Poster: Device-Cloud Collaborative Video Storage for Real-time Ultra HD Video Playback.
HOTMOBILE '24 Proceedings of the 25th International Workshop on Mobile Computing Systems and Applications(2024)
Abstract
With the rapid advancement of mobile hardware, smart-phones are now capable of capturing and playing videos in 4K and even 8K resolution, improving immersive and enjoyable viewing experience for users. However, storing these ultra-high-resolution videos poses a significant burden on the local storage of mobile devices. An alternative method is storing video on cloud with the benefits of scalable storage space, cross-device data access, data sharing and backup. Recently, the policy has been integrated into mainstream mobile operation systems. Nevertheless, downloading video from cloud may face high latency under poor network conditions, significantly diminishing the user experience. Recent advances in client-side computation create a new opportunity for utilizing network-based super-resolution techniques to improve the quality of the displayed low-resolution videos during playback[1]. However, super-resolution is an ill-posed problem, indicating that even the same low-resolution video may correspond to multiple high-resolution videos. This implies that relying solely on low-resolution information to recover high-definition details is a challenging task. Studies have revealed that the utilization of reference high-definition information can significantly enhance the effectiveness of super-resolution algorithms[2]. We observe that a device-cloud collaborative paradigm offers new opportunities to address the challenges of storing and playing high-resolution videos. By integrating cloud storage with on-device video enhancement and using high-resolution patches from the cloud as reference information, the quality of displayed local video can be significantly improved, thereby enhancing the playback experience. We propose the DuoSR system, which encompasses two phases: storage phase and playback phase. In the storage phase (Figure 1a), We have designed a down-sampling neural network and joint training it with the super-resolution network to optimize video compression. Concurrently, we design a neural-enhanced quality prediction module to predict the video quality under different reference patch and different key frame. The module generates a quality prediction table, facilitating cloud quality planner during the playback phase. In the playback phase (Figure 1b), initially, when the user accesses a video, real-time device and network information are uploaded to the cloud. Based on these data, the cloud, aiming to maximize the Quality of Experience (QoE), determines the key frames for super-resolution and the reference information to be transmitted. Upon receiving the information about the key frames and the reference information on the device, RefVSR is performed on the key frames to reconstruct high resolution key frames. For non-key frames, we reuse the results from key frames, employing the video codec's motion vectors and residual information to upsample them.
MoreTranslated text
AI Read Science
Must-Reading Tree
Example
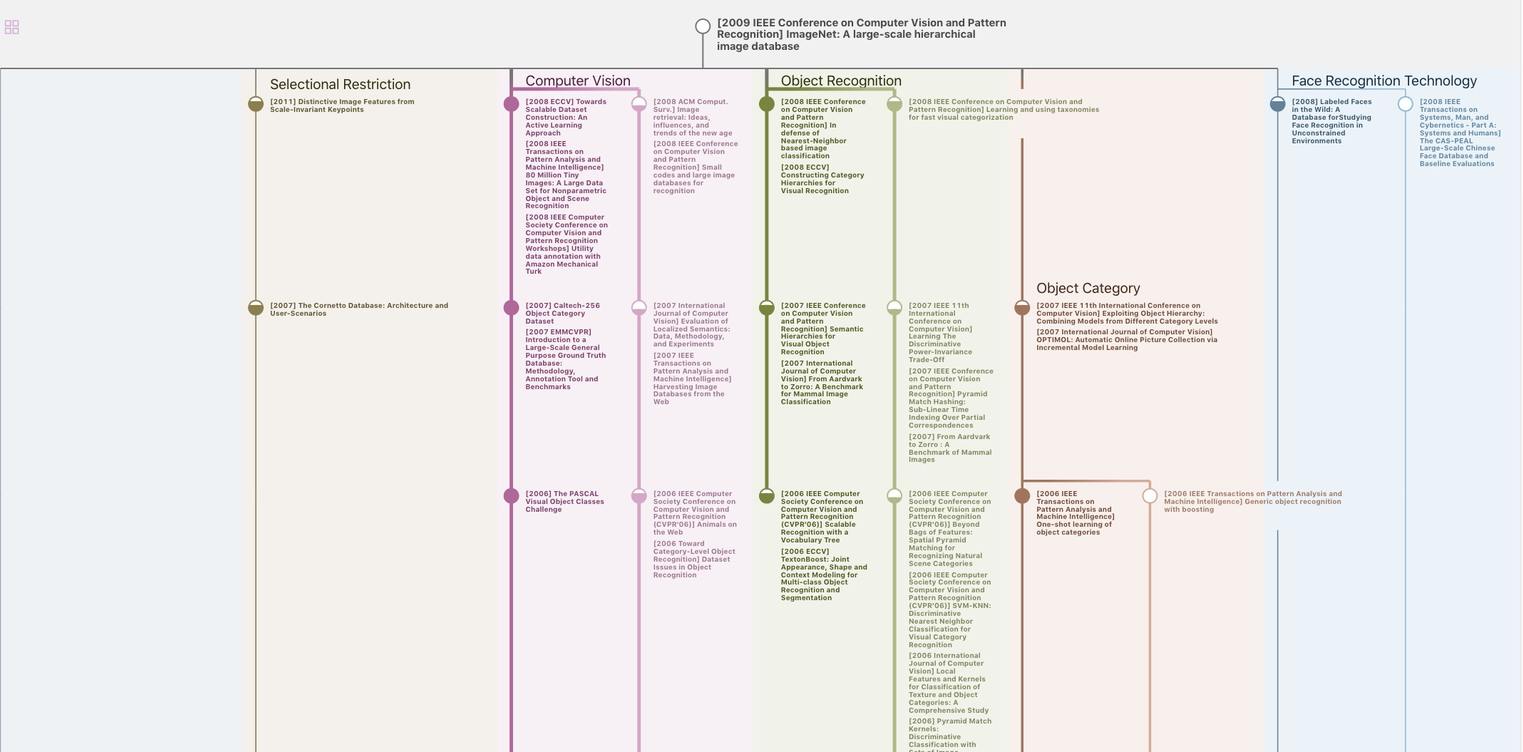
Generate MRT to find the research sequence of this paper
Chat Paper
Summary is being generated by the instructions you defined