Ribonanza: deep learning of RNA structure through dual crowdsourcing.
bioRxiv : the preprint server for biology(2024)
摘要
Prediction of RNA structure from sequence remains an unsolved problem, and progress has been slowed by a paucity of experimental data. Here, we present Ribonanza, a dataset of chemical mapping measurements on two million diverse RNA sequences collected through Eterna and other crowdsourced initiatives. Ribonanza measurements enabled solicitation, training, and prospective evaluation of diverse deep neural networks through a Kaggle challenge, followed by distillation into a single, self-contained model called RibonanzaNet. When fine tuned on auxiliary datasets, RibonanzaNet achieves state-of-the-art performance in modeling experimental sequence dropout, RNA hydrolytic degradation, and RNA secondary structure, with implications for modeling RNA tertiary structure.
更多查看译文
AI 理解论文
溯源树
样例
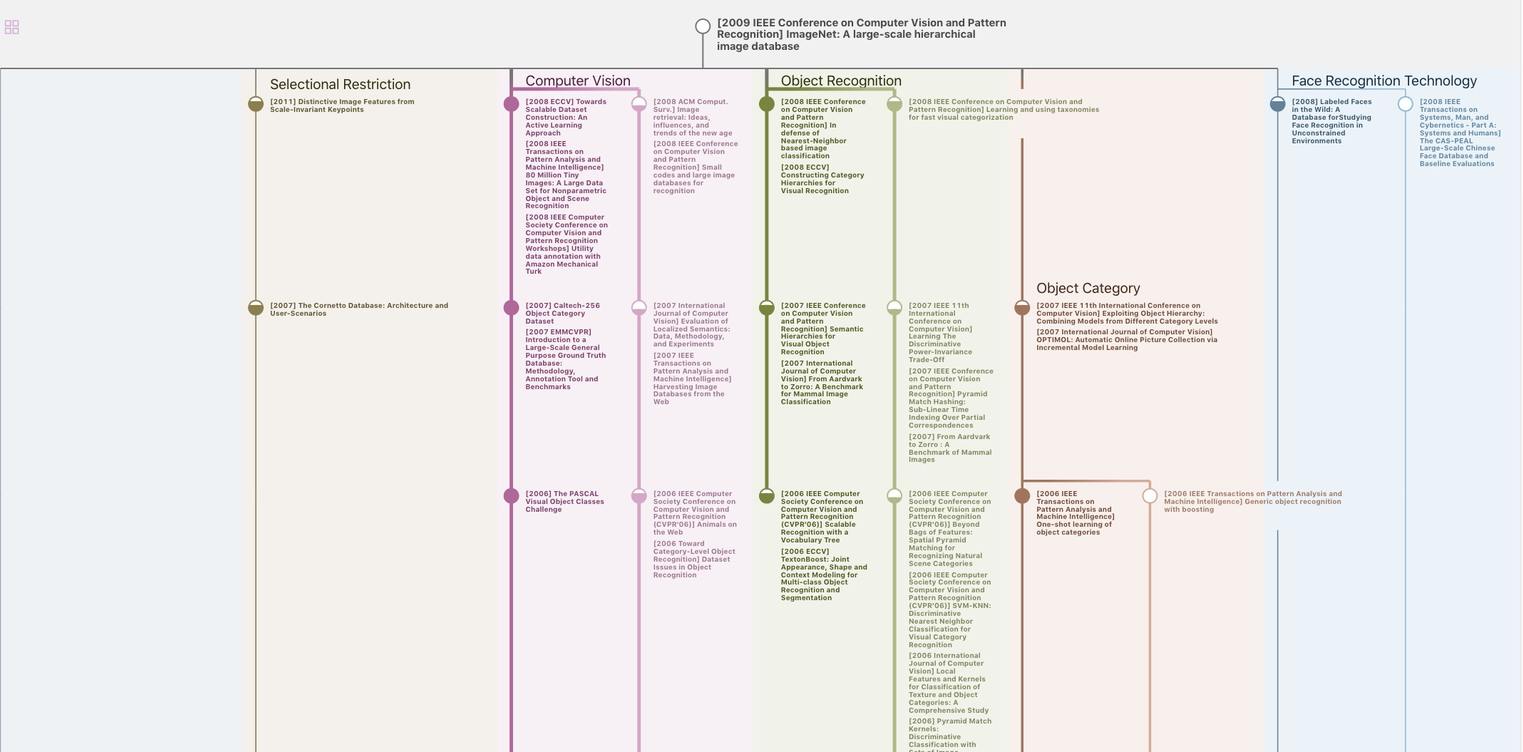
生成溯源树,研究论文发展脉络
Chat Paper
正在生成论文摘要