Scalable Community Search with Accuracy Guarantee on Attributed Graphs
CoRR(2024)
摘要
Given an attributed graph G and a query node q, Community
Search over Attributed Graphs (CS-AG) aims
to find a structure- and attribute-cohesive subgraph from G that contains
q. Although CS-AG has been widely studied, they still face three challenges.
(1) Exact methods based on graph traversal are time-consuming, especially for
large graphs. Some tailored indices can improve efficiency, but introduce
nonnegligible storage and maintenance overhead. (2) Approximate methods with a
loose approximation ratio only provide a coarse-grained evaluation of a
community's quality, rather than a reliable evaluation with an accuracy
guarantee in runtime. (3) Attribute cohesiveness metrics often ignores the
important correlation with the query node q. We formally define our CS-AG
problem atop a q-centric attribute cohesiveness metric considering both
textual and numerical attributes, for k-core model on homogeneous graphs. We
show the problem is NP-hard. To solve it, we first propose an exact baseline
with three pruning strategies. Then, we propose an index-free
sampling-estimation-based method to quickly return an approximate community
with an accuracy guarantee, in the form of a confidence interval. Once a good
result satisfying a user-desired error bound is reached, we terminate it early.
We extend it to heterogeneous graphs, k-truss model, and size-bounded CS.
Comprehensive experimental studies on ten real-world datasets show its
superiority, e.g., at least 1.54× (41.1× on average) faster in
response time and a reliable relative error (within a user-specific error
bound) of attribute cohesiveness is achieved.
更多查看译文
AI 理解论文
溯源树
样例
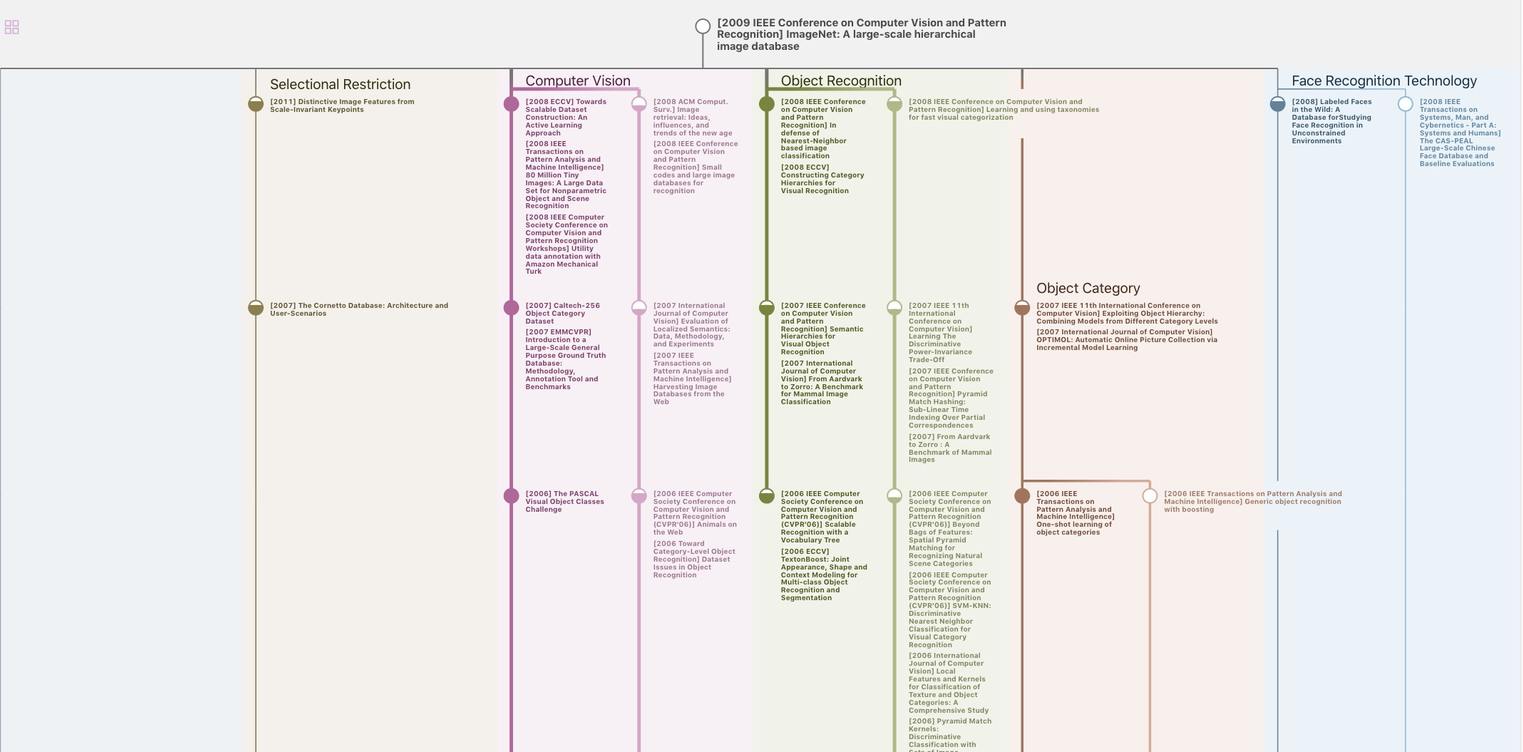
生成溯源树,研究论文发展脉络
Chat Paper
正在生成论文摘要