Unsupervised Zero-Shot Reinforcement Learning via Functional Reward Encodings
ICML 2024(2024)
Abstract
Can we pre-train a generalist agent from a large amount of unlabeled offline trajectories such that it can be immediately adapted to any new downstream tasks in a zero-shot manner? In this work, we present afunctionalreward encoding (FRE) as a general, scalable solution to thiszero-shot RLproblem. Our main idea is to learn functional representations of any arbitrary tasks by encoding their state-reward samples using a transformer-based variational auto-encoder. This functional encoding not only enables the pre-training of an agent from a wide diversity of general unsupervised reward functions, but also provides a way to solve any new downstream tasks in a zero-shot manner, given a small number of reward-annotated samples. We empirically show that FRE agents trained on diverse random unsupervised reward functions can generalize to solve novel tasks in a range of simulated robotic benchmarks, often outperforming previous zero-shot RL and offline RL methods.
MoreTranslated text
AI Read Science
Must-Reading Tree
Example
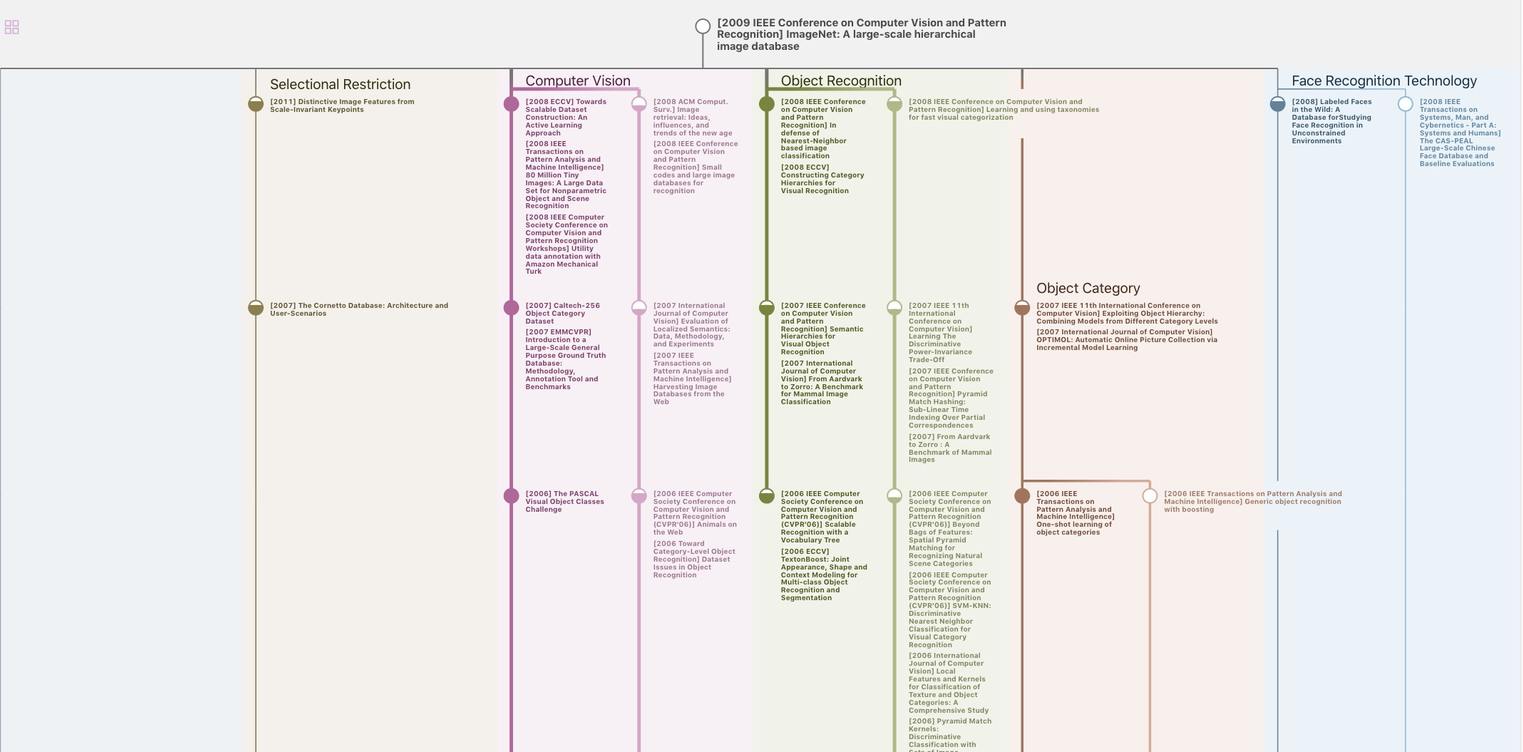
Generate MRT to find the research sequence of this paper
Chat Paper
Summary is being generated by the instructions you defined