On the Privacy Leakage of Over-the-Air Federated Learning Over MIMO Fading Channels.
Global Communications Conference(2023)
摘要
Federated learning (FL) allows edge devices to collaboratively train machine learning models without directly sharing data. While over-the-air model aggregation improves communication efficiency, model uploading can lead to privacy risks. Previous research has focused on over-the-air FL with a single-antenna server, leveraging communication noise to enhance user-level privacy. This method achieves the so-called “free” privacy by decreasing transmit power instead of introducing additional privacy-preserving mechanisms at the devices. In this paper, we analyze the privacy leakage of over-the-air FL over a multiple-input multiple-output (MIMO) fading channel. We show that FL model aggregation with a multiple-antenna server amplifies privacy leakage. Consequently, relying solely on communication noise is inefficient to meet high privacy requirements, particularly when the receive antenna array is large. This calls for a joint optimization algorithm for the device-side privacy-preserving mechanism and the receiving protocol to achieve a better privacy-learning tradeoff. Numerical results validate our analysis and highlight the impact of the transmit power and the receive antenna array size on the privacy leakage.
更多查看译文
关键词
Multiple-input Multiple-output,Fading Channel,Federated Learning,Privacy Leakage,Machine Learning,Model Aggregation,Privacy Requirements,Federated Learning Model,Gaussian Noise,Information Extraction,Data Privacy,Additive Noise,Time Slot,Beamforming,Training Iterations,Local Gradient,Wireless Devices,Minimum Mean Square Error,Local Dataset,Threat Model,Artificial Noise,Loss Of Privacy,Edge Server,Differential Privacy,Multiple-input Multiple-output Channel,Transceiver Design,Normalized Mean Square Error,Training Round,Local Training,Signal Vector
AI 理解论文
溯源树
样例
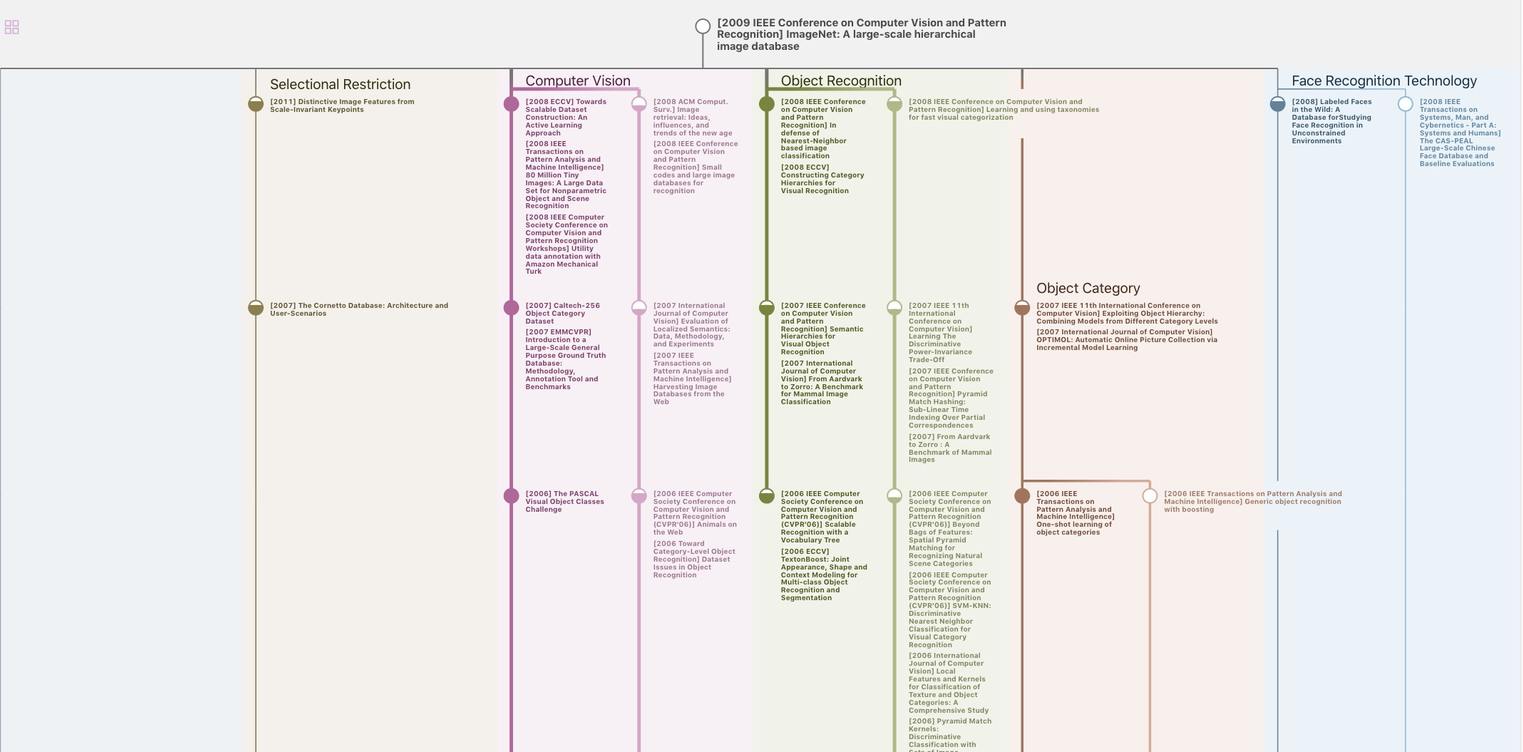
生成溯源树,研究论文发展脉络
Chat Paper
正在生成论文摘要