Improved Clustering Using Nice Initialization
IEEE CONFERENCE ON GLOBAL COMMUNICATIONS, GLOBECOM(2023)
摘要
Popular clustering methods, such as the k-means and the Gaussian mixture model (GMM), are composed of an initialization stage and an iteration stage. Although this is well known, the role of the two stages has not been well studied for existing clustering methods yet. To understand this issue, the current research investigates well-known existing k-means and the GMM methods. The finding is that the initialization stage is more critical than the iteration stage. Based on this issue, the research develops improved k-means and GMM methods by using a nice initialization, which significantly enhances the performance of the corresponding methods. Experiments based on Monte Carlo simulations show that the proposed improved k-means and GMM methods can completely identify the true clusters if they are well separated from each, but this cannot be achieved by the existing k-means and GMM methods. Experiments based on a real-world dataset show that the proposed methods can be efficiently combined with dimension-reduction techniques for clustering high-dimensional massive data.
更多查看译文
关键词
adjusted rand index,EM-algorithm,Gaussian mixture model,k-means
AI 理解论文
溯源树
样例
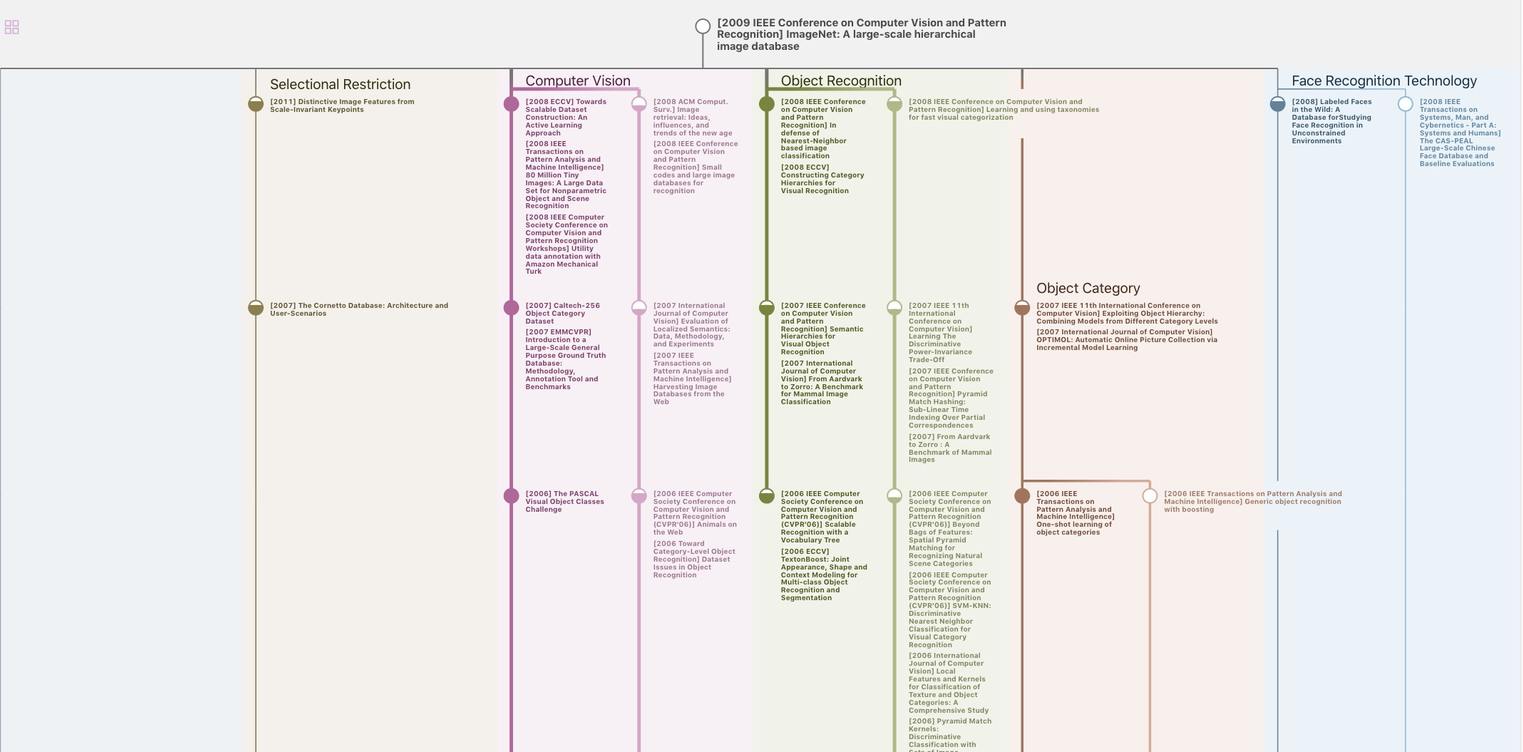
生成溯源树,研究论文发展脉络
Chat Paper
正在生成论文摘要