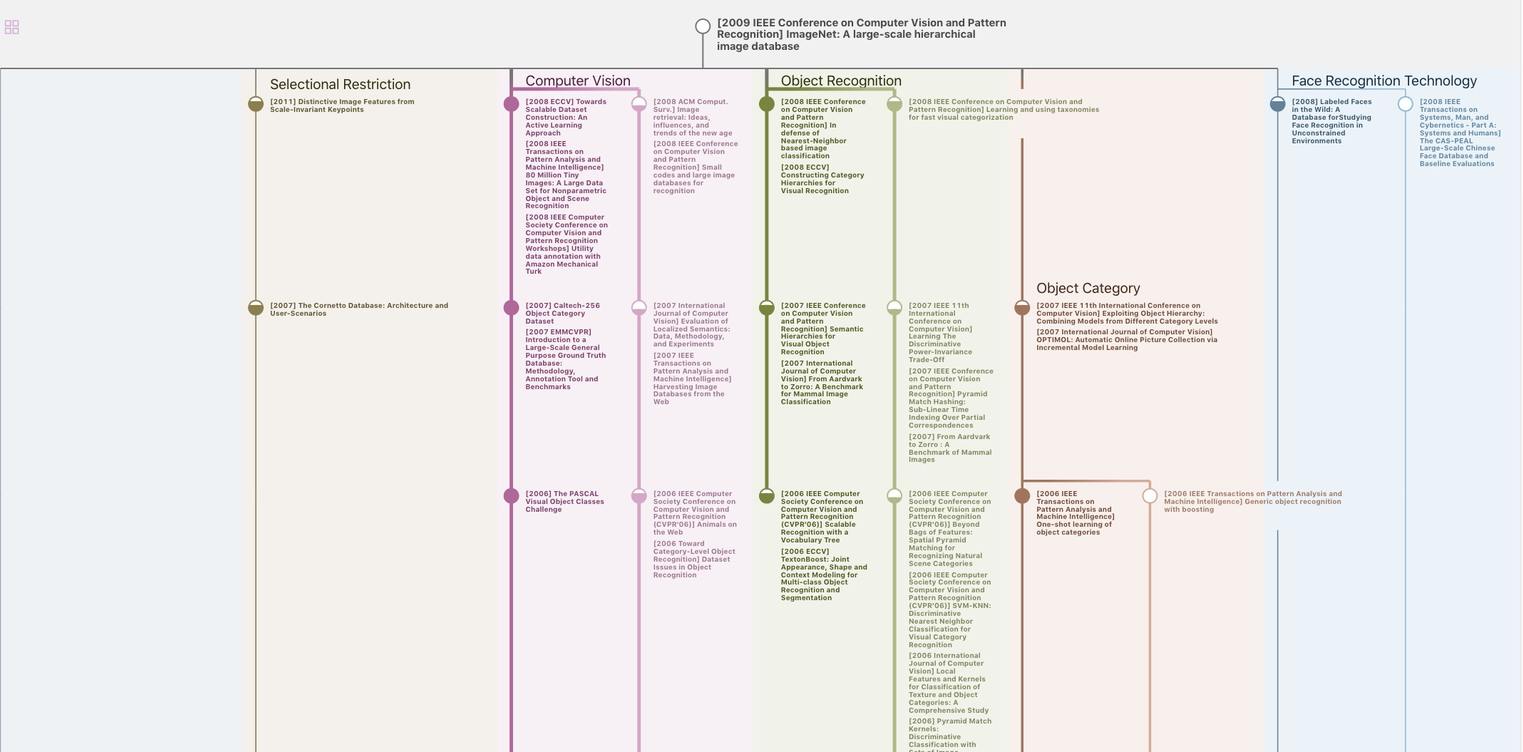
Generate MRT to find the research sequence of this paper
Chat Paper
Summary is being generated by the instructions you defined