Physics-Informed LSTM-Based Delay Compensation Framework for Teleoperated UGVs
CoRR(2024)
摘要
Bilateral teleoperation of low-speed Unmanned Ground Vehicles (UGVs) on soft
terrains is crucial for applications like lunar exploration, offering effective
control of terrain-induced longitudinal slippage. However, latency arising from
transmission delays over a network presents a challenge in maintaining
high-fidelity closed-loop integration, potentially hindering UGV controls and
leading to poor command-tracking performance. To address this challenge, this
paper proposes a novel predictor framework that employs a Physics-informed Long
Short-Term Memory (PiLSTM) network for designing bilateral teleoperator
controls that effectively compensate for large delays. Contrasting with
conventional model-free predictor frameworks, which are limited by their linear
nature in capturing nonlinear and temporal dynamic behaviors, our approach
integrates the LSTM structure with physical constraints for enhanced
performance and better generalization across varied scenarios. Specifically,
four distinct predictors were employed in the framework: two compensate for
forward delays, while the other two compensate for backward delays. Due to
their effectiveness in learning from temporal data, the proposed PiLSTM
framework demonstrates a 26.1 improvement in delay compensation over the
conventional model-free predictors for large delays in open-loop case studies.
Subsequently, experiments were conducted to validate the efficacy of the
framework in close-loop scenarios, particularly to compensate for the
real-network delays experienced by teleoperated UGVs coupled with longitudinal
slippage. The results confirm the proposed framework is effective in restoring
the fidelity of the closed-loop integration. This improvement is showcased
through improved performance and transparency, which leads to excellent
command-tracking performance.
更多查看译文
AI 理解论文
溯源树
样例
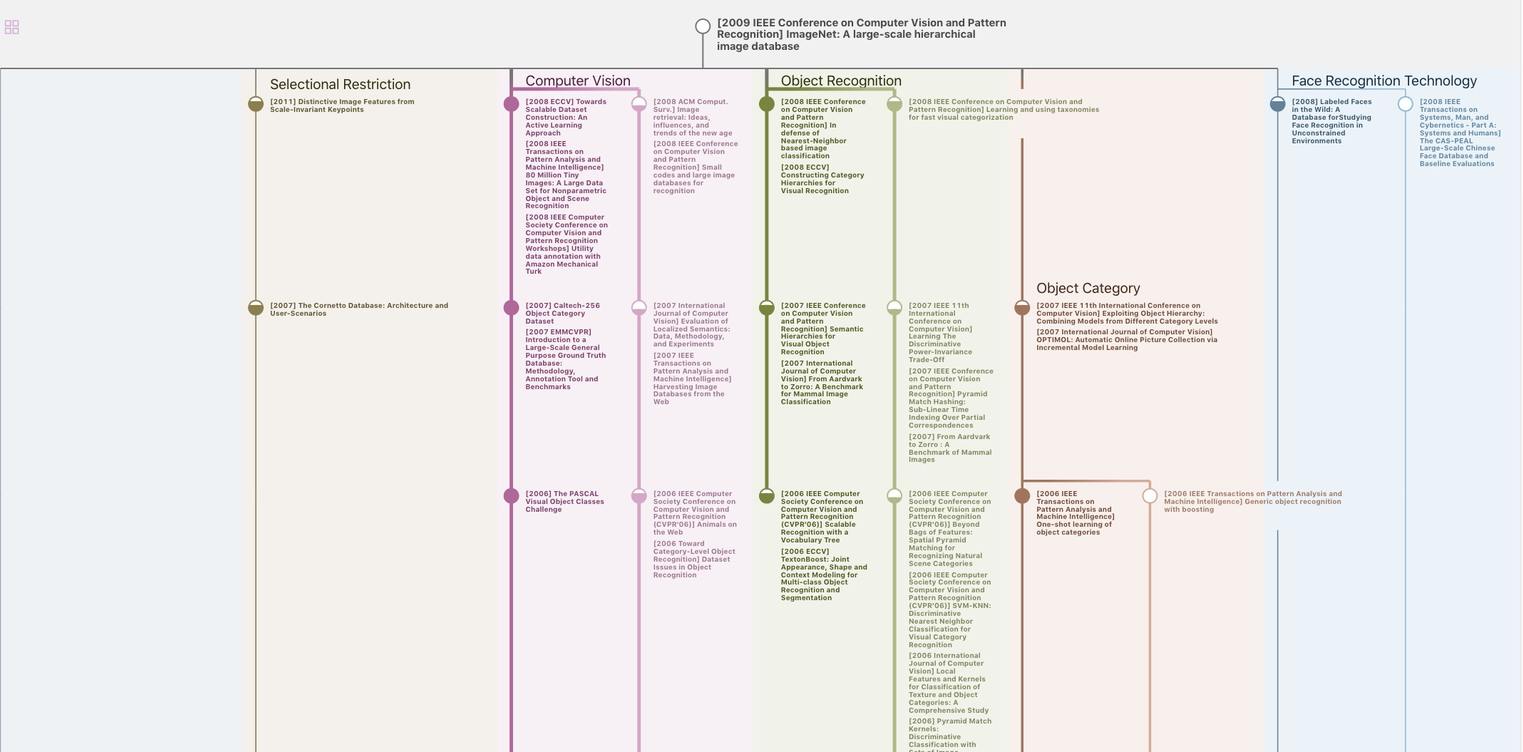
生成溯源树,研究论文发展脉络
Chat Paper
正在生成论文摘要