Trajectory Design for 3D UAV Localization in UAV Based Networks
IEEE CONFERENCE ON GLOBAL COMMUNICATIONS, GLOBECOM(2023)
摘要
In this paper, the problem of using several controlled unmanned aerial vehicles (UAVs) to localize a target UAV in real time is investigated. In the considered model, the controlled UAV consists of one active UAV and four passive UAVs. Each passive UAV receives signals transmitted from the active UAV and reflected by the target UAV, and then estimates the distance from the active UAV to the target UAV and then from the target UAV to the passive UAV. Each passive UAV then transmits this distance information to a base station (BS), which estimates the location of the target UAV. Since the target UAV will change its location according to its performed task, each controlled UAV must optimize its trajectory to continuously localize the target UAV. This trajectory design problem is formulated as an optimization problem whose goal is to jointly optimize the trajectories of active and passive UAVs so as to maximize the target UAV positioning accuracy. To solve this problem, a Z function decomposition based reinforcement learning (ZD-RL) method is proposed. Compared to value function decomposition based RL (VD-RL), the proposed method can find the probability distribution of the sum of future rewards to accurately estimate the expected value of the sum of future rewards, thus finding better trajectories for controlled UAVs and improving target UAV positioning accuracy. Simulation results show that the proposed ZD-RL method can reduce the positioning errors by up to 58.3% and 84.8%, compared to VD-RL and independent DRL methods, respectively.
更多查看译文
关键词
Unmanned Aerial Vehicles,Trajectory Design,Optimization Problem,Value Function,Position Error,Reinforcement Learning Methods,Functional Decomposition,Sum Of Rewards,Neural Network,Signal-to-noise,Deep Neural Network,Distancing Measures,Random Method,Time Slot,Path Loss,3D Coordinates,Pitch Angle,Yaw Angle,Non-line-of-sight,Q-function,Deep Q-network,Deep Neural Network Parameters,Huber Loss,Ground Base Stations
AI 理解论文
溯源树
样例
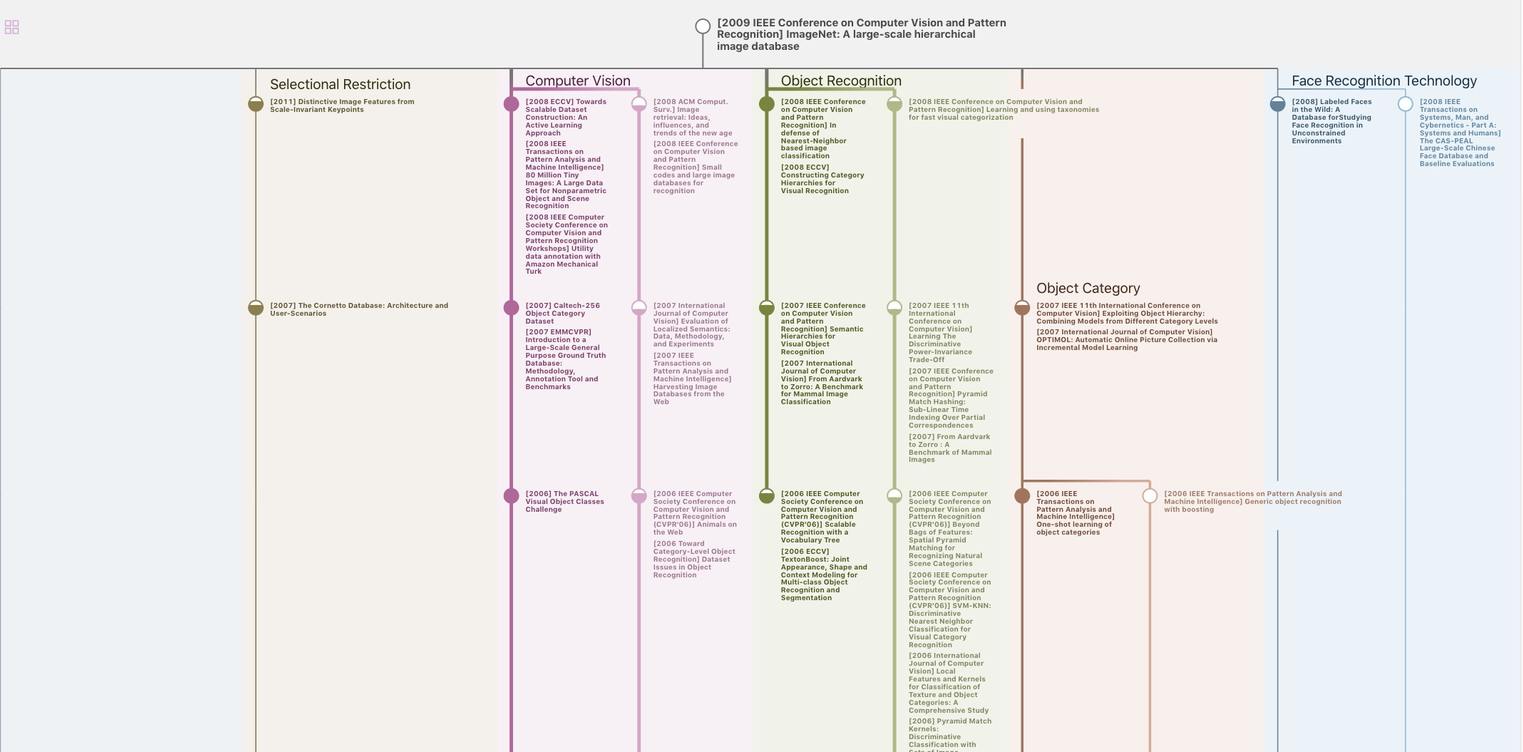
生成溯源树,研究论文发展脉络
Chat Paper
正在生成论文摘要