Sparse Variational Contaminated Noise Gaussian Process Regression for Forecasting Geomagnetic Perturbations
CoRR(2024)
摘要
Gaussian Processes (GP) have become popular machine learning methods for
kernel based learning on datasets with complicated covariance structures. In
this paper, we present a novel extension to the GP framework using a
contaminated normal likelihood function to better account for heteroscedastic
variance and outlier noise. We propose a scalable inference algorithm based on
the Sparse Variational Gaussian Process (SVGP) method for fitting sparse
Gaussian process regression models with contaminated normal noise on large
datasets. We examine an application to geomagnetic ground perturbations, where
the state-of-art prediction model is based on neural networks. We show that our
approach yields shorter predictions intervals for similar coverage and accuracy
when compared to an artificial dense neural network baseline.
更多查看译文
AI 理解论文
溯源树
样例
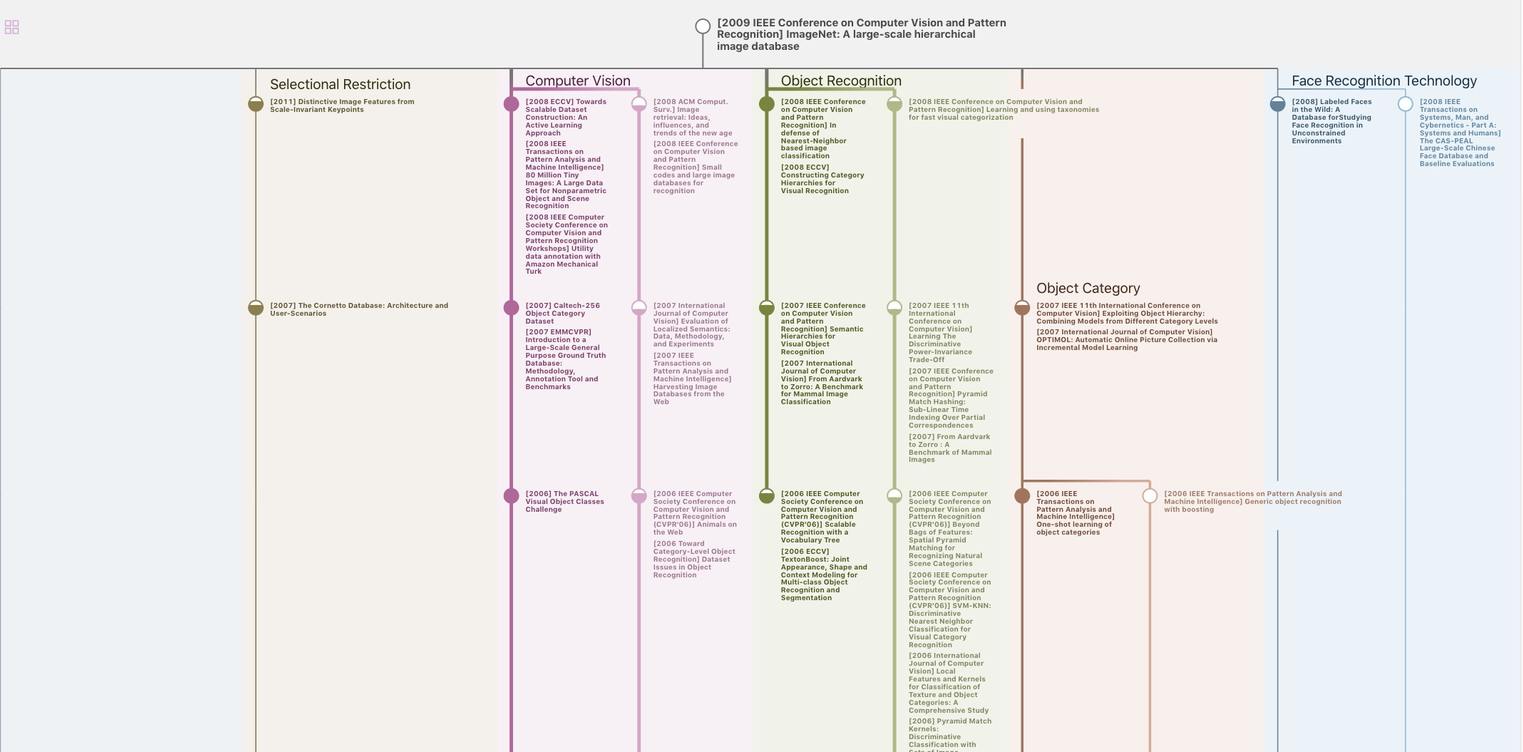
生成溯源树,研究论文发展脉络
Chat Paper
正在生成论文摘要