Efficient Federated Learning using Random Pruning in Resource-Constrained Edge Intelligence Networks
IEEE CONFERENCE ON GLOBAL COMMUNICATIONS, GLOBECOM(2023)
摘要
We study efficient federated learning (FL) using random pruning in resource-constrained edge intelligence networks. We propose an edge device selection strategy to identify appropriate edge devices for participating in FL at the beginning of each training iteration. We then formulate an optimization problem that jointly optimizes the pruning ratio, CPU frequency, uplink power, and bandwidth allocation for the selected edge devices. Since the optimization problem is non-convex and challenging to solve directly, we decompose it into three subproblems and propose efficient algorithms or closed-form solutions for each subproblem. Based on the solutions to the subproblems, an alternating optimization algorithm is constructed to solve the original problem. Simulation results demonstrate that our scheme outperforms baseline schemes in terms of both learning accuracy and energy consumption.
更多查看译文
关键词
Federated learning,random pruning,device selection,resource allocation
AI 理解论文
溯源树
样例
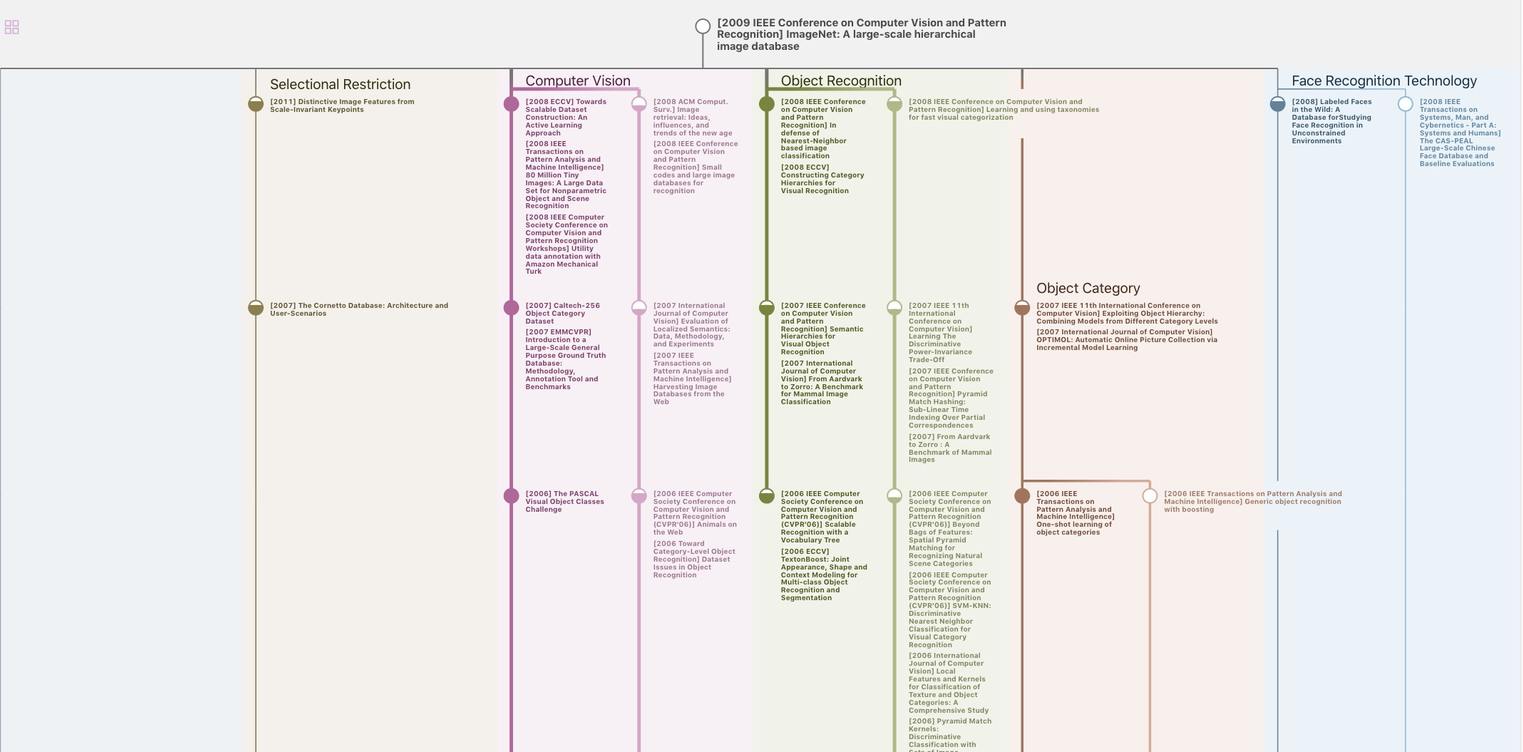
生成溯源树,研究论文发展脉络
Chat Paper
正在生成论文摘要