Model-agnostic generation-enhanced technology for few-shot intrusion detection
Applied Intelligence(2024)
摘要
Malicious traffic on the Internet has become an increasingly serious problem, and several artificial intelligence (AI)-based malicious traffic detection methods have been proposed. Generally, AI-based methods need numerous benign and specific types of malicious traffic training instances to achieve better detection results. However, for attacks with only a few instances, known as the few-shot attacks, these methods often perform poorly, and how to train a model for detecting few-shot attacks is a huge challenge. For this problem, we propose a novel intrusion detection system based on generative adversarial networks and model-agnostic meta-learning. The system adopts a hybrid detection mechanism where an anomaly-based classifier determines whether incoming traffic is malicious and a signature-based classifier identifies the class of malicious traffic. In the system, the samples of few-shot attacks are augmented by maximizing the use of meta-knowledge and then applied to assist the detection of few-shot attacks to obtain better detection results. The experiments show that for CSE-CIC-IDS2018 and Bot-IoT datasets, this system can detect malicious traffic with 94.3
更多查看译文
关键词
Intrusion detection,Model-agnostic meta-learning,Generative adversarial network,Few-shot attacks,Imbalanced dataset
AI 理解论文
溯源树
样例
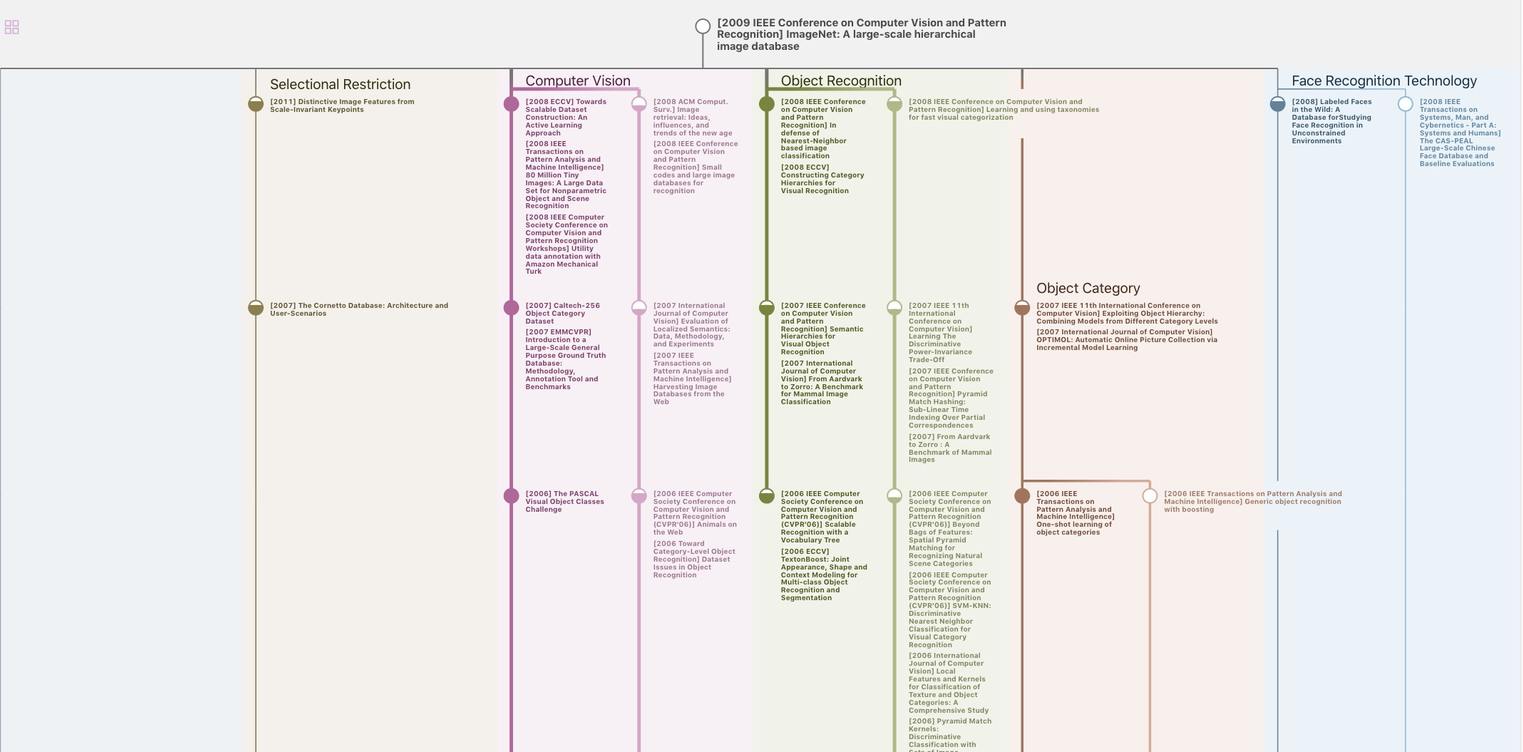
生成溯源树,研究论文发展脉络
Chat Paper
正在生成论文摘要