Light Weight AI: Representing ML Inference as Efficient Mathematical Relations for Embedded RAN Devices
IEEE CONFERENCE ON GLOBAL COMMUNICATIONS, GLOBECOM(2023)
摘要
Wireless 5G and beyond (B5G) technology offers multiple of machine learning (ML) use cases, including congestion detection, handover prediction, MAC scheduling and more. Many of these use cases involve solving complex problems that need neural networks (NN) or classical ML algorithms to achieve optimal solutions. However, implementing these NN inferences on resource-constraint base stations (BS) pose significant challenges. BSs has several limitations in terms of CPU frequency, number of cores, memory capacity, and the absence of dedicated ML hardware (HW) offloads. In this paper, the authors, propose a lightweight artificial intelligence (LWAI) method to derive computationally efficient mathematical relations (EMR) between Key Performance Indicators (KPIs) using reinforcement learning (RL). The derived EMR enables ML inference to be implemented on resource-constrained BSs. LWAI takes KPIs information as input and provides EMRs as output. The generated EMRs are then deployed on the BS. Inference is made to the BS using these relations. This approach makes ML inference realizable on embedded BSs with commercial-grade accuracy and optimal real-time prediction latency. We demonstrate the effectiveness and applicability of the LWAI framework in two real-world scenarios. The results highlight the potential for our approach to detect congestion by predicting physical resource block (PRB) and energy savings in BS. Our results show the efficacy of EMRs. LWAI framework-derived EMRs consume around 10% CPU cycles and 5% memory to execute as compared to NN models while maintaining over 90% prediction accuracy. Our approach opens up new possibilities for realizing ML inference ideas on resource-constraint embedded devices.
更多查看译文
关键词
Reinforcement learning,machine learning,wireless communication,resource optimization
AI 理解论文
溯源树
样例
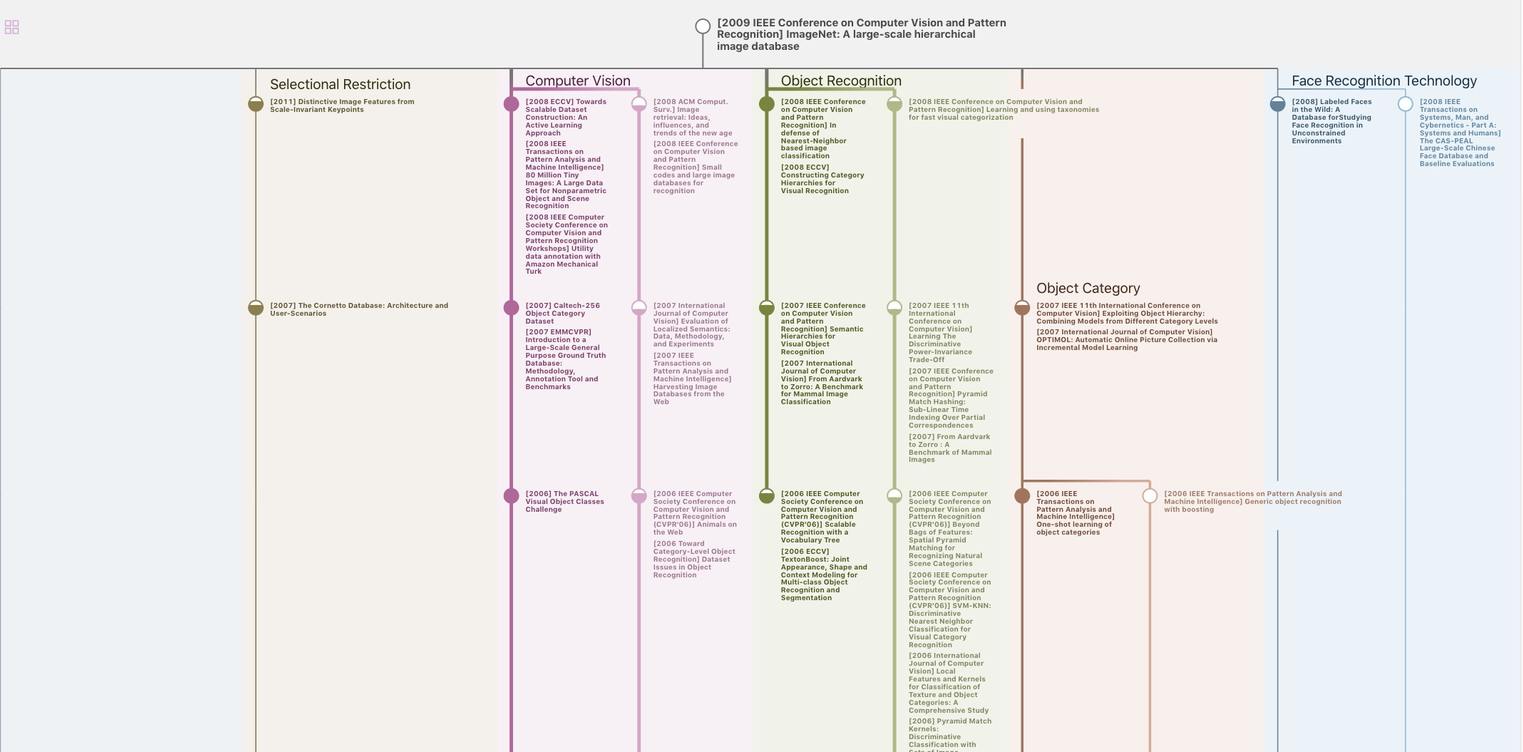
生成溯源树,研究论文发展脉络
Chat Paper
正在生成论文摘要