Energy-Efficient Dynamic Device Scheduling for Over-the-Air Federated Learning in UAV Swarms.
Global Communications Conference(2023)
摘要
Recent years have envisioned the widespread adoption of machine learning (ML) in unmanned aerial vehicle (UAV) swarms for task execution. However, it is hard for the traditional centralized ML approaches to be applied in UAV swarms due to the large latency, communication cost, and privacy disclosure when transmitting the raw data. As an alternative, over-the-air computation (AirComp)-enabled federated learning (FL) is expected as a communication-efficient solution by harnessing the interference. However, the benefit of AirComp is at the cost of compromised training performance due to the channel distortion caused by the fading channel and noise and straggler issues resulting from the aligned parameters. In addition, the limited energy budget and dynamic environment incorporating the mobility characteristic of UAVs and time-varying channel conditions make these issues more complex. To solve problems aforementioned, this work proposes an energy-and-communication-efficient device scheduling scheme for AirComp-enabled FL system in the UAV swarms. Specifically, we first derive the optimality gap to characterize the impact of channel distortion and device selection on training performance. Then based on this result, we formulate an optimization problem to minimize the optimality gap by scheduling an appropriate number of competent following UAVs in each round, considering the transmission and computation energy consumption as well. Simulation results validate that the proposed scheme can achieve superior training performance in terms of test accuracy compared with baselines, and shows robustness with the increasing scale of UAV swarm.
更多查看译文
关键词
Unmanned Aerial Vehicles,Federated Learning,Energy-efficient Devices,Unmanned Aerial Vehicle Swarm,Device Scheduling,Energy Consumption,Test Accuracy,Channel State,Training Performance,Fading Channel,Communication Cost,Impact Of Selection,Scheduling Scheme,Device Selection,Computation Energy,Transmission Energy Consumption,Computation Energy Consumption,Learning Rate,Global Model,Air Quality,Communication Rounds,Channel Gain,Random Noise,Local Training,Federated Learning Framework,Single Round,Local Updates,Target Recognition,Line-of-sight,Path Loss
AI 理解论文
溯源树
样例
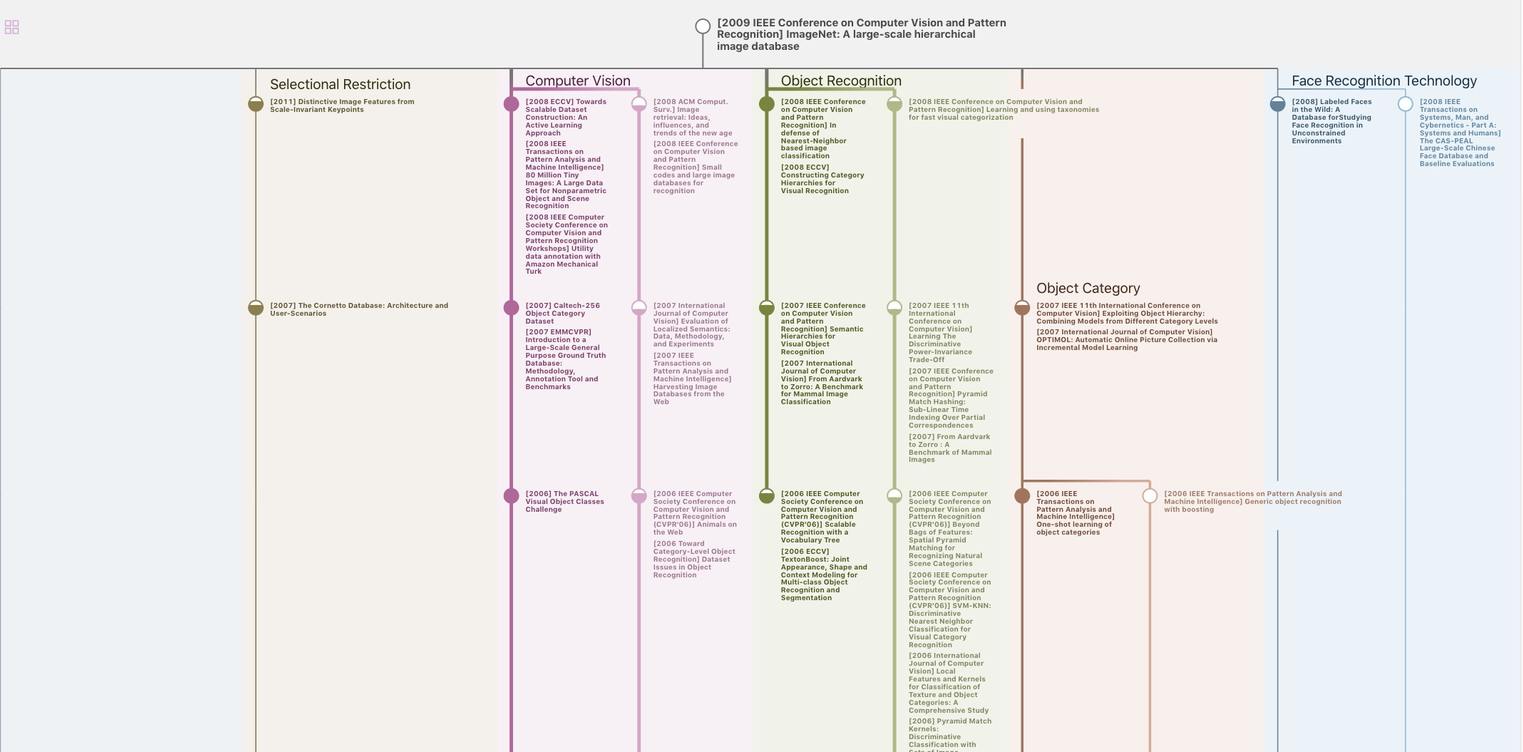
生成溯源树,研究论文发展脉络
Chat Paper
正在生成论文摘要