Social-Inspired Multicast Feature Selections with Mobile Edge Computing.
Global Communications Conference(2023)
摘要
The emergence of AI has shifted the focus of wireless communications towards deep semantic-level fidelity (i.e., semantic communication networks), emphasizing the significance and effectiveness of transmitted data. However, semantic feature selection considering multicast users with social relations for feature sharing has not been explored. In this paper, we formulate a new optimization problem to minimize the total communication, forwarding, and computation costs, with the proof of NP-hardness and inapproximability. We propose a new algorithm, Multicast Semantic Feature Selection (MSFS), with the notions of Cross Task Semantic Indicator, Substituted Subgraph, and Socially Feature Selection Indicator, to select features on different mobile edge computing servers and cluster the users to receive features via multicast. Simulations with real datasets manifest that MSFS can reduce the total cost by more than 50% compared with state-of-the-art algorithms.
更多查看译文
关键词
Mobile Edge Computing,Social Relationships,Computational Cost,Communication Cost,Total Computation,Total Communication,Total Computational Cost,Similar Characteristics,Data Rate,Additive Noise,Multiple Tasks,Shared Features,Path Loss,Task Group,Task Characteristics,Computational Capabilities,Redundant Features,Reconstruction Loss,Communication Overhead,Graph Features,Co-occurrence Features,Air Quality Index,Social Graph,User Clustering,Regularization Loss,Inexpressible,Feature Selection Problem,Channel State,Performance Gap,Higher Total Costs
AI 理解论文
溯源树
样例
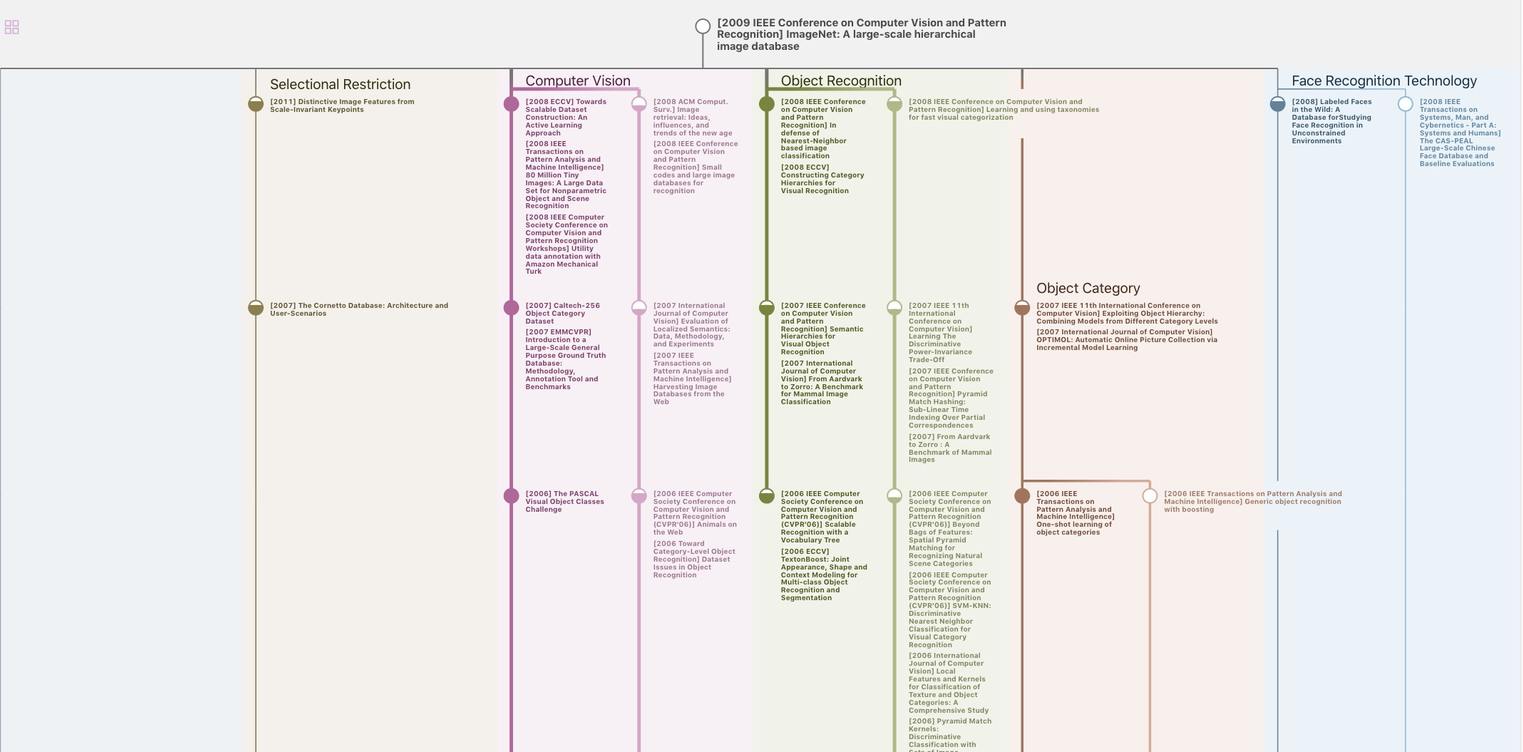
生成溯源树,研究论文发展脉络
Chat Paper
正在生成论文摘要