The risks of risk assessment: causal blind spots when using prediction models for treatment decisions
arxiv(2024)
摘要
Prediction models are increasingly proposed for guiding treatment decisions,
but most fail to address the special role of treatments, leading to
inappropriate use. This paper highlights the limitations of using standard
prediction models for treatment decision support. We identify `causal blind
spots' in three common approaches to handling treatments in prediction
modelling: including treatment as a predictor, restricting data based on
treatment status and ignoring treatments. When predictions are used to inform
treatment decisions, confounders, colliders and mediators, as well as changes
in treatment protocols over time may lead to misinformed decision-making. We
illustrate potential harmful consequences in several medical applications. We
advocate for an extension of guidelines for development, reporting and
evaluation of prediction models to ensure that the intended use of the model is
matched to an appropriate risk estimand. When prediction models are intended to
inform treatment decisions, prediction models should specify upfront the
treatment decisions they aim to support and target a prediction estimand in
line with that goal. This requires a shift towards developing predictions under
the specific treatment options under consideration (`predictions under
interventions'). Predictions under interventions need causal reasoning and
inference techniques during development and validation. We argue that this will
improve the efficacy of prediction models in guiding treatment decisions and
prevent potential negative effects on patient outcomes.
更多查看译文
AI 理解论文
溯源树
样例
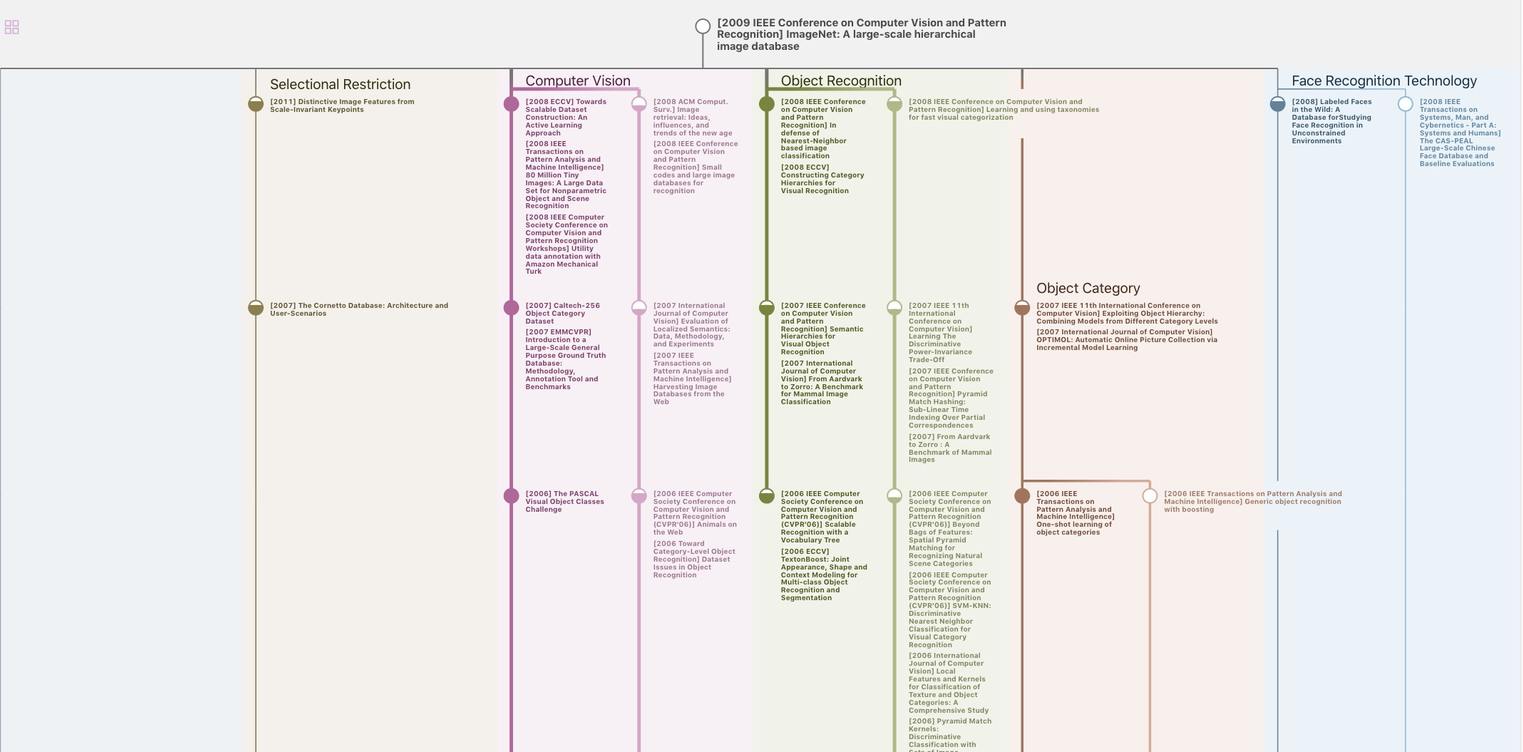
生成溯源树,研究论文发展脉络
Chat Paper
正在生成论文摘要