Poster: Olfactory Sensing in Turbulent Airflow via Collaborative Robots.
Workshop on Mobile Computing Systems and Applications(2024)
摘要
Unforeseen gas leaks can swiftly create a highly flammable atmosphere, which will lead to risks of explosions, health hazards, as well as long-term environmental impacts, especially when the gas is toxic. Hence, localizing the gas source as soon as possible remains paramount to executing subsequent mitigation measures, such as shutting off valves and effectively containing or sealing the leaks. Assigning human operators to engage in these laborious activities poses a risk to their health and lives. Deploying autonomous mobile olfactory robots for gas source localization (GSL) presents a solution with great potential, as they can search the environment efficiently without risking human lives. We task gas leakage in a factory with intricate pipelines as an example as shown in fig. 1(a). Here, a fleet of robots equipped with gas concentration sensors [4] and wind sensors are dispatched to search the emission source. Unfortunately, existing approaches are not able to offer feasible GSL solutions for mobile olfaction-based robots in realistic environments [1]. Specifically, the patchy nature of gas plumes and the discontinuity of the concentration field confuses and traps the robots. As demonstrated by experimental data in fig. 1(b), plumes fragment into disjointed patches spatially. This intermittent characteristic introduces uncertainty in the source direction when robots devise paths using noisy and local sensory data, ultimately leading to their entrapment within these patches. Our work aims to deploy and schedule a team of robots to conduct efficient GSL in realistic patchy gas environments. We develop GasHunter, a collaborative strategy for multiple robots by exchange-based team roles adaptation to balance the search efficiency and localization effectiveness of gas sources. Robots are assigned heterogeneous roles that represent different planning preferences. For example, some robots are responsible for inspecting spurious concentration maxima (exploiter), while others are in charge of discovering new potential source positions (explorer). The robots' roles are adjusted (exchanged) adaptively based on their current positions and the potential to localize the source in the surroundings, thus parallelizing the exploration of the environment and exploitation of collected information in a flexible manner. We validate our design with experiments on a multi-robot testbed and large scale physical feature based simulations. We compared GasHunter with two related state-of-the-art (SOTA) methods, Surge-Cast [2] and Infotaxis [3]. We visualize the trajectories of all the methods in fig. 2 (a)-(c). It is demonstrated that GasHunter produces smoother trajectories than baselines. Surge-Cast yields inefficient zig-zag paths, Infotaxis suffers from trap in patchy areas. fig. 2 (d) illustrates the nearest distance to the source within all robots over time. It's verified that GasHunter navigates towards the source the most efficiently and arrives at the source the most quickly, indicating a decrease in search time by 37.23% and 22.46% in comparison to the two baselines, respectively.
更多查看译文
AI 理解论文
溯源树
样例
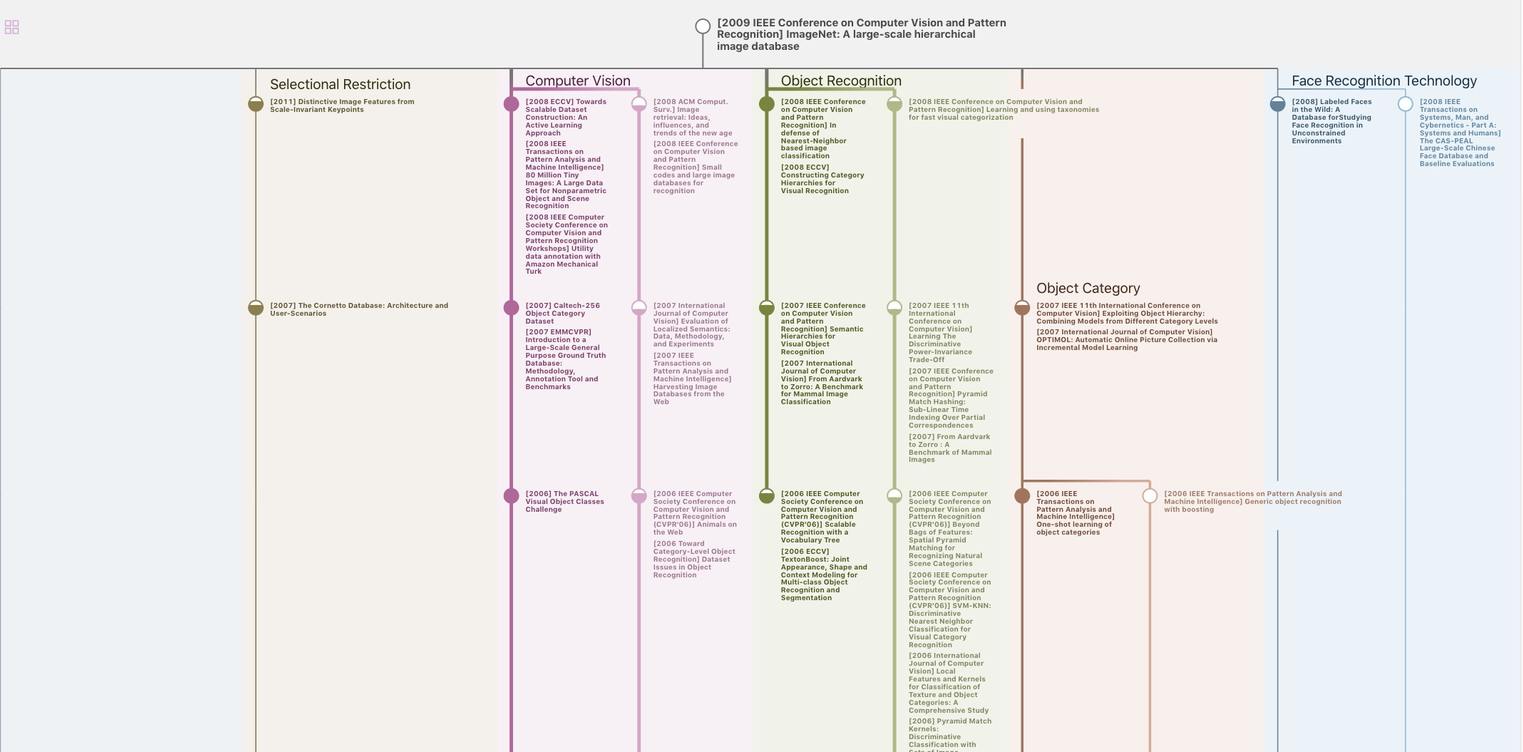
生成溯源树,研究论文发展脉络
Chat Paper
正在生成论文摘要