LS-YOLO: A Novel Model for Detecting Multiscale Landslides With Remote Sensing Images
IEEE JOURNAL OF SELECTED TOPICS IN APPLIED EARTH OBSERVATIONS AND REMOTE SENSING(2024)
摘要
The landslide is a widespread and devastating natural disaster, posing serious threats to human life, security, and natural assets. Investigating efficient methods for accurate landslide detection with remote sensing images has important academic and practical implications. In this article, we proposed an LS-YOLO, a novel and effective model for landslide detection with remote sensing images. We first built a multiscale landslide dataset (MSLD) and introduced random seeds in the data augmentation to increase data robustness. Considering the multiscale characteristic of landslides in remote sensing images, a multiscale feature extraction module is designed based on efficient channel attention, average pooling, and spatial separable convolution. To increase the receptive field of the model, dilated convolution is employed to the decoupled head. Specifically, the context enhancement module consisting of dilation convolutions is added to the decoupled head regression task branch, and then the improved decoupled head is to replace the coupled head in YOLOv5s. Extensive experiments show that our proposed model has high performance for multiscale landslide detection and outperforms other object detection models (faster RCNN, SSD, EfficientDet-D0, YOLOv5s, YOLOv7, and YOLOX). Compared with the baseline model YOLOv5s, the AP of the LS-YOLO for detecting landslides has increased by 2.18%-97.06%.
更多查看译文
关键词
Landslides,Remote sensing,YOLO,Feature extraction,Convolution,Head,Disasters,Deep learning,dilated convolution,landslide detection,remote sensing images,spatial separable convolution,YOLOv5s
AI 理解论文
溯源树
样例
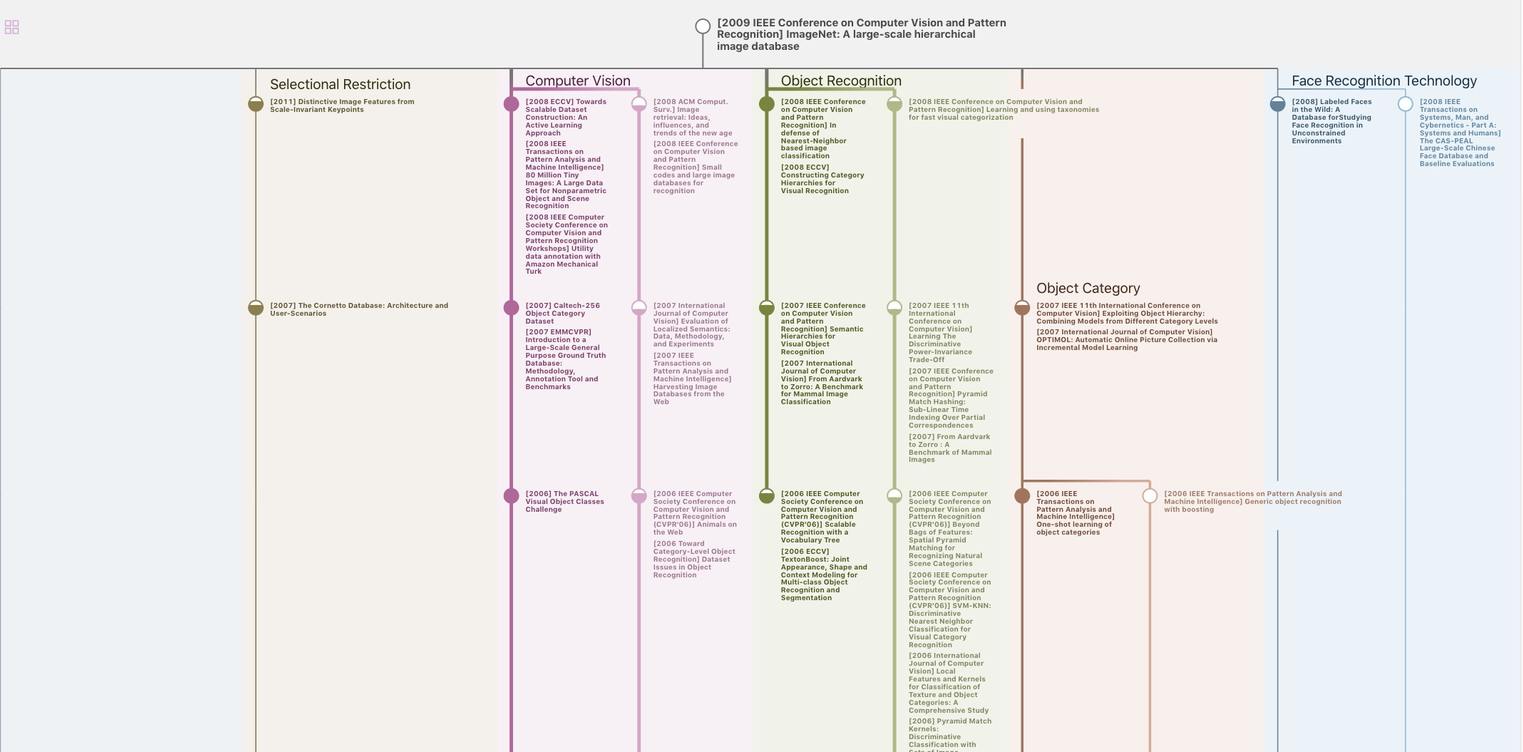
生成溯源树,研究论文发展脉络
Chat Paper
正在生成论文摘要