UC-FL: A User Cooperation Framework for Wireless Federated Learning.
Global Communications Conference(2023)
摘要
This paper considers a wireless federated learning (FL) system, where the parameters of neural networks (NNs) from distributed users are transmitted to the base station (BS) periodically via wireless links for global aggregation. Due to random fading, users experiencing deteriorated channel conditions are unable to upload their NN parameters successfully, which lowers the convergence rate and degrades the accuracy of the NN model. In order to mitigate the influence of channel fading and accelerate convergence, we propose UC-FL, a user cooperation framework for wireless FL. Unlike the traditional FL paradigm where only “vertical” connections (i.e., users-to-BS) are supported, in the UC-FL framework, “horizontal” connections (i.e., users-to-users) are also introduced to enable user cooperation. In this manner, users with good channel conditions help those experiencing deep fading channels to upload their NN parameters, which provides more opportunities for distributed users to participate in global aggregation. Moreover, a novel global aggregation weight design is proposed by taking into account the channel conditions, to further improve the performance. Simulation results demonstrate the superiority of the proposed UC-FL compared with the classic FedAvg counterpart in terms of model accuracy and convergence rate.
更多查看译文
关键词
Federated Learning,User Cooperation,Neural Network,Accuracy Of Model,Artificial Neural Network,Convergence Rate,Channel State,Fading Channel,Neural Network Parameters,Weight Aggregates,Global Weight,Training Data,Objective Function,Convolutional Layers,Test Accuracy,Exponential Distribution,Performance Gain,Global Parameters,Channel Capacity,Local Updates,Uplink Transmission,Transmission Failure,User Selection,Probability Of User
AI 理解论文
溯源树
样例
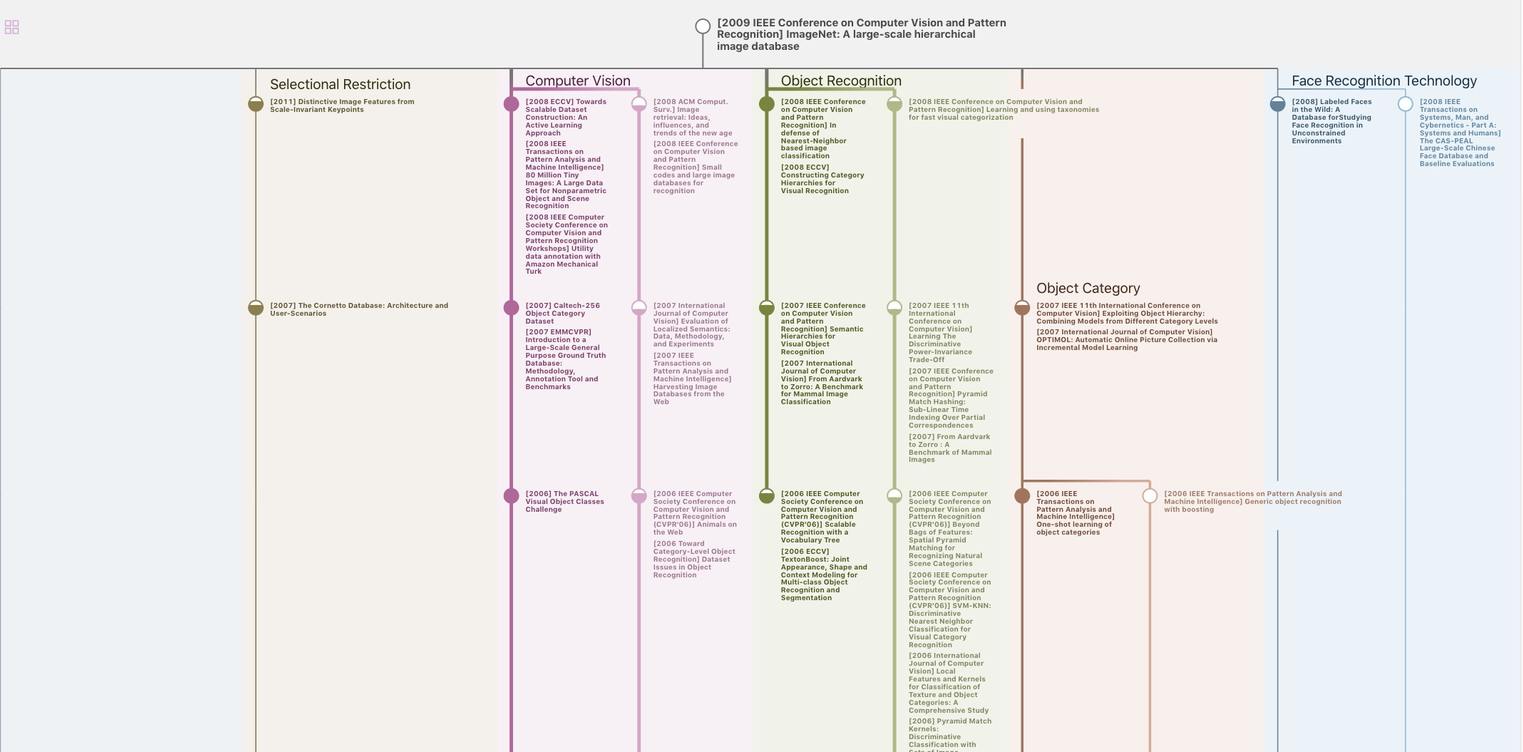
生成溯源树,研究论文发展脉络
Chat Paper
正在生成论文摘要