PAFL: Parameter-Authentication Federated Learning for Internet of Vehicles
IEEE CONFERENCE ON GLOBAL COMMUNICATIONS, GLOBECOM(2023)
摘要
Federated learning is an emerging distributed learning paradigm which brings an efficient and privacy-preserving intelligent model for the Internet of Vehicles (IoV). Unfortunately, federated learning is vulnerable to abnormal model attacks as it is hard to authenticate model parameters. Abnormal local models may slow down the convergence rate, reduce the accuracy of global models, and even deliberately control the global model in the attackers' chosen way. Furthermore, an abnormal global model may deduce sensitive information about vehicles and hinder the execution of genuine tasks. Therefore, in this paper, we propose a parameter-authentication federated learning (PAFL) scheme that can protect privacy of vehicles, such as driving habits, and defend against abnormal model attacks simultaneously. Concretely, we equip the federated learning framework with the zero knowledge proof and Pedersen commitment to prove and authenticate the reliability of model parameters. Security and privacy analysis, as well as performance evaluation show that the PAFL scheme can successfully detect abnormal models with higher detection rate and achieve more secure global aggregation than existing representative schemes.
更多查看译文
关键词
federated learning,IoV,zero knowledge proof,Pedersen commitment,privacy preserving,secure aggregation
AI 理解论文
溯源树
样例
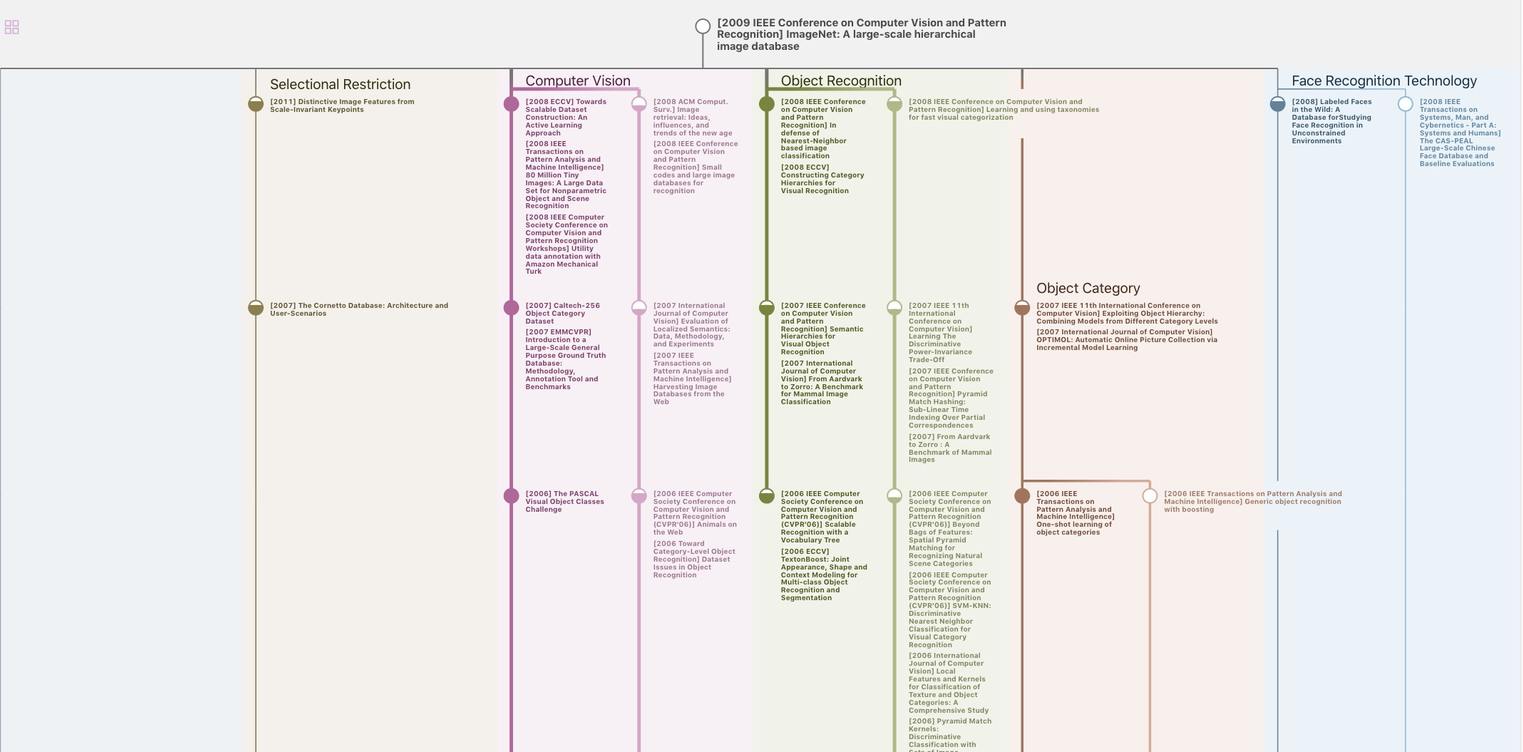
生成溯源树,研究论文发展脉络
Chat Paper
正在生成论文摘要