FedFaSt: Selective Federated Learning using Fittest Parameters Aggregation and Slotted Clients Training
IEEE CONFERENCE ON GLOBAL COMMUNICATIONS, GLOBECOM(2023)
Abstract
This paper proposes a novel selective federated learning (FL) algorithm, called fittest aggregation and slotted training (FedFaSt). It relies on a "free-for-all" client training process to score clients' efficiency while applying the "natural selection" principle to elect the fittest clients to be used in FL training and aggregation processes. While relying on a combined data quality and training performance metric for scoring clients, FedFaSt implements a slotted training model enabling teams of fittest clients to participate in the training and aggregation processes for a fixed number of successive rounds, called slots. Performance validation using X-ray datasets reveals that FedFaSt outperforms selective federated learning algorithms like FedAVG, FedRand, and FedPow in terms of accuracy, convergence to the global optimum, time complexity, and robustness against attacks.
MoreTranslated text
Key words
Federated Learning,Selective Federated Learning,FedFaSt,FedAVG,FedPow,X-Ray Dataset,AI for Health
AI Read Science
Must-Reading Tree
Example
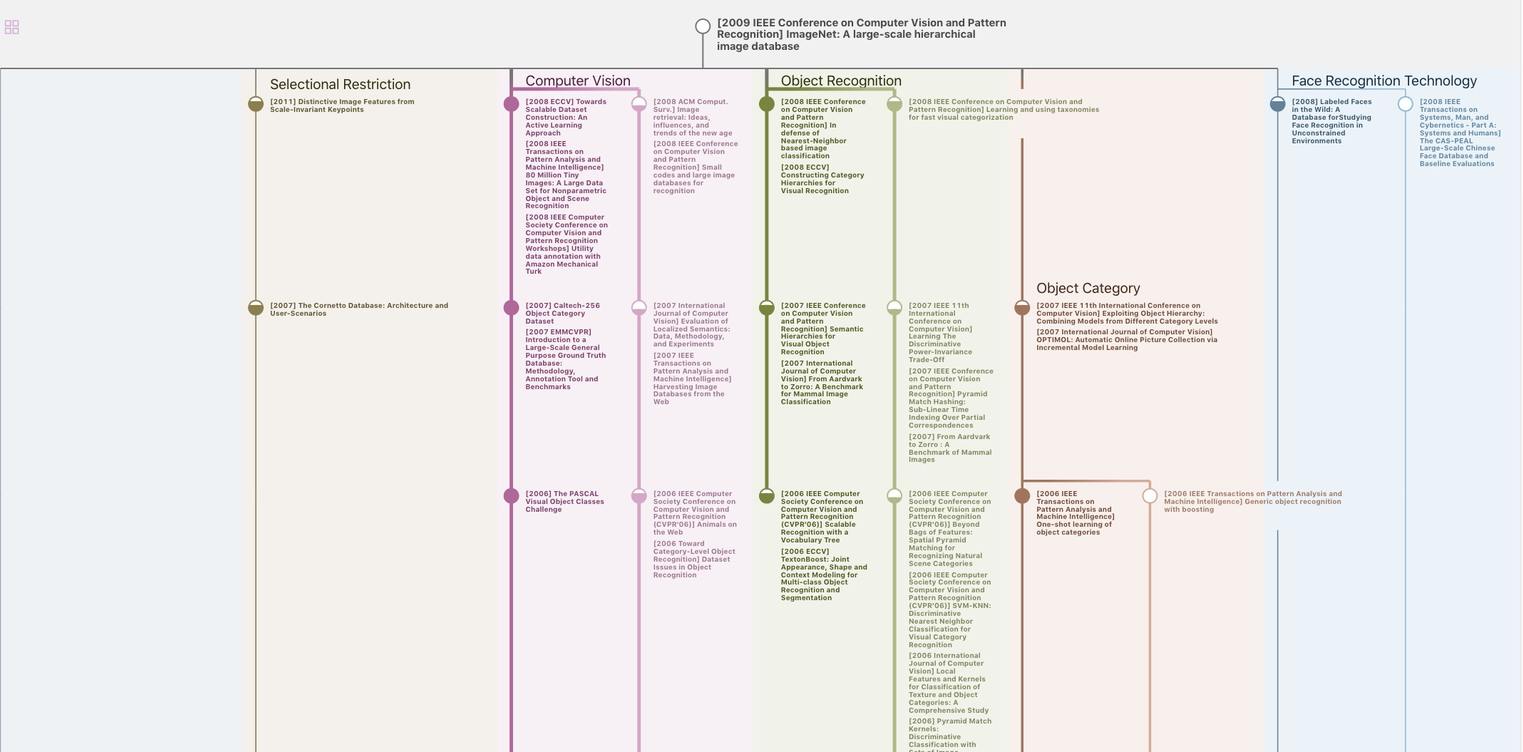
Generate MRT to find the research sequence of this paper
Chat Paper
Summary is being generated by the instructions you defined