Towards Differentially Private Over-the-Air Federated Learning via Device Sampling
IEEE CONFERENCE ON GLOBAL COMMUNICATIONS, GLOBECOM(2023)
摘要
Recent years have witnessed the development of federated learning (FL) that allows wireless devices (WDs) to collaboratively learn a global model under the coordination of a parameter server without sharing local datasets. To meet the communication efficiency and privacy requirements, over-the-air computation and differential privacy (DP) are further incorporated in FL by leveraging the signal-superposition property of multiple-access channels, as well as artificial noises to perturb local model updates for DP preservation. In this paper, we consider the device sampling with replacement, as an amplifier for the DP levels of WDs, in differentially private over-the-air FL. Accordingly, we study the joint optimization of device sampling strategy and over-the-air transceiver design that maximizes the learning performance while satisfying the DP requirement of each WD. The problem is challenging due to the intractable FL convergence rate and privacy losses under the sampling randomness, and the strong coupling among mixed decision variables. To tackle this problem, we first derive the analytical learning convergence rate and privacy losses of WDs, based on which the optimal transceiver design and device sampling strategy are obtained in closed forms. Numerical results demonstrate the effectiveness of our proposed approach compared with representative baselines.
更多查看译文
关键词
Differential privacy,federated learning,over-the-air computation,device sampling
AI 理解论文
溯源树
样例
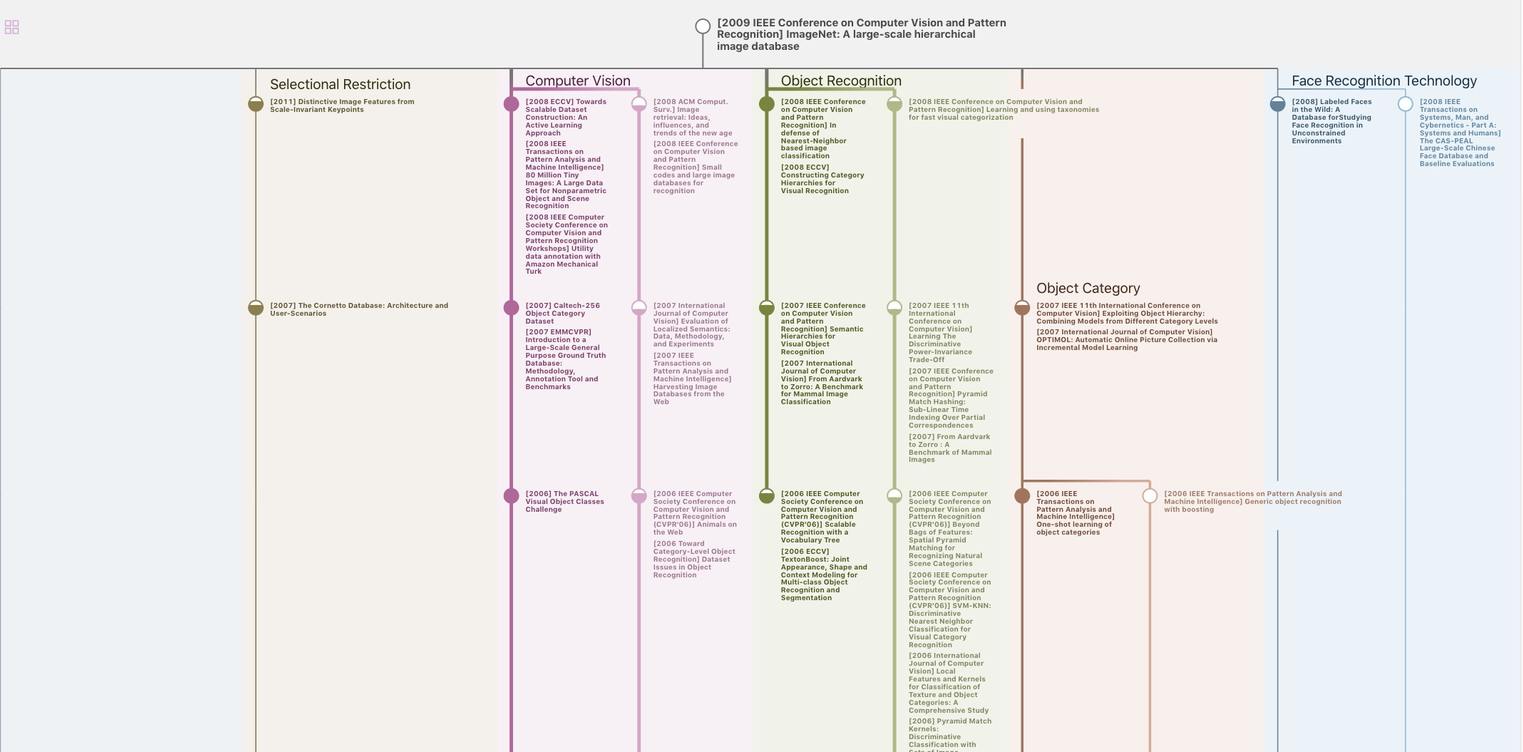
生成溯源树,研究论文发展脉络
Chat Paper
正在生成论文摘要