QoS-aware Reinforcement Learning based Green Trajectory Design for UAV-aided Backscattering
IEEE CONFERENCE ON GLOBAL COMMUNICATIONS, GLOBECOM(2023)
Abstract
Backscatter communication (BackCom) has been gaining a lot of interest as a low-energy consumption energy harvesting solution. Here, the limited transmission range of BackCom systems constraint can now be resolved by using mobile data collectors or readers such as unmanned aerial vehicles (UAVs). In this study, we investigate a monostatic UAV-assisted BackCom system where backscatter devices (BDs) are served in a time-division multiple access (TDMA) fashion. Here we solve an energy efficiency (EE) maximization problem by jointly optimizing the transmit power allocation and the UAV's trajectory while adhering to the quality of service constraints. Since the problem is non-convex and combinatorial in nature, we employ a reinforcement learning framework that utilizes a finite-state Markov decision process. We introduce a low-complexity Expected State-Action-Reward-State-Action (ESARSA) algorithm to determine the UAV's optimal trajectory with power allocation. A closed-form solution is proposed for global power optimization. In simulations, we compare the implemented ESARSA against the State-Action-Reward-State-Action (SARSA) and Q-learning algorithms and show that the proposed ESARSA algorithm can provide a 24% gain over the fixed allocation benchmark.
MoreTranslated text
Key words
Trajectory Design,Service Quality,Global Optimization,Great Power,Unmanned Aerial Vehicles,State Machine,Joint Optimization,Markov Decision Process,Trajectory Optimization,Reinforcement Learning Framework,Time Division Multiple Access,Q-learning Algorithm,Unmanned Aerial Vehicle Trajectory,Quality Of Service Constraints,Transmit Power Allocation,Optimization Problem,Data Rate,Internet Of Things,Time Slot,Path Loss,Data Rate Requirements,Discrete Action,Reinforcement Learning Algorithm,Greedy Policy,Global Optimal Solution,Channel Gain,Access Protocol,Optimal Power Allocation,Benchmark Algorithms,Improve Energy Efficiency
AI Read Science
Must-Reading Tree
Example
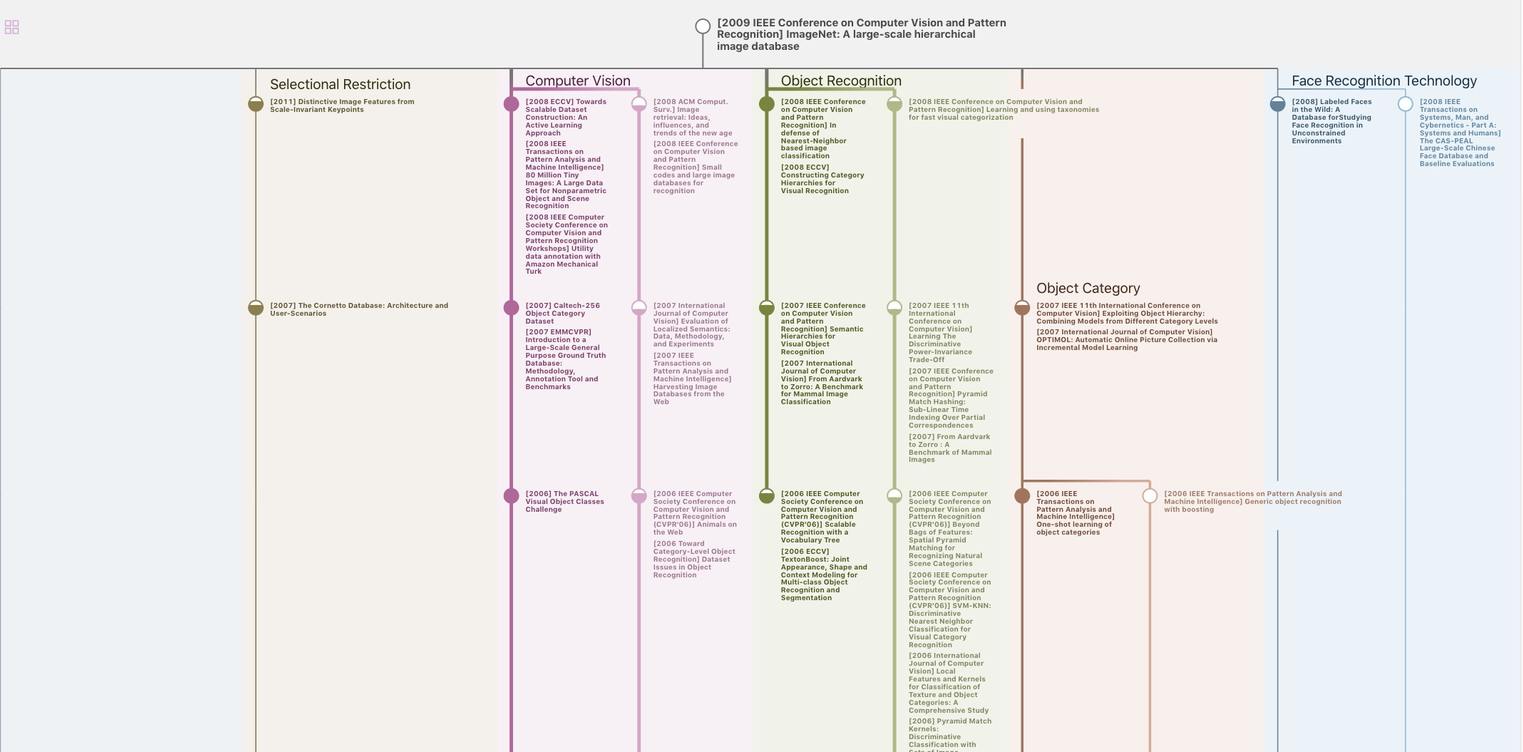
Generate MRT to find the research sequence of this paper
Chat Paper
Summary is being generated by the instructions you defined