Resource-Constrained Specific Emitter Identification Using End-to-End Sparse Feature Selection
IEEE CONFERENCE ON GLOBAL COMMUNICATIONS, GLOBECOM(2023)
摘要
Specific emitter identification (SEI) refers to a process to determine the category of emitters by extracting, analyzing and matching the characteristics of received emitter signals. With the increasingly complex environment, traditional SEI methods, such as parameter matching, become difficult to meet the needs of robust and effective signal identification. Deep learning (DL) possesses powerful feature extraction ability and has been widely used in SEI. The superior performance of DL-based SEI methods also brings problems of redundant model parameters and high feature dimensionality, which further causes slow convergence rate, high storage requirements, and ever-increasing computational complexity. In this paper, we propose an SEI method based on end-to-end sparse feature selection (SFS) to make model pay more attention to features with good identification performance. Specifically, we add sparse parameters to features and design loss function composed of cross-entropy loss and sparse regularization. Several experiments are conducted on ADS-B, WiFi and LoRa datasets. From the simulation results, our proposed SFS-SEI method improves feature sparsity, speeds up loss convergence, reduces model parameters on the premise of ensuring accuracy. Code is available at: https://github.com/sleepeach/SFS-SEI.
更多查看译文
关键词
Specific emitter identification (SEI),deep learning (DL),sparse feature selection (SFS),sparse regularization
AI 理解论文
溯源树
样例
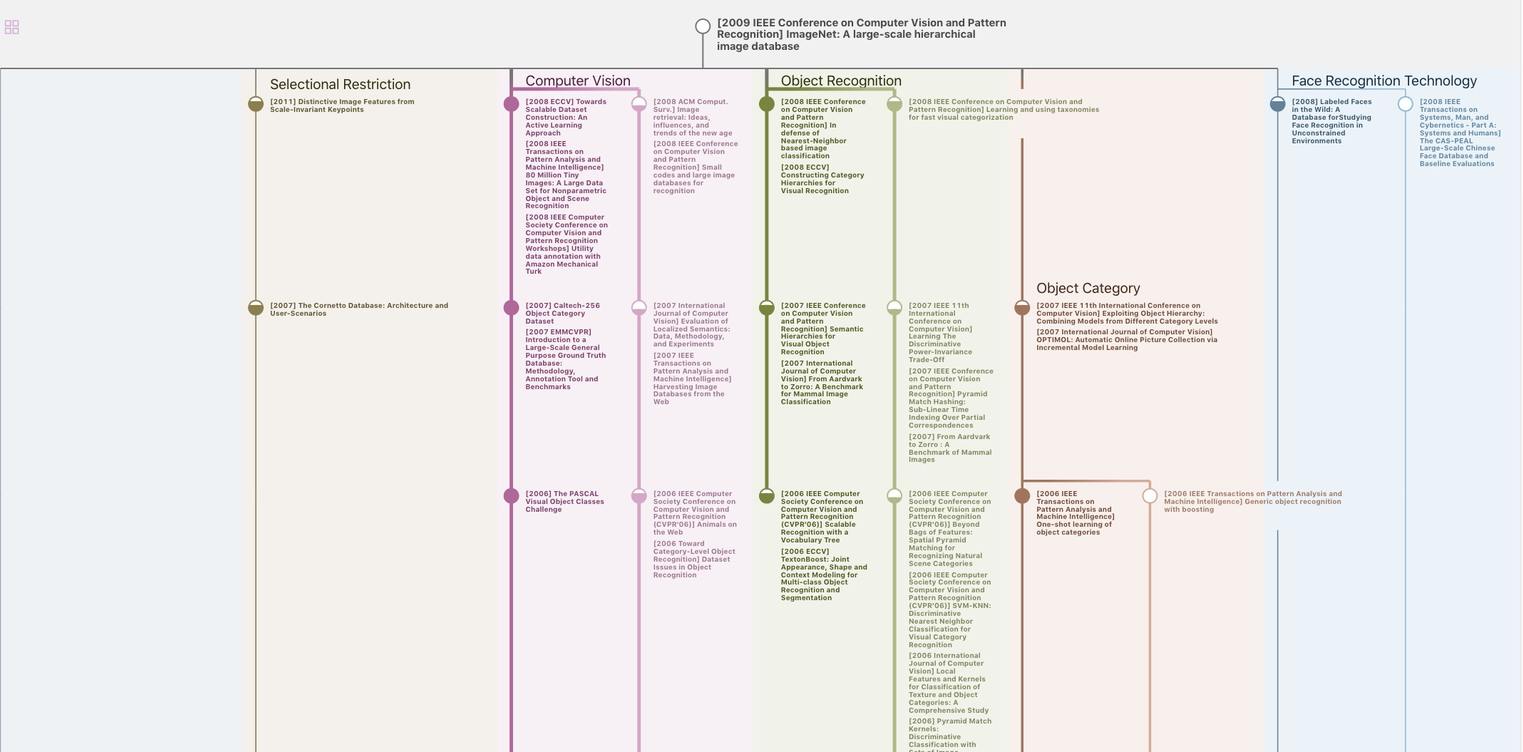
生成溯源树,研究论文发展脉络
Chat Paper
正在生成论文摘要