Probabilistic seasonal forecasts of North Atlantic atmospheric circulation using complex systems modelling and comparison with dynamical models
METEOROLOGICAL APPLICATIONS(2024)
Abstract
Dynamical seasonal forecast models are improving with time but tend to underestimate the amplitude of atmospheric circulation variability and to have lower skill in predicting summer variability than in winter. Here, we construct Nonlinear AutoRegressive Moving Average models with eXogenous inputs (NARMAX) to develop the analysis of drivers of North Atlantic atmospheric circulation and jet-stream variability, focusing on the East Atlantic (EA) and Scandinavian (SCA) patterns as well as the North Atlantic Oscillation (NAO) index. New time series of these indices are developed from empirical orthogonal function (EOF) analysis. Geopotential height data from the ERA5 reanalysis are used to generate the EOFs. Sets of predictors with known associations with these drivers are developed and used to formulate a sliding-window NARMAX model. This model demonstrates a high degree of predictive accuracy, as indicated by its average correlation coefficients over the testing period (2006-2021): 0.78 for NAO, 0.83 for EA and 0.68 for SCA. In comparison, the SEAS5 and GloSea5 dynamical forecast models exhibit lower correlations with observed circulation changes: for NAO, the correlation coefficients are 0.51 for SEAS5 and 0.34 for GloSea5, for EA they are 0.15 and 0.09, respectively, and for SCA, they are 0.28 and 0.24, respectively. Comparison of NARMAX predictions with forecasts and hindcasts from the SEAS5 and GloSea5 models highlights areas where NARMAX can be used to help improve seasonal forecast skill and inform the development of dynamical models, especially in the case of summer. Dynamical seasonal forecast models are improving with time but tend to underestimate the amplitude of atmospheric circulation variability and have lower skill in predicting summer variability than in winter. This study constructs Nonlinear AutoRegressive Moving Average models with eXogenous inputs (NARMAX) to develop the analysis of drivers of North Atlantic atmospheric circulation and jet-stream variability. This model exhibits high skill in its predictions and robustness to help improve seasonal forecast skills and inform the development of dynamical models. image
MoreTranslated text
Key words
ensemble forecasts,forecasting,machine learning,miscellaneous,NARMAX,NorthAtlantic atmospheric circulation,probabilistic forecasts,probabilistic seasonal forecast,seasonal,verification,weather prediction
AI Read Science
Must-Reading Tree
Example
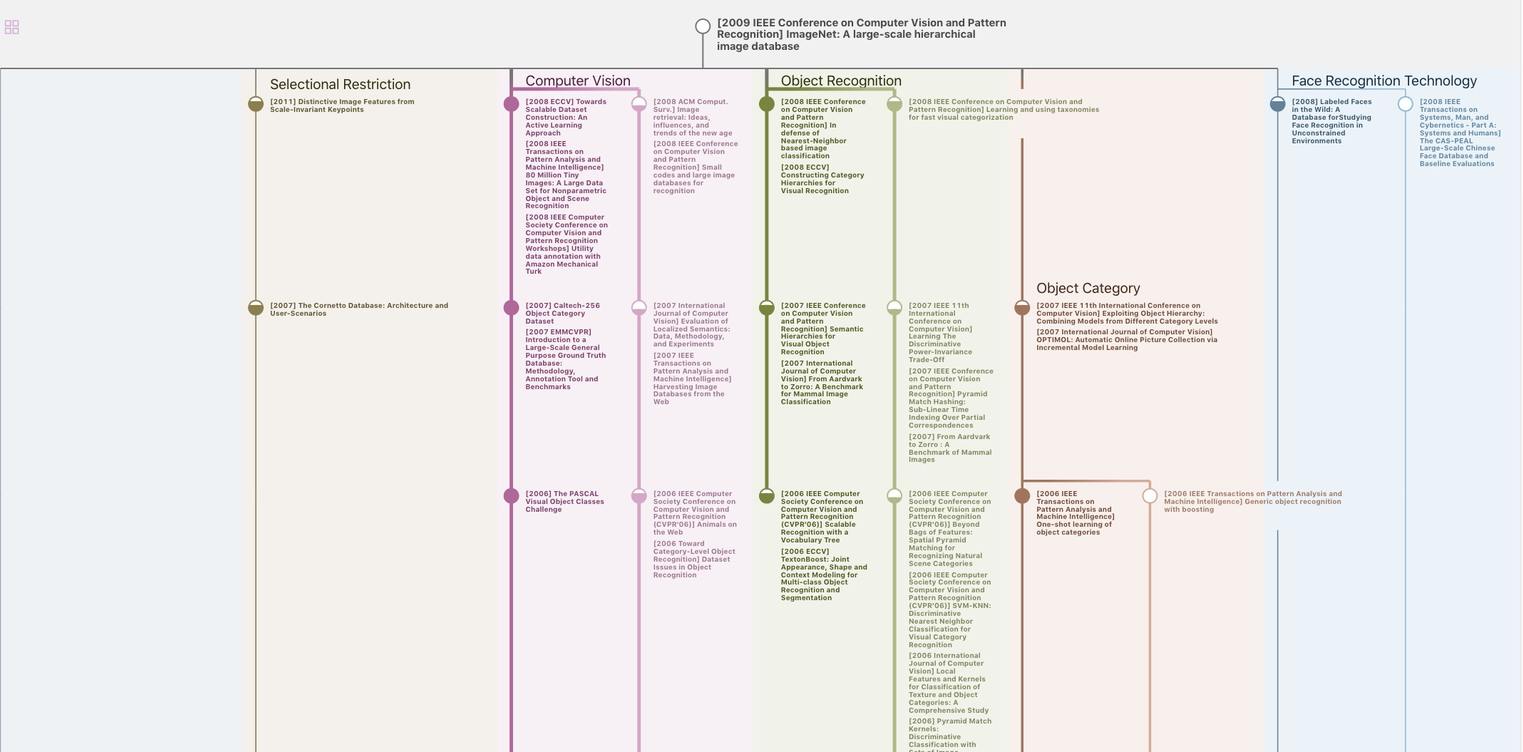
Generate MRT to find the research sequence of this paper
Chat Paper
Summary is being generated by the instructions you defined