Large Stepsize Gradient Descent for Logistic Loss: Non-Monotonicity of the Loss Improves Optimization Efficiency
CoRR(2024)
摘要
We consider gradient descent (GD) with a constant stepsize applied to
logistic regression with linearly separable data, where the constant stepsize
η is so large that the loss initially oscillates. We show that GD exits
this initial oscillatory phase rapidly – in 𝒪(η) steps – and
subsequently achieves an 𝒪̃(1 / (η t) ) convergence rate
after t additional steps. Our results imply that, given a budget of T
steps, GD can achieve an accelerated loss of 𝒪̃(1/T^2) with
an aggressive stepsize η:= Θ( T), without any use of momentum or
variable stepsize schedulers. Our proof technique is versatile and also handles
general classification loss functions (where exponential tails are needed for
the 𝒪̃(1/T^2) acceleration), nonlinear predictors in the
neural tangent kernel regime, and online stochastic gradient descent (SGD) with
a large stepsize, under suitable separability conditions.
更多查看译文
AI 理解论文
溯源树
样例
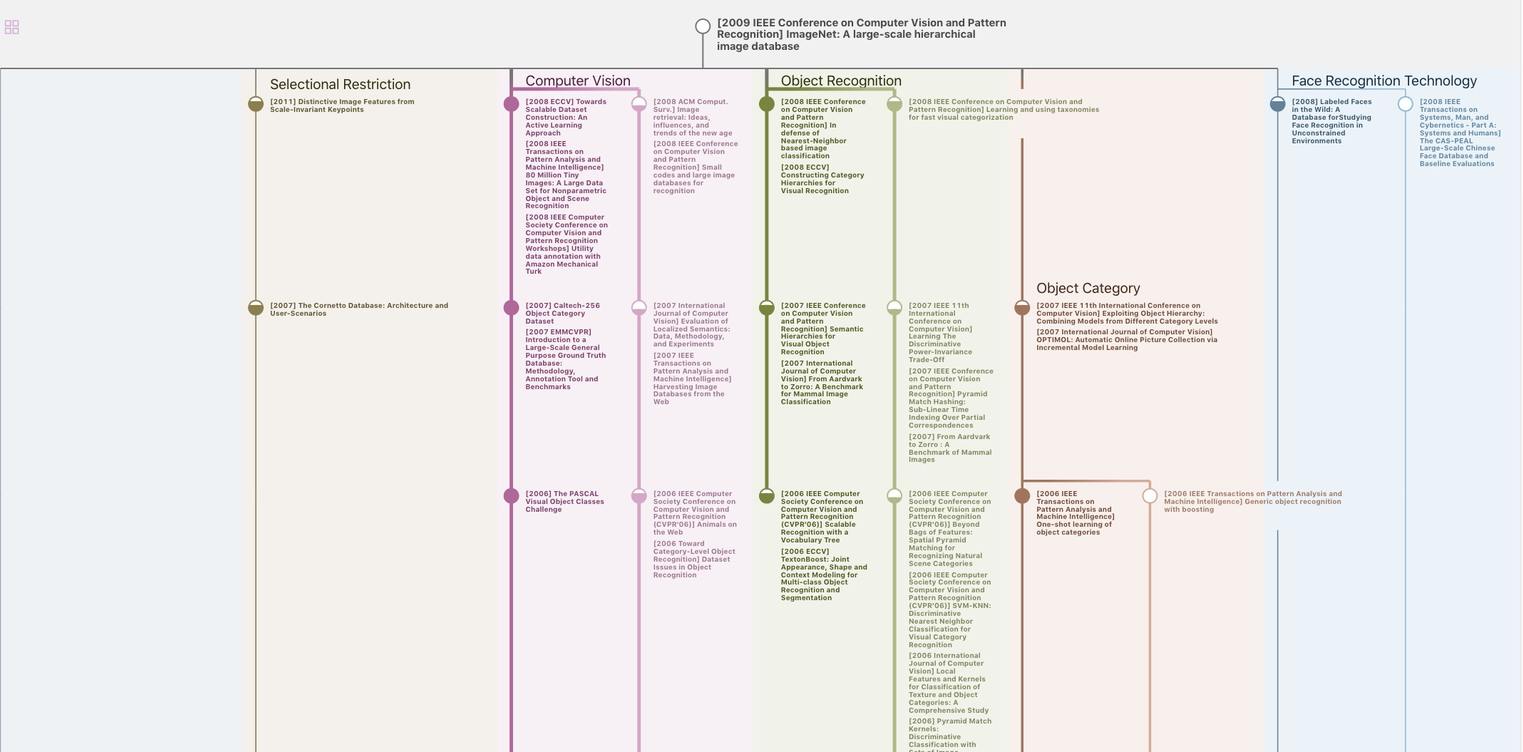
生成溯源树,研究论文发展脉络
Chat Paper
正在生成论文摘要