Predicting postoperative risks using large language models
arxiv(2024)
Abstract
Predicting postoperative risk can inform effective care management
planning. We explored large language models (LLMs) in predicting postoperative
risk through clinical texts using various tuning strategies. Records spanning
84,875 patients from Barnes Jewish Hospital (BJH) between 2018 2021, with a
mean duration of follow-up based on the length of postoperative ICU stay less
than 7 days, were utilized. Methods were replicated on the MIMIC-III dataset.
Outcomes included 30-day mortality, pulmonary embolism (PE) pneumonia. Three
domain adaptation finetuning strategies were implemented for three LLMs
(BioGPT, ClinicalBERT BioClinicalBERT): self-supervised objectives;
incorporating labels with semi-supervised fine-tuning; foundational modelling
through multi-task learning. Model performance was compared using the AUROC
AUPRC for classification tasks MSE R2 for regression tasks. Cohort had a
mean age of 56.9 (sd: 16.8) years; 50.3
outperformed traditional word embeddings, with absolute maximal gains of 38.3
for AUROC 14
further improved performance by 3.2
labels into the finetuning procedure further boosted performances, with
semi-supervised finetuning improving by 1.8
foundational modelling improving by 3.6
self-supervised finetuning. Pre-trained clinical LLMs offer opportunities for
postoperative risk predictions with unseen data, further improvements from
finetuning suggests benefits in adapting pre-trained models to note-specific
perioperative use cases. Incorporating labels can further boost performance.
The superior performance of foundational models suggests the potential of
task-agnostic learning towards the generalizable LLMs in perioperative care.
MoreTranslated text
AI Read Science
Must-Reading Tree
Example
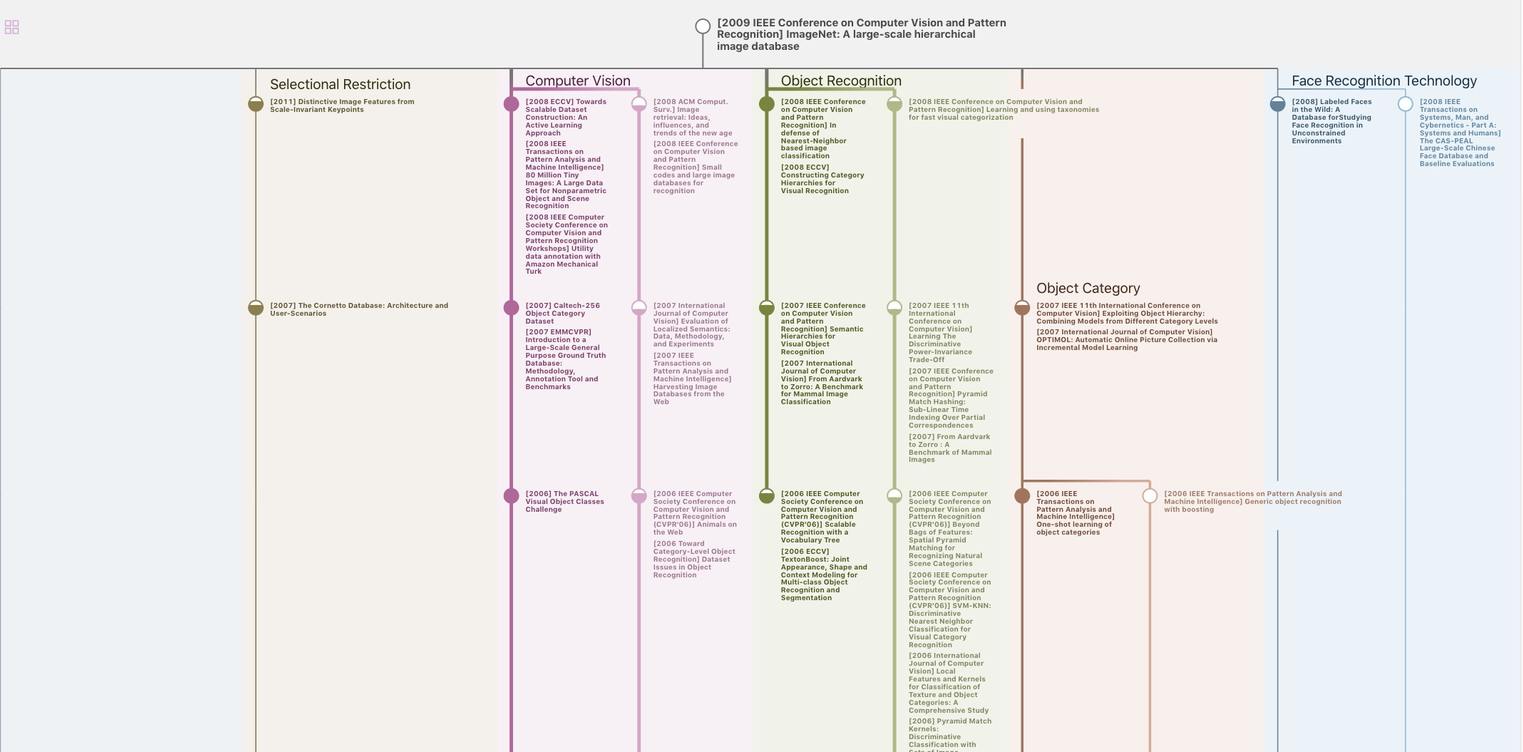
Generate MRT to find the research sequence of this paper
Chat Paper
Summary is being generated by the instructions you defined